mirror of
https://github.com/huggingface/text-generation-inference.git
synced 2025-04-24 16:32:12 +00:00
Add changes from Optimum Habana's TGI folder
This commit is contained in:
parent
7a6fad6aac
commit
cc744ba426
159
Dockerfile
159
Dockerfile
@ -2,8 +2,6 @@
|
||||
FROM lukemathwalker/cargo-chef:latest-rust-1.71 AS chef
|
||||
WORKDIR /usr/src
|
||||
|
||||
ARG CARGO_REGISTRIES_CRATES_IO_PROTOCOL=sparse
|
||||
|
||||
FROM chef as planner
|
||||
COPY Cargo.toml Cargo.toml
|
||||
COPY rust-toolchain.toml rust-toolchain.toml
|
||||
@ -15,9 +13,6 @@ RUN cargo chef prepare --recipe-path recipe.json
|
||||
|
||||
FROM chef AS builder
|
||||
|
||||
ARG GIT_SHA
|
||||
ARG DOCKER_LABEL
|
||||
|
||||
RUN PROTOC_ZIP=protoc-21.12-linux-x86_64.zip && \
|
||||
curl -OL https://github.com/protocolbuffers/protobuf/releases/download/v21.12/$PROTOC_ZIP && \
|
||||
unzip -o $PROTOC_ZIP -d /usr/local bin/protoc && \
|
||||
@ -35,123 +30,18 @@ COPY router router
|
||||
COPY launcher launcher
|
||||
RUN cargo build --release
|
||||
|
||||
# Python builder
|
||||
# Adapted from: https://github.com/pytorch/pytorch/blob/master/Dockerfile
|
||||
FROM debian:bullseye-slim as pytorch-install
|
||||
|
||||
ARG PYTORCH_VERSION=2.0.1
|
||||
ARG PYTHON_VERSION=3.9
|
||||
# Keep in sync with `server/pyproject.toml
|
||||
ARG CUDA_VERSION=11.8
|
||||
ARG MAMBA_VERSION=23.1.0-1
|
||||
ARG CUDA_CHANNEL=nvidia
|
||||
ARG INSTALL_CHANNEL=pytorch
|
||||
# Automatically set by buildx
|
||||
ARG TARGETPLATFORM
|
||||
|
||||
ENV PATH /opt/conda/bin:$PATH
|
||||
|
||||
RUN apt-get update && DEBIAN_FRONTEND=noninteractive apt-get install -y --no-install-recommends \
|
||||
build-essential \
|
||||
ca-certificates \
|
||||
ccache \
|
||||
curl \
|
||||
git && \
|
||||
rm -rf /var/lib/apt/lists/*
|
||||
|
||||
# Install conda
|
||||
# translating Docker's TARGETPLATFORM into mamba arches
|
||||
RUN case ${TARGETPLATFORM} in \
|
||||
"linux/arm64") MAMBA_ARCH=aarch64 ;; \
|
||||
*) MAMBA_ARCH=x86_64 ;; \
|
||||
esac && \
|
||||
curl -fsSL -v -o ~/mambaforge.sh -O "https://github.com/conda-forge/miniforge/releases/download/${MAMBA_VERSION}/Mambaforge-${MAMBA_VERSION}-Linux-${MAMBA_ARCH}.sh"
|
||||
RUN chmod +x ~/mambaforge.sh && \
|
||||
bash ~/mambaforge.sh -b -p /opt/conda && \
|
||||
rm ~/mambaforge.sh
|
||||
|
||||
# Install pytorch
|
||||
# On arm64 we exit with an error code
|
||||
RUN case ${TARGETPLATFORM} in \
|
||||
"linux/arm64") exit 1 ;; \
|
||||
*) /opt/conda/bin/conda update -y conda && \
|
||||
/opt/conda/bin/conda install -c "${INSTALL_CHANNEL}" -c "${CUDA_CHANNEL}" -y "python=${PYTHON_VERSION}" pytorch==$PYTORCH_VERSION "pytorch-cuda=$(echo $CUDA_VERSION | cut -d'.' -f 1-2)" ;; \
|
||||
esac && \
|
||||
/opt/conda/bin/conda clean -ya
|
||||
|
||||
# CUDA kernels builder image
|
||||
FROM pytorch-install as kernel-builder
|
||||
|
||||
RUN apt-get update && DEBIAN_FRONTEND=noninteractive apt-get install -y --no-install-recommends \
|
||||
ninja-build \
|
||||
&& rm -rf /var/lib/apt/lists/*
|
||||
|
||||
RUN /opt/conda/bin/conda install -c "nvidia/label/cuda-11.8.0" cuda==11.8 && \
|
||||
/opt/conda/bin/conda clean -ya
|
||||
|
||||
# Build Flash Attention CUDA kernels
|
||||
FROM kernel-builder as flash-att-builder
|
||||
|
||||
WORKDIR /usr/src
|
||||
|
||||
COPY server/Makefile-flash-att Makefile
|
||||
|
||||
# Build specific version of flash attention
|
||||
RUN make build-flash-attention
|
||||
|
||||
# Build Flash Attention v2 CUDA kernels
|
||||
FROM kernel-builder as flash-att-v2-builder
|
||||
|
||||
WORKDIR /usr/src
|
||||
|
||||
COPY server/Makefile-flash-att-v2 Makefile
|
||||
|
||||
# Build specific version of flash attention v2
|
||||
RUN make build-flash-attention-v2
|
||||
|
||||
# Build Transformers exllama kernels
|
||||
FROM kernel-builder as exllama-kernels-builder
|
||||
WORKDIR /usr/src
|
||||
COPY server/exllama_kernels/ .
|
||||
# Build specific version of transformers
|
||||
RUN TORCH_CUDA_ARCH_LIST="8.0;8.6+PTX" python setup.py build
|
||||
|
||||
# Build Transformers awq kernels
|
||||
FROM kernel-builder as awq-kernels-builder
|
||||
WORKDIR /usr/src
|
||||
COPY server/Makefile-awq Makefile
|
||||
# Build specific version of transformers
|
||||
RUN TORCH_CUDA_ARCH_LIST="8.0;8.6+PTX" make build-awq
|
||||
|
||||
# Build Transformers CUDA kernels
|
||||
FROM kernel-builder as custom-kernels-builder
|
||||
WORKDIR /usr/src
|
||||
COPY server/custom_kernels/ .
|
||||
# Build specific version of transformers
|
||||
RUN python setup.py build
|
||||
|
||||
# Build vllm CUDA kernels
|
||||
FROM kernel-builder as vllm-builder
|
||||
|
||||
WORKDIR /usr/src
|
||||
|
||||
COPY server/Makefile-vllm Makefile
|
||||
|
||||
# Build specific version of vllm
|
||||
RUN make build-vllm
|
||||
|
||||
# Text Generation Inference base image
|
||||
FROM nvidia/cuda:11.8.0-base-ubuntu20.04 as base
|
||||
|
||||
# Conda env
|
||||
ENV PATH=/opt/conda/bin:$PATH \
|
||||
CONDA_PREFIX=/opt/conda
|
||||
FROM vault.habana.ai/gaudi-docker/1.13.0/ubuntu22.04/habanalabs/pytorch-installer-2.1.0:latest as base
|
||||
|
||||
# Text Generation Inference base env
|
||||
ENV HUGGINGFACE_HUB_CACHE=/data \
|
||||
HF_HUB_ENABLE_HF_TRANSFER=1 \
|
||||
PORT=80
|
||||
|
||||
# libssl.so.1.1 is not installed on Ubuntu 22.04 by default, install it
|
||||
RUN wget http://nz2.archive.ubuntu.com/ubuntu/pool/main/o/openssl/libssl1.1_1.1.1f-1ubuntu2_amd64.deb && \
|
||||
dpkg -i ./libssl1.1_1.1.1f-1ubuntu2_amd64.deb
|
||||
|
||||
WORKDIR /usr/src
|
||||
|
||||
RUN apt-get update && DEBIAN_FRONTEND=noninteractive apt-get install -y --no-install-recommends \
|
||||
@ -161,30 +51,6 @@ RUN apt-get update && DEBIAN_FRONTEND=noninteractive apt-get install -y --no-ins
|
||||
curl \
|
||||
&& rm -rf /var/lib/apt/lists/*
|
||||
|
||||
# Copy conda with PyTorch installed
|
||||
COPY --from=pytorch-install /opt/conda /opt/conda
|
||||
|
||||
# Copy build artifacts from flash attention builder
|
||||
COPY --from=flash-att-builder /usr/src/flash-attention/build/lib.linux-x86_64-cpython-39 /opt/conda/lib/python3.9/site-packages
|
||||
COPY --from=flash-att-builder /usr/src/flash-attention/csrc/layer_norm/build/lib.linux-x86_64-cpython-39 /opt/conda/lib/python3.9/site-packages
|
||||
COPY --from=flash-att-builder /usr/src/flash-attention/csrc/rotary/build/lib.linux-x86_64-cpython-39 /opt/conda/lib/python3.9/site-packages
|
||||
|
||||
# Copy build artifacts from flash attention v2 builder
|
||||
COPY --from=flash-att-v2-builder /usr/src/flash-attention-v2/build/lib.linux-x86_64-cpython-39 /opt/conda/lib/python3.9/site-packages
|
||||
|
||||
# Copy build artifacts from custom kernels builder
|
||||
COPY --from=custom-kernels-builder /usr/src/build/lib.linux-x86_64-cpython-39 /opt/conda/lib/python3.9/site-packages
|
||||
# Copy build artifacts from exllama kernels builder
|
||||
COPY --from=exllama-kernels-builder /usr/src/build/lib.linux-x86_64-cpython-39 /opt/conda/lib/python3.9/site-packages
|
||||
# Copy build artifacts from awq kernels builder
|
||||
COPY --from=awq-kernels-builder /usr/src/llm-awq/awq/kernels/build/lib.linux-x86_64-cpython-39 /opt/conda/lib/python3.9/site-packages
|
||||
|
||||
# Copy builds artifacts from vllm builder
|
||||
COPY --from=vllm-builder /usr/src/vllm/build/lib.linux-x86_64-cpython-39 /opt/conda/lib/python3.9/site-packages
|
||||
|
||||
# Install flash-attention dependencies
|
||||
RUN pip install einops --no-cache-dir
|
||||
|
||||
# Install server
|
||||
COPY proto proto
|
||||
COPY server server
|
||||
@ -192,7 +58,7 @@ COPY server/Makefile server/Makefile
|
||||
RUN cd server && \
|
||||
make gen-server && \
|
||||
pip install -r requirements.txt && \
|
||||
pip install ".[bnb, accelerate, quantize]" --no-cache-dir
|
||||
pip install . --no-cache-dir
|
||||
|
||||
# Install benchmarker
|
||||
COPY --from=builder /usr/src/target/release/text-generation-benchmark /usr/local/bin/text-generation-benchmark
|
||||
@ -201,19 +67,6 @@ COPY --from=builder /usr/src/target/release/text-generation-router /usr/local/bi
|
||||
# Install launcher
|
||||
COPY --from=builder /usr/src/target/release/text-generation-launcher /usr/local/bin/text-generation-launcher
|
||||
|
||||
RUN apt-get update && DEBIAN_FRONTEND=noninteractive apt-get install -y --no-install-recommends \
|
||||
build-essential \
|
||||
g++ \
|
||||
&& rm -rf /var/lib/apt/lists/*
|
||||
|
||||
# AWS Sagemaker compatbile image
|
||||
FROM base as sagemaker
|
||||
|
||||
COPY sagemaker-entrypoint.sh entrypoint.sh
|
||||
RUN chmod +x entrypoint.sh
|
||||
|
||||
ENTRYPOINT ["./entrypoint.sh"]
|
||||
|
||||
# Final image
|
||||
FROM base
|
||||
|
||||
|
9
Makefile
9
Makefile
@ -1,9 +1,6 @@
|
||||
install-server:
|
||||
cd server && make install
|
||||
|
||||
install-custom-kernels:
|
||||
if [ "$$BUILD_EXTENSIONS" = "True" ]; then cd server/custom_kernels && python setup.py install; else echo "Custom kernels are disabled, you need to set the BUILD_EXTENSIONS environment variable to 'True' in order to build them. (Please read the docs, kernels might not work on all hardware)"; fi
|
||||
|
||||
install-integration-tests:
|
||||
cd integration-tests && pip install -r requirements.txt
|
||||
cd clients/python && pip install .
|
||||
@ -45,8 +42,8 @@ python-tests: python-server-tests python-client-tests
|
||||
run-falcon-7b-instruct:
|
||||
text-generation-launcher --model-id tiiuae/falcon-7b-instruct --port 8080
|
||||
|
||||
run-falcon-7b-instruct-quantize:
|
||||
text-generation-launcher --model-id tiiuae/falcon-7b-instruct --quantize bitsandbytes --port 8080
|
||||
|
||||
clean:
|
||||
rm -rf target aml
|
||||
|
||||
debug_image_build:
|
||||
docker build --no-cache --progress=plain -t debug_tgi .
|
||||
|
359
README.md
359
README.md
@ -1,288 +1,83 @@
|
||||
<!---
|
||||
Copyright 2023 The HuggingFace Team. All rights reserved.
|
||||
|
||||
Licensed under the Apache License, Version 2.0 (the "License");
|
||||
you may not use this file except in compliance with the License.
|
||||
You may obtain a copy of the License at
|
||||
|
||||
http://www.apache.org/licenses/LICENSE-2.0
|
||||
|
||||
Unless required by applicable law or agreed to in writing, software
|
||||
distributed under the License is distributed on an "AS IS" BASIS,
|
||||
WITHOUT WARRANTIES OR CONDITIONS OF ANY KIND, either express or implied.
|
||||
See the License for the specific language governing permissions and
|
||||
limitations under the License.
|
||||
-->
|
||||
|
||||
# Text Generation Inference on Habana Gaudi
|
||||
|
||||
To use [🤗 text-generation-inference](https://github.com/huggingface/text-generation-inference) on Habana Gaudi/Gaudi2, follow these steps:
|
||||
|
||||
1. Build the Docker image located in this folder with:
|
||||
```bash
|
||||
docker build -t tgi_gaudi .
|
||||
```
|
||||
2. Launch a local server instance on 1 Gaudi card:
|
||||
```bash
|
||||
model=meta-llama/Llama-2-7b-hf
|
||||
volume=$PWD/data # share a volume with the Docker container to avoid downloading weights every run
|
||||
|
||||
docker run -p 8080:80 -v $volume:/data --runtime=habana -e HABANA_VISIBLE_DEVICES=all -e OMPI_MCA_btl_vader_single_copy_mechanism=none --cap-add=sys_nice --ipc=host tgi_gaudi --model-id $model
|
||||
```
|
||||
3. Launch a local server instance on 8 Gaudi cards:
|
||||
```bash
|
||||
model=meta-llama/Llama-2-70b-hf
|
||||
volume=$PWD/data # share a volume with the Docker container to avoid downloading weights every run
|
||||
|
||||
docker run -p 8080:80 -v $volume:/data --runtime=habana -e PT_HPU_ENABLE_LAZY_COLLECTIVES=true -e HABANA_VISIBLE_DEVICES=all -e OMPI_MCA_btl_vader_single_copy_mechanism=none --cap-add=sys_nice --ipc=host tgi_gaudi --model-id $model --sharded true --num-shard 8
|
||||
```
|
||||
4. You can then send a request:
|
||||
```bash
|
||||
curl 127.0.0.1:8080/generate \
|
||||
-X POST \
|
||||
-d '{"inputs":"What is Deep Learning?","parameters":{"max_new_tokens":17, "do_sample": true}}' \
|
||||
-H 'Content-Type: application/json'
|
||||
```
|
||||
> The first call will be slower as the model is compiled.
|
||||
5. To run benchmark test, please refer [TGI's benchmark tool](https://github.com/huggingface/text-generation-inference/tree/main/benchmark).
|
||||
|
||||
To run it on the same machine, you can do the following:
|
||||
* `docker exec -it <docker name> bash` , pick the docker started from step 3 or 4 using docker ps
|
||||
* `text-generation-benchmark -t <model-id>` , pass the model-id from docker run command
|
||||
* after the completion of tests, hit ctrl+c to see the performance data summary.
|
||||
|
||||
> For gated models such as [StarCoder](https://huggingface.co/bigcode/starcoder), you will have to pass `-e HUGGING_FACE_HUB_TOKEN=<token>` to the `docker run` command above with a valid Hugging Face Hub read token.
|
||||
|
||||
For more information and documentation about Text Generation Inference, checkout [the README](https://github.com/huggingface/text-generation-inference#text-generation-inference) of the original repo.
|
||||
|
||||
Not all features of TGI are currently supported as this is still a work in progress.
|
||||
|
||||
New changes are added for the current release:
|
||||
- Sharded feature with support for DeepSpeed-inference auto tensor parallism. Also use HPU graph for performance improvement.
|
||||
- Torch profile.
|
||||
|
||||
|
||||
Enviroment Variables Added:
|
||||
|
||||
<div align="center">
|
||||
|
||||
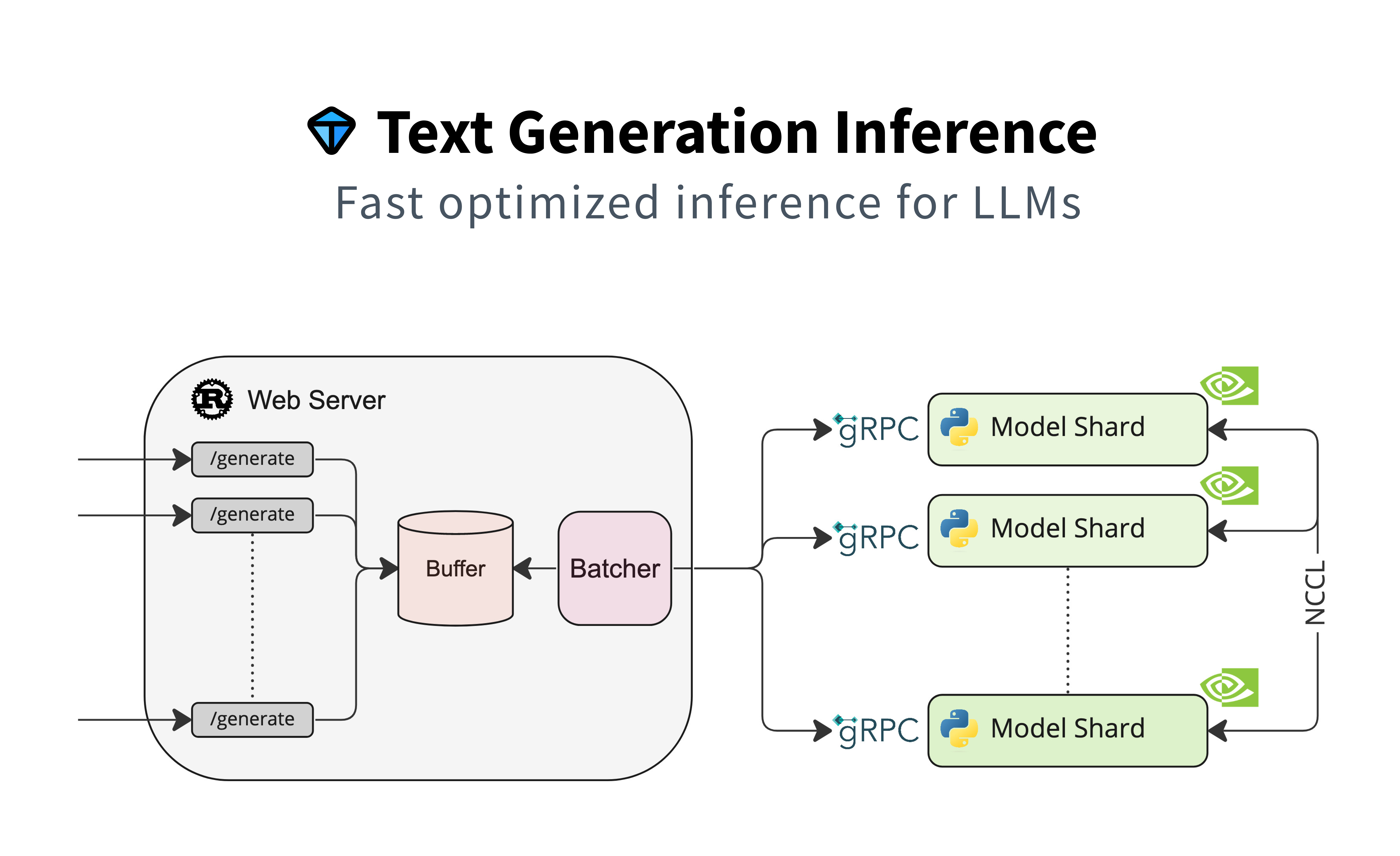
|
||||
|
||||
# Text Generation Inference
|
||||
|
||||
<a href="https://github.com/huggingface/text-generation-inference">
|
||||
<img alt="GitHub Repo stars" src="https://img.shields.io/github/stars/huggingface/text-generation-inference?style=social">
|
||||
</a>
|
||||
<a href="https://huggingface.github.io/text-generation-inference">
|
||||
<img alt="Swagger API documentation" src="https://img.shields.io/badge/API-Swagger-informational">
|
||||
</a>
|
||||
|
||||
A Rust, Python and gRPC server for text generation inference. Used in production at [HuggingFace](https://huggingface.co)
|
||||
to power Hugging Chat, the Inference API and Inference Endpoint.
|
||||
| Name | Value(s) | Default | Description | Usage |
|
||||
|------------------ |:---------------|:------------|:-------------------- |:---------------------------------
|
||||
| MAX_TOTAL_TOKENS | integer | 0 | Control the padding of input | add -e in docker run, such |
|
||||
| ENABLE_HPU_GRAPH | true/false | true | Enable hpu graph or not | add -e in docker run command |
|
||||
| PROF_WARMUPSTEP | integer | 0 | Enable/disable profile, control profile warmup step, 0 means disable profile | add -e in docker run command |
|
||||
| PROF_STEP | interger | 5 | Control profile step | add -e in docker run command |
|
||||
| PROF_PATH | string | /root/text-generation-inference | Define profile folder | add -e in docker run command |
|
||||
| LIMIT_HPU_GRAPH | True/False | False | Skip HPU graph usage for prefill to save memory | add -e in docker run command |
|
||||
|
||||
</div>
|
||||
|
||||
## Table of contents
|
||||
|
||||
- [Features](#features)
|
||||
- [Optimized Architectures](#optimized-architectures)
|
||||
- [Get Started](#get-started)
|
||||
- [Docker](#docker)
|
||||
- [API Documentation](#api-documentation)
|
||||
- [Using a private or gated model](#using-a-private-or-gated-model)
|
||||
- [A note on Shared Memory](#a-note-on-shared-memory-shm)
|
||||
- [Distributed Tracing](#distributed-tracing)
|
||||
- [Local Install](#local-install)
|
||||
- [CUDA Kernels](#cuda-kernels)
|
||||
- [Run Falcon](#run-falcon)
|
||||
- [Run](#run)
|
||||
- [Quantization](#quantization)
|
||||
- [Develop](#develop)
|
||||
- [Testing](#testing)
|
||||
- [Other supported hardware](#other-supported-hardware)
|
||||
|
||||
## Features
|
||||
|
||||
- Serve the most popular Large Language Models with a simple launcher
|
||||
- Tensor Parallelism for faster inference on multiple GPUs
|
||||
- Token streaming using Server-Sent Events (SSE)
|
||||
- [Continuous batching of incoming requests](https://github.com/huggingface/text-generation-inference/tree/main/router) for increased total throughput
|
||||
- Optimized transformers code for inference using [flash-attention](https://github.com/HazyResearch/flash-attention) and [Paged Attention](https://github.com/vllm-project/vllm) on the most popular architectures
|
||||
- Quantization with [bitsandbytes](https://github.com/TimDettmers/bitsandbytes) and [GPT-Q](https://arxiv.org/abs/2210.17323)
|
||||
- [Safetensors](https://github.com/huggingface/safetensors) weight loading
|
||||
- Watermarking with [A Watermark for Large Language Models](https://arxiv.org/abs/2301.10226)
|
||||
- Logits warper (temperature scaling, top-p, top-k, repetition penalty, more details see [transformers.LogitsProcessor](https://huggingface.co/docs/transformers/internal/generation_utils#transformers.LogitsProcessor))
|
||||
- Stop sequences
|
||||
- Log probabilities
|
||||
- Production ready (distributed tracing with Open Telemetry, Prometheus metrics)
|
||||
- Custom Prompt Generation: Easily generate text by providing custom prompts to guide the model's output.
|
||||
- Fine-tuning Support: Utilize fine-tuned models for specific tasks to achieve higher accuracy and performance.
|
||||
|
||||
|
||||
## Optimized architectures
|
||||
|
||||
- [BLOOM](https://huggingface.co/bigscience/bloom)
|
||||
- [FLAN-T5](https://huggingface.co/google/flan-t5-xxl)
|
||||
- [Galactica](https://huggingface.co/facebook/galactica-120b)
|
||||
- [GPT-Neox](https://huggingface.co/EleutherAI/gpt-neox-20b)
|
||||
- [Llama](https://github.com/facebookresearch/llama)
|
||||
- [OPT](https://huggingface.co/facebook/opt-66b)
|
||||
- [SantaCoder](https://huggingface.co/bigcode/santacoder)
|
||||
- [Starcoder](https://huggingface.co/bigcode/starcoder)
|
||||
- [Falcon 7B](https://huggingface.co/tiiuae/falcon-7b)
|
||||
- [Falcon 40B](https://huggingface.co/tiiuae/falcon-40b)
|
||||
- [MPT](https://huggingface.co/mosaicml/mpt-30b)
|
||||
- [Llama V2](https://huggingface.co/meta-llama)
|
||||
- [Code Llama](https://huggingface.co/codellama)
|
||||
- [Mistral](https://huggingface.co/mistralai/Mistral-7B-Instruct-v0.1)
|
||||
|
||||
Other architectures are supported on a best effort basis using:
|
||||
|
||||
`AutoModelForCausalLM.from_pretrained(<model>, device_map="auto")`
|
||||
|
||||
or
|
||||
|
||||
`AutoModelForSeq2SeqLM.from_pretrained(<model>, device_map="auto")`
|
||||
|
||||
## Get started
|
||||
|
||||
### Docker
|
||||
|
||||
The easiest way of getting started is using the official Docker container:
|
||||
|
||||
```shell
|
||||
model=tiiuae/falcon-7b-instruct
|
||||
volume=$PWD/data # share a volume with the Docker container to avoid downloading weights every run
|
||||
|
||||
docker run --gpus all --shm-size 1g -p 8080:80 -v $volume:/data ghcr.io/huggingface/text-generation-inference:1.1.0 --model-id $model
|
||||
```
|
||||
**Note:** To use GPUs, you need to install the [NVIDIA Container Toolkit](https://docs.nvidia.com/datacenter/cloud-native/container-toolkit/install-guide.html). We also recommend using NVIDIA drivers with CUDA version 11.8 or higher. For running the Docker container on a machine with no GPUs or CUDA support, it is enough to remove the `--gpus all` flag and add `--disable-custom-kernels`, please note CPU is not the intended platform for this project, so performance might be subpar.
|
||||
|
||||
To see all options to serve your models (in the [code](https://github.com/huggingface/text-generation-inference/blob/main/launcher/src/main.rs) or in the cli):
|
||||
```
|
||||
text-generation-launcher --help
|
||||
```
|
||||
|
||||
You can then query the model using either the `/generate` or `/generate_stream` routes:
|
||||
|
||||
```shell
|
||||
curl 127.0.0.1:8080/generate \
|
||||
-X POST \
|
||||
-d '{"inputs":"What is Deep Learning?","parameters":{"max_new_tokens":20}}' \
|
||||
-H 'Content-Type: application/json'
|
||||
```
|
||||
|
||||
```shell
|
||||
curl 127.0.0.1:8080/generate_stream \
|
||||
-X POST \
|
||||
-d '{"inputs":"What is Deep Learning?","parameters":{"max_new_tokens":20}}' \
|
||||
-H 'Content-Type: application/json'
|
||||
```
|
||||
|
||||
or from Python:
|
||||
|
||||
```shell
|
||||
pip install text-generation
|
||||
```
|
||||
|
||||
```python
|
||||
from text_generation import Client
|
||||
|
||||
client = Client("http://127.0.0.1:8080")
|
||||
print(client.generate("What is Deep Learning?", max_new_tokens=20).generated_text)
|
||||
|
||||
text = ""
|
||||
for response in client.generate_stream("What is Deep Learning?", max_new_tokens=20):
|
||||
if not response.token.special:
|
||||
text += response.token.text
|
||||
print(text)
|
||||
```
|
||||
|
||||
### API documentation
|
||||
|
||||
You can consult the OpenAPI documentation of the `text-generation-inference` REST API using the `/docs` route.
|
||||
The Swagger UI is also available at: [https://huggingface.github.io/text-generation-inference](https://huggingface.github.io/text-generation-inference).
|
||||
|
||||
### Using a private or gated model
|
||||
|
||||
You have the option to utilize the `HUGGING_FACE_HUB_TOKEN` environment variable for configuring the token employed by
|
||||
`text-generation-inference`. This allows you to gain access to protected resources.
|
||||
|
||||
For example, if you want to serve the gated Llama V2 model variants:
|
||||
|
||||
1. Go to https://huggingface.co/settings/tokens
|
||||
2. Copy your cli READ token
|
||||
3. Export `HUGGING_FACE_HUB_TOKEN=<your cli READ token>`
|
||||
|
||||
or with Docker:
|
||||
|
||||
```shell
|
||||
model=meta-llama/Llama-2-7b-chat-hf
|
||||
volume=$PWD/data # share a volume with the Docker container to avoid downloading weights every run
|
||||
token=<your cli READ token>
|
||||
|
||||
docker run --gpus all --shm-size 1g -e HUGGING_FACE_HUB_TOKEN=$token -p 8080:80 -v $volume:/data ghcr.io/huggingface/text-generation-inference:1.1.0 --model-id $model
|
||||
```
|
||||
|
||||
### A note on Shared Memory (shm)
|
||||
|
||||
[`NCCL`](https://docs.nvidia.com/deeplearning/nccl/user-guide/docs/index.html) is a communication framework used by
|
||||
`PyTorch` to do distributed training/inference. `text-generation-inference` make
|
||||
use of `NCCL` to enable Tensor Parallelism to dramatically speed up inference for large language models.
|
||||
|
||||
In order to share data between the different devices of a `NCCL` group, `NCCL` might fall back to using the host memory if
|
||||
peer-to-peer using NVLink or PCI is not possible.
|
||||
|
||||
To allow the container to use 1G of Shared Memory and support SHM sharing, we add `--shm-size 1g` on the above command.
|
||||
|
||||
If you are running `text-generation-inference` inside `Kubernetes`. You can also add Shared Memory to the container by
|
||||
creating a volume with:
|
||||
|
||||
```yaml
|
||||
- name: shm
|
||||
emptyDir:
|
||||
medium: Memory
|
||||
sizeLimit: 1Gi
|
||||
```
|
||||
|
||||
and mounting it to `/dev/shm`.
|
||||
|
||||
Finally, you can also disable SHM sharing by using the `NCCL_SHM_DISABLE=1` environment variable. However, note that
|
||||
this will impact performance.
|
||||
|
||||
### Distributed Tracing
|
||||
|
||||
`text-generation-inference` is instrumented with distributed tracing using OpenTelemetry. You can use this feature
|
||||
by setting the address to an OTLP collector with the `--otlp-endpoint` argument.
|
||||
|
||||
### Local install
|
||||
|
||||
You can also opt to install `text-generation-inference` locally.
|
||||
|
||||
First [install Rust](https://rustup.rs/) and create a Python virtual environment with at least
|
||||
Python 3.9, e.g. using `conda`:
|
||||
|
||||
```shell
|
||||
curl --proto '=https' --tlsv1.2 -sSf https://sh.rustup.rs | sh
|
||||
|
||||
conda create -n text-generation-inference python=3.9
|
||||
conda activate text-generation-inference
|
||||
```
|
||||
|
||||
You may also need to install Protoc.
|
||||
|
||||
On Linux:
|
||||
|
||||
```shell
|
||||
PROTOC_ZIP=protoc-21.12-linux-x86_64.zip
|
||||
curl -OL https://github.com/protocolbuffers/protobuf/releases/download/v21.12/$PROTOC_ZIP
|
||||
sudo unzip -o $PROTOC_ZIP -d /usr/local bin/protoc
|
||||
sudo unzip -o $PROTOC_ZIP -d /usr/local 'include/*'
|
||||
rm -f $PROTOC_ZIP
|
||||
```
|
||||
|
||||
On MacOS, using Homebrew:
|
||||
|
||||
```shell
|
||||
brew install protobuf
|
||||
```
|
||||
|
||||
Then run:
|
||||
|
||||
```shell
|
||||
BUILD_EXTENSIONS=True make install # Install repository and HF/transformer fork with CUDA kernels
|
||||
make run-falcon-7b-instruct
|
||||
```
|
||||
|
||||
**Note:** on some machines, you may also need the OpenSSL libraries and gcc. On Linux machines, run:
|
||||
|
||||
```shell
|
||||
sudo apt-get install libssl-dev gcc -y
|
||||
```
|
||||
|
||||
### CUDA Kernels
|
||||
|
||||
The custom CUDA kernels are only tested on NVIDIA A100s. If you have any installation or runtime issues, you can remove
|
||||
the kernels by using the `DISABLE_CUSTOM_KERNELS=True` environment variable.
|
||||
|
||||
Be aware that the official Docker image has them enabled by default.
|
||||
|
||||
## Run Falcon
|
||||
|
||||
### Run
|
||||
|
||||
```shell
|
||||
make run-falcon-7b-instruct
|
||||
```
|
||||
|
||||
### Quantization
|
||||
|
||||
You can also quantize the weights with bitsandbytes to reduce the VRAM requirement:
|
||||
|
||||
```shell
|
||||
make run-falcon-7b-instruct-quantize
|
||||
```
|
||||
|
||||
4bit quantization is available using the [NF4 and FP4 data types from bitsandbytes](https://arxiv.org/pdf/2305.14314.pdf). It can be enabled by providing `--quantize bitsandbytes-nf4` or `--quantize bitsandbytes-fp4` as a command line argument to `text-generation-launcher`.
|
||||
|
||||
## Develop
|
||||
|
||||
```shell
|
||||
make server-dev
|
||||
make router-dev
|
||||
```
|
||||
|
||||
## Testing
|
||||
|
||||
```shell
|
||||
# python
|
||||
make python-server-tests
|
||||
make python-client-tests
|
||||
# or both server and client tests
|
||||
make python-tests
|
||||
# rust cargo tests
|
||||
make rust-tests
|
||||
# integration tests
|
||||
make integration-tests
|
||||
```
|
||||
|
||||
|
||||
## Other supported hardware
|
||||
|
||||
TGI is also supported on the following AI hardware accelerators:
|
||||
- *Habana first-gen Gaudi and Gaudi2:* checkout [here](https://github.com/huggingface/optimum-habana/tree/main/text-generation-inference) how to serve models with TGI on Gaudi and Gaudi2 with [Optimum Habana](https://huggingface.co/docs/optimum/habana/index)
|
||||
|
||||
> The license to use TGI on Habana Gaudi is the one of TGI: https://github.com/huggingface/text-generation-inference/blob/main/LICENSE
|
||||
>
|
||||
> Please reach out to api-enterprise@huggingface.co if you have any question.
|
||||
|
@ -459,7 +459,9 @@ fn shard_manager(
|
||||
let mut envs: Vec<(OsString, OsString)> = env::vars_os().collect();
|
||||
|
||||
// Torch Distributed Env vars
|
||||
envs.push(("RANK".into(), rank.to_string().into()));
|
||||
if world_size == 1 {
|
||||
envs.push(("RANK".into(), rank.to_string().into()));
|
||||
}
|
||||
envs.push(("WORLD_SIZE".into(), world_size.to_string().into()));
|
||||
envs.push(("MASTER_ADDR".into(), master_addr.into()));
|
||||
envs.push(("MASTER_PORT".into(), master_port.to_string().into()));
|
||||
@ -870,7 +872,7 @@ fn spawn_shards(
|
||||
running: Arc<AtomicBool>,
|
||||
) -> Result<(), LauncherError> {
|
||||
// Start shard processes
|
||||
for rank in 0..num_shard {
|
||||
for rank in 0..1 {
|
||||
let model_id = args.model_id.clone();
|
||||
let revision = args.revision.clone();
|
||||
let uds_path = args.shard_uds_path.clone();
|
||||
@ -921,12 +923,12 @@ fn spawn_shards(
|
||||
drop(shutdown_sender);
|
||||
|
||||
// Wait for shard to start
|
||||
let mut shard_ready = 0;
|
||||
let mut shard_ready = 0;
|
||||
while running.load(Ordering::SeqCst) {
|
||||
match status_receiver.try_recv() {
|
||||
Ok(ShardStatus::Ready) => {
|
||||
shard_ready += 1;
|
||||
if shard_ready == num_shard {
|
||||
if shard_ready == 1 {
|
||||
break;
|
||||
}
|
||||
}
|
||||
|
@ -16,11 +16,7 @@ gen-server:
|
||||
find text_generation_server/pb/ -type f -name "*.py" -print0 -exec sed -i -e 's/^\(import.*pb2\)/from . \1/g' {} \;
|
||||
touch text_generation_server/pb/__init__.py
|
||||
|
||||
install-torch:
|
||||
# Install specific version of torch
|
||||
pip install torch --extra-index-url https://download.pytorch.org/whl/cu118 --no-cache-dir
|
||||
|
||||
install: gen-server install-torch
|
||||
install: gen-server
|
||||
pip install pip --upgrade
|
||||
pip install -r requirements.txt
|
||||
pip install -e ".[bnb, accelerate]"
|
||||
@ -28,5 +24,12 @@ install: gen-server install-torch
|
||||
run-dev:
|
||||
SAFETENSORS_FAST_GPU=1 python -m torch.distributed.run --nproc_per_node=2 text_generation_server/cli.py serve bigscience/bloom-560m --sharded
|
||||
|
||||
install-poetry:
|
||||
curl -sSL https://install.python-poetry.org | python3 -
|
||||
|
||||
update-lock:
|
||||
rm poetry.lock
|
||||
poetry lock --no-update
|
||||
|
||||
export-requirements:
|
||||
poetry export -o requirements.txt -E bnb -E quantize --without-hashes
|
||||
poetry export -f requirements.txt --without-hashes --output requirements.txt
|
||||
|
@ -9,52 +9,32 @@ text-generation-server = 'text_generation_server.cli:app'
|
||||
|
||||
[tool.poetry.dependencies]
|
||||
python = ">=3.9,<3.13"
|
||||
protobuf = "^4.21.7"
|
||||
protobuf = "^3.20.3"
|
||||
grpcio = "^1.51.1"
|
||||
grpcio-status = "^1.51.1"
|
||||
grpcio-reflection = "^1.51.1"
|
||||
grpcio-status = "*"
|
||||
grpcio-reflection = "*"
|
||||
grpc-interceptor = "^0.15.0"
|
||||
typer = "^0.6.1"
|
||||
accelerate = { version = "^0.20.0", optional = true }
|
||||
bitsandbytes = { version = "^0.41.1", optional = true }
|
||||
safetensors = "^0.3.2"
|
||||
safetensors = "0.3.2"
|
||||
loguru = "^0.6.0"
|
||||
opentelemetry-api = "^1.15.0"
|
||||
opentelemetry-exporter-otlp = "^1.15.0"
|
||||
opentelemetry-instrumentation-grpc = "^0.36b0"
|
||||
hf-transfer = "^0.1.2"
|
||||
sentencepiece = "^0.1.97"
|
||||
tokenizers = "^0.13.3"
|
||||
tokenizers = "^0.14.1"
|
||||
huggingface-hub = "^0.16.4"
|
||||
transformers = "^4.32.1"
|
||||
einops = "^0.6.1"
|
||||
texttable = { version = "^1.6.7", optional = true }
|
||||
datasets = { version = "^2.14.0", optional = true }
|
||||
peft = "^0.4.0"
|
||||
torch = { version = "^2.0.1" }
|
||||
scipy = "^1.11.1"
|
||||
pillow = "^10.0.0"
|
||||
|
||||
[tool.poetry.extras]
|
||||
accelerate = ["accelerate"]
|
||||
bnb = ["bitsandbytes"]
|
||||
quantize = ["texttable", "datasets", "accelerate"]
|
||||
deepspeed = { git = "https://github.com/HabanaAI/DeepSpeed.git", branch = "1.13.0" }
|
||||
optimum-habana = { git = "https://github.com/huggingface/optimum-habana.git", branch = "main" }
|
||||
|
||||
[tool.poetry.group.dev.dependencies]
|
||||
grpcio-tools = "^1.51.1"
|
||||
grpcio-tools = "*"
|
||||
pytest = "^7.3.0"
|
||||
|
||||
|
||||
[[tool.poetry.source]]
|
||||
name = "pytorch-gpu-src"
|
||||
url = "https://download.pytorch.org/whl/cu118"
|
||||
priority = "explicit"
|
||||
|
||||
[tool.pytest.ini_options]
|
||||
markers = ["private: marks tests as requiring an admin hf token (deselect with '-m \"not private\"')"]
|
||||
|
||||
[build-system]
|
||||
requires = [
|
||||
"poetry-core>=1.0.0",
|
||||
]
|
||||
requires = ["poetry-core>=1.0.0"]
|
||||
build-backend = "poetry.core.masonry.api"
|
||||
|
@ -1,30 +1,32 @@
|
||||
accelerate==0.20.3 ; python_version >= "3.9" and python_version < "3.13"
|
||||
accelerate>=0.22.0 ; python_version >= "3.9" and python_version < "3.13"
|
||||
aiohttp==3.8.5 ; python_version >= "3.9" and python_version < "3.13"
|
||||
aiosignal==1.3.1 ; python_version >= "3.9" and python_version < "3.13"
|
||||
async-timeout==4.0.3 ; python_version >= "3.9" and python_version < "3.13"
|
||||
attrs==23.1.0 ; python_version >= "3.9" and python_version < "3.13"
|
||||
backoff==2.2.1 ; python_version >= "3.9" and python_version < "3.13"
|
||||
bitsandbytes==0.41.1 ; python_version >= "3.9" and python_version < "3.13"
|
||||
certifi==2023.7.22 ; python_version >= "3.9" and python_version < "3.13"
|
||||
charset-normalizer==3.2.0 ; python_version >= "3.9" and python_version < "3.13"
|
||||
click==8.1.7 ; python_version >= "3.9" and python_version < "3.13"
|
||||
colorama==0.4.6 ; python_version >= "3.9" and python_version < "3.13" and (sys_platform == "win32" or platform_system == "Windows")
|
||||
datasets==2.14.5 ; python_version >= "3.9" and python_version < "3.13"
|
||||
coloredlogs==15.0.1 ; python_version >= "3.9" and python_version < "3.13"
|
||||
datasets==2.14.4 ; python_version >= "3.9" and python_version < "3.13"
|
||||
deprecated==1.2.14 ; python_version >= "3.9" and python_version < "3.13"
|
||||
diffusers==0.20.1 ; python_version >= "3.9" and python_version < "3.13"
|
||||
dill==0.3.7 ; python_version >= "3.9" and python_version < "3.13"
|
||||
einops==0.6.1 ; python_version >= "3.9" and python_version < "3.13"
|
||||
filelock==3.12.4 ; python_version >= "3.9" and python_version < "3.13"
|
||||
filelock==3.12.3 ; python_version >= "3.9" and python_version < "3.13"
|
||||
frozenlist==1.4.0 ; python_version >= "3.9" and python_version < "3.13"
|
||||
fsspec==2023.6.0 ; python_version >= "3.9" and python_version < "3.13"
|
||||
fsspec[http]==2023.6.0 ; python_version >= "3.9" and python_version < "3.13"
|
||||
googleapis-common-protos==1.60.0 ; python_version >= "3.9" and python_version < "3.13"
|
||||
grpc-interceptor==0.15.3 ; python_version >= "3.9" and python_version < "3.13"
|
||||
grpcio-reflection==1.58.0 ; python_version >= "3.9" and python_version < "3.13"
|
||||
grpcio-status==1.58.0 ; python_version >= "3.9" and python_version < "3.13"
|
||||
grpcio==1.58.0 ; python_version >= "3.9" and python_version < "3.13"
|
||||
grpcio-reflection==1.48.2 ; python_version >= "3.9" and python_version < "3.13"
|
||||
grpcio-status==1.48.2 ; python_version >= "3.9" and python_version < "3.13"
|
||||
grpcio==1.57.0 ; python_version >= "3.9" and python_version < "3.13"
|
||||
hf-transfer==0.1.3 ; python_version >= "3.9" and python_version < "3.13"
|
||||
huggingface-hub==0.16.4 ; python_version >= "3.9" and python_version < "3.13"
|
||||
humanfriendly==10.0 ; python_version >= "3.9" and python_version < "3.13"
|
||||
idna==3.4 ; python_version >= "3.9" and python_version < "3.13"
|
||||
importlib-metadata==6.8.0 ; python_version >= "3.9" and python_version < "3.13"
|
||||
jinja2==3.1.2 ; python_version >= "3.9" and python_version < "3.13"
|
||||
loguru==0.6.0 ; python_version >= "3.9" and python_version < "3.13"
|
||||
markupsafe==2.1.3 ; python_version >= "3.9" and python_version < "3.13"
|
||||
@ -32,7 +34,7 @@ mpmath==1.3.0 ; python_version >= "3.9" and python_version < "3.13"
|
||||
multidict==6.0.4 ; python_version >= "3.9" and python_version < "3.13"
|
||||
multiprocess==0.70.15 ; python_version >= "3.9" and python_version < "3.13"
|
||||
networkx==3.1 ; python_version >= "3.9" and python_version < "3.13"
|
||||
numpy==1.26.0 ; python_version >= "3.9" and python_version < "3.13"
|
||||
numpy==1.25.2 ; python_version >= "3.9" and python_version < "3.13"
|
||||
opentelemetry-api==1.15.0 ; python_version >= "3.9" and python_version < "3.13"
|
||||
opentelemetry-exporter-otlp-proto-grpc==1.15.0 ; python_version >= "3.9" and python_version < "3.13"
|
||||
opentelemetry-exporter-otlp-proto-http==1.15.0 ; python_version >= "3.9" and python_version < "3.13"
|
||||
@ -42,34 +44,35 @@ opentelemetry-instrumentation==0.36b0 ; python_version >= "3.9" and python_versi
|
||||
opentelemetry-proto==1.15.0 ; python_version >= "3.9" and python_version < "3.13"
|
||||
opentelemetry-sdk==1.15.0 ; python_version >= "3.9" and python_version < "3.13"
|
||||
opentelemetry-semantic-conventions==0.36b0 ; python_version >= "3.9" and python_version < "3.13"
|
||||
optimum==1.13.2 ; python_version >= "3.9" and python_version < "3.13"
|
||||
packaging==23.1 ; python_version >= "3.9" and python_version < "3.13"
|
||||
pandas==2.1.1 ; python_version >= "3.9" and python_version < "3.13"
|
||||
pandas==2.0.3 ; python_version >= "3.9" and python_version < "3.13"
|
||||
peft==0.4.0 ; python_version >= "3.9" and python_version < "3.13"
|
||||
pillow==10.0.1 ; python_version >= "3.9" and python_version < "3.13"
|
||||
protobuf==4.24.3 ; python_version >= "3.9" and python_version < "3.13"
|
||||
pillow==10.0.0 ; python_version >= "3.9" and python_version < "3.13"
|
||||
protobuf==3.20.3 ; python_version >= "3.9" and python_version < "3.13"
|
||||
psutil==5.9.5 ; python_version >= "3.9" and python_version < "3.13"
|
||||
pyarrow==13.0.0 ; python_version >= "3.9" and python_version < "3.13"
|
||||
pyreadline3==3.4.1 ; sys_platform == "win32" and python_version >= "3.9" and python_version < "3.13"
|
||||
python-dateutil==2.8.2 ; python_version >= "3.9" and python_version < "3.13"
|
||||
pytz==2023.3.post1 ; python_version >= "3.9" and python_version < "3.13"
|
||||
pytz==2023.3 ; python_version >= "3.9" and python_version < "3.13"
|
||||
pyyaml==6.0.1 ; python_version >= "3.9" and python_version < "3.13"
|
||||
regex==2023.8.8 ; python_version >= "3.9" and python_version < "3.13"
|
||||
requests==2.31.0 ; python_version >= "3.9" and python_version < "3.13"
|
||||
safetensors==0.3.3 ; python_version >= "3.9" and python_version < "3.13"
|
||||
scipy==1.11.2 ; python_version >= "3.9" and python_version < "3.13"
|
||||
safetensors==0.3.2 ; python_version >= "3.9" and python_version < "3.13"
|
||||
sentencepiece==0.1.99 ; python_version >= "3.9" and python_version < "3.13"
|
||||
setuptools==68.2.2 ; python_version >= "3.9" and python_version < "3.13"
|
||||
setuptools==68.1.2 ; python_version >= "3.9" and python_version < "3.13"
|
||||
six==1.16.0 ; python_version >= "3.9" and python_version < "3.13"
|
||||
sympy==1.12 ; python_version >= "3.9" and python_version < "3.13"
|
||||
texttable==1.6.7 ; python_version >= "3.9" and python_version < "3.13"
|
||||
tokenizers==0.13.3 ; python_version >= "3.9" and python_version < "3.13"
|
||||
torch==2.0.1 ; python_version >= "3.9" and python_version < "3.13"
|
||||
tokenizers==0.14.1 ; python_version >= "3.9" and python_version < "3.13"
|
||||
tqdm==4.66.1 ; python_version >= "3.9" and python_version < "3.13"
|
||||
transformers==4.33.2 ; python_version >= "3.9" and python_version < "3.13"
|
||||
transformers==4.34.1 ; python_version >= "3.9" and python_version < "3.13"
|
||||
transformers[sentencepiece]==4.34.1 ; python_version >= "3.9" and python_version < "3.13"
|
||||
typer==0.6.1 ; python_version >= "3.9" and python_version < "3.13"
|
||||
typing-extensions==4.8.0 ; python_version >= "3.9" and python_version < "3.13"
|
||||
typing-extensions==4.7.1 ; python_version >= "3.9" and python_version < "3.13"
|
||||
tzdata==2023.3 ; python_version >= "3.9" and python_version < "3.13"
|
||||
urllib3==2.0.5 ; python_version >= "3.9" and python_version < "3.13"
|
||||
urllib3==2.0.4 ; python_version >= "3.9" and python_version < "3.13"
|
||||
win32-setctime==1.1.0 ; python_version >= "3.9" and python_version < "3.13" and sys_platform == "win32"
|
||||
wrapt==1.15.0 ; python_version >= "3.9" and python_version < "3.13"
|
||||
xxhash==3.3.0 ; python_version >= "3.9" and python_version < "3.13"
|
||||
yarl==1.9.2 ; python_version >= "3.9" and python_version < "3.13"
|
||||
zipp==3.16.2 ; python_version >= "3.9" and python_version < "3.13"
|
||||
|
@ -6,7 +6,6 @@ from pathlib import Path
|
||||
from loguru import logger
|
||||
from typing import Optional
|
||||
from enum import Enum
|
||||
from huggingface_hub import hf_hub_download
|
||||
|
||||
|
||||
app = typer.Typer()
|
||||
@ -14,11 +13,7 @@ app = typer.Typer()
|
||||
|
||||
class Quantization(str, Enum):
|
||||
bitsandbytes = "bitsandbytes"
|
||||
bitsandbytes_nf4 = "bitsandbytes-nf4"
|
||||
bitsandbytes_fp4 = "bitsandbytes-fp4"
|
||||
gptq = "gptq"
|
||||
awq = "awq"
|
||||
eetq = "eetq"
|
||||
|
||||
|
||||
class Dtype(str, Enum):
|
||||
@ -40,18 +35,9 @@ def serve(
|
||||
otlp_endpoint: Optional[str] = None,
|
||||
):
|
||||
if sharded:
|
||||
assert (
|
||||
os.getenv("RANK", None) is not None
|
||||
), "RANK must be set when sharded is True"
|
||||
assert (
|
||||
os.getenv("WORLD_SIZE", None) is not None
|
||||
), "WORLD_SIZE must be set when sharded is True"
|
||||
assert (
|
||||
os.getenv("MASTER_ADDR", None) is not None
|
||||
), "MASTER_ADDR must be set when sharded is True"
|
||||
assert (
|
||||
os.getenv("MASTER_PORT", None) is not None
|
||||
), "MASTER_PORT must be set when sharded is True"
|
||||
assert os.getenv("WORLD_SIZE", None) is not None, "WORLD_SIZE must be set when sharded is True"
|
||||
assert os.getenv("MASTER_ADDR", None) is not None, "MASTER_ADDR must be set when sharded is True"
|
||||
assert os.getenv("MASTER_PORT", None) is not None, "MASTER_PORT must be set when sharded is True"
|
||||
|
||||
# Remove default handler
|
||||
logger.remove()
|
||||
@ -75,14 +61,29 @@ def serve(
|
||||
|
||||
# Downgrade enum into str for easier management later on
|
||||
quantize = None if quantize is None else quantize.value
|
||||
dtype = None if dtype is None else dtype.value
|
||||
if dtype is not None and quantize is not None:
|
||||
raise RuntimeError(
|
||||
"Only 1 can be set between `dtype` and `quantize`, as they both decide how goes the final model."
|
||||
)
|
||||
server.serve(
|
||||
model_id, revision, sharded, quantize, dtype, trust_remote_code, uds_path
|
||||
)
|
||||
dtype = "bfloat16" if dtype is None else dtype.value
|
||||
|
||||
logger.info("CLI SHARDED = {} DTYPE = {}".format(sharded, dtype))
|
||||
|
||||
if sharded:
|
||||
tgi_file = Path(__file__).resolve().parent / "tgi_service.py"
|
||||
num_shard = int(os.getenv("WORLD_SIZE", "1"))
|
||||
logger.info("CLI SHARDED = {}".format(num_shard))
|
||||
import subprocess
|
||||
|
||||
cmd = f"deepspeed --num_nodes 1 --num_gpus {num_shard} --no_local_rank {tgi_file} --model_id {model_id} --revision {revision} --sharded {sharded} --dtype {dtype} --uds_path {uds_path}"
|
||||
logger.info("CLI server start deepspeed ={} ".format(cmd))
|
||||
sys.stdout.flush()
|
||||
sys.stderr.flush()
|
||||
with subprocess.Popen(cmd, shell=True, executable="/bin/bash") as proc:
|
||||
proc.wait()
|
||||
sys.stdout.flush()
|
||||
sys.stderr.flush()
|
||||
if proc.returncode != 0:
|
||||
logger.error(f"{cmd} exited with status = {proc.returncode}")
|
||||
return proc.returncode
|
||||
else:
|
||||
server.serve(model_id, revision, dtype, uds_path, sharded)
|
||||
|
||||
|
||||
@app.command()
|
||||
@ -93,7 +94,6 @@ def download_weights(
|
||||
auto_convert: bool = True,
|
||||
logger_level: str = "INFO",
|
||||
json_output: bool = False,
|
||||
trust_remote_code: bool = False,
|
||||
):
|
||||
# Remove default handler
|
||||
logger.remove()
|
||||
@ -124,19 +124,6 @@ def download_weights(
|
||||
) is not None
|
||||
|
||||
if not is_local_model:
|
||||
try:
|
||||
adapter_config_filename = hf_hub_download(
|
||||
model_id, revision=revision, filename="adapter_config.json"
|
||||
)
|
||||
utils.download_and_unload_peft(
|
||||
model_id, revision, trust_remote_code=trust_remote_code
|
||||
)
|
||||
is_local_model = True
|
||||
utils.weight_files(model_id, revision, extension)
|
||||
return
|
||||
except (utils.LocalEntryNotFoundError, utils.EntryNotFoundError):
|
||||
pass
|
||||
|
||||
# Try to download weights from the hub
|
||||
try:
|
||||
filenames = utils.weight_hub_files(model_id, revision, extension)
|
||||
@ -175,30 +162,24 @@ def download_weights(
|
||||
)
|
||||
|
||||
# Safetensors final filenames
|
||||
local_st_files = [
|
||||
p.parent / f"{p.stem.lstrip('pytorch_')}.safetensors"
|
||||
for p in local_pt_files
|
||||
]
|
||||
local_st_files = [p.parent / f"{p.stem.lstrip('pytorch_')}.safetensors" for p in local_pt_files]
|
||||
try:
|
||||
import transformers
|
||||
import json
|
||||
from transformers import AutoConfig
|
||||
|
||||
if is_local_model:
|
||||
config_filename = os.path.join(model_id, "config.json")
|
||||
else:
|
||||
config_filename = hf_hub_download(
|
||||
model_id, revision=revision, filename="config.json"
|
||||
)
|
||||
with open(config_filename, "r") as f:
|
||||
config = json.load(f)
|
||||
architecture = config["architectures"][0]
|
||||
config = AutoConfig.from_pretrained(
|
||||
model_id,
|
||||
revision=revision,
|
||||
)
|
||||
architecture = config.architectures[0]
|
||||
|
||||
class_ = getattr(transformers, architecture)
|
||||
|
||||
# Name for this varible depends on transformers version.
|
||||
discard_names = getattr(class_, "_tied_weights_keys", [])
|
||||
discard_names.extend(getattr(class_, "_keys_to_ignore_on_load_missing", []))
|
||||
|
||||
except Exception as e:
|
||||
except Exception:
|
||||
discard_names = []
|
||||
# Convert pytorch weights to safetensors
|
||||
utils.convert_files(local_pt_files, local_st_files, discard_names)
|
||||
@ -216,8 +197,6 @@ def quantize(
|
||||
percdamp: float = 0.01,
|
||||
act_order: bool = False,
|
||||
):
|
||||
if revision is None:
|
||||
revision = "main"
|
||||
download_weights(
|
||||
model_id=model_id,
|
||||
revision=revision,
|
||||
@ -231,7 +210,6 @@ def quantize(
|
||||
bits=4,
|
||||
groupsize=128,
|
||||
output_dir=output_dir,
|
||||
revision=revision,
|
||||
trust_remote_code=trust_remote_code,
|
||||
upload_to_model_id=upload_to_model_id,
|
||||
percdamp=percdamp,
|
||||
|
@ -1,336 +1,35 @@
|
||||
import os
|
||||
import torch
|
||||
|
||||
from loguru import logger
|
||||
from transformers.configuration_utils import PretrainedConfig
|
||||
from transformers.models.auto import modeling_auto
|
||||
from transformers import AutoConfig
|
||||
from typing import Optional
|
||||
|
||||
from text_generation_server.models.model import Model
|
||||
from text_generation_server.models.causal_lm import CausalLM
|
||||
from text_generation_server.models.flash_causal_lm import FlashCausalLM
|
||||
from text_generation_server.models.bloom import BLOOMSharded
|
||||
from text_generation_server.models.mpt import MPTSharded
|
||||
from text_generation_server.models.seq2seq_lm import Seq2SeqLM
|
||||
from text_generation_server.models.rw import RW
|
||||
from text_generation_server.models.opt import OPTSharded
|
||||
from text_generation_server.models.galactica import GalacticaSharded
|
||||
from text_generation_server.models.bloom import BLOOM
|
||||
from text_generation_server.models.santacoder import SantaCoder
|
||||
from text_generation_server.models.t5 import T5Sharded
|
||||
from text_generation_server.models.gpt_neox import GPTNeoxSharded
|
||||
|
||||
# The flag below controls whether to allow TF32 on matmul. This flag defaults to False
|
||||
# in PyTorch 1.12 and later.
|
||||
torch.backends.cuda.matmul.allow_tf32 = True
|
||||
|
||||
# The flag below controls whether to allow TF32 on cuDNN. This flag defaults to True.
|
||||
torch.backends.cudnn.allow_tf32 = True
|
||||
|
||||
# Disable gradients
|
||||
torch.set_grad_enabled(False)
|
||||
|
||||
__all__ = [
|
||||
"Model",
|
||||
"BLOOMSharded",
|
||||
"CausalLM",
|
||||
"FlashCausalLM",
|
||||
"GalacticaSharded",
|
||||
"Seq2SeqLM",
|
||||
"SantaCoder",
|
||||
"OPTSharded",
|
||||
"T5Sharded",
|
||||
"get_model",
|
||||
]
|
||||
|
||||
FLASH_ATT_ERROR_MESSAGE = "{} requires Flash Attention enabled models."
|
||||
|
||||
FLASH_ATTENTION = True
|
||||
try:
|
||||
from text_generation_server.models.flash_rw import FlashRWSharded
|
||||
from text_generation_server.models.flash_neox import FlashNeoXSharded
|
||||
from text_generation_server.models.flash_llama import (
|
||||
FlashLlama,
|
||||
)
|
||||
from text_generation_server.models.flash_santacoder import (
|
||||
FlashSantacoderSharded,
|
||||
)
|
||||
from text_generation_server.models.idefics import IDEFICSSharded
|
||||
|
||||
except ImportError as e:
|
||||
logger.warning(f"Could not import Flash Attention enabled models: {e}")
|
||||
FLASH_ATTENTION = False
|
||||
|
||||
if FLASH_ATTENTION:
|
||||
__all__.append(FlashNeoXSharded)
|
||||
__all__.append(FlashRWSharded)
|
||||
__all__.append(FlashSantacoderSharded)
|
||||
__all__.append(FlashLlama)
|
||||
__all__.append(IDEFICSSharded)
|
||||
|
||||
MISTRAL = True
|
||||
try:
|
||||
from text_generation_server.models.flash_mistral import FlashMistral
|
||||
except ImportError as e:
|
||||
logger.warning(f"Could not import Mistral model: {e}")
|
||||
MISTRAL = False
|
||||
|
||||
if MISTRAL:
|
||||
__all__.append(FlashMistral)
|
||||
|
||||
|
||||
def get_model(
|
||||
model_id: str,
|
||||
revision: Optional[str],
|
||||
sharded: bool,
|
||||
quantize: Optional[str],
|
||||
dtype: Optional[str],
|
||||
trust_remote_code: bool,
|
||||
dtype: Optional[torch.dtype] = None,
|
||||
) -> Model:
|
||||
if dtype is None:
|
||||
dtype = torch.float16
|
||||
elif dtype == "float16":
|
||||
dtype = torch.float16
|
||||
elif dtype == "bfloat16":
|
||||
dtype = torch.bfloat16
|
||||
else:
|
||||
raise RuntimeError(f"Unknown dtype {dtype}")
|
||||
|
||||
if "facebook/galactica" in model_id:
|
||||
return GalacticaSharded(
|
||||
model_id,
|
||||
revision,
|
||||
quantize=quantize,
|
||||
dtype=dtype,
|
||||
trust_remote_code=trust_remote_code,
|
||||
)
|
||||
|
||||
if model_id.startswith("bigcode/"):
|
||||
if FLASH_ATTENTION:
|
||||
return FlashSantacoderSharded(
|
||||
model_id,
|
||||
revision,
|
||||
quantize=quantize,
|
||||
dtype=dtype,
|
||||
trust_remote_code=trust_remote_code,
|
||||
)
|
||||
elif sharded:
|
||||
raise NotImplementedError(
|
||||
FLASH_ATT_ERROR_MESSAGE.format("Sharded Santacoder")
|
||||
)
|
||||
else:
|
||||
return SantaCoder(
|
||||
model_id,
|
||||
revision,
|
||||
quantize=quantize,
|
||||
dtype=dtype,
|
||||
trust_remote_code=trust_remote_code,
|
||||
)
|
||||
|
||||
config_dict, _ = PretrainedConfig.get_config_dict(
|
||||
model_id, revision=revision, trust_remote_code=trust_remote_code
|
||||
)
|
||||
model_type = config_dict["model_type"]
|
||||
config = AutoConfig.from_pretrained(model_id, revision=revision)
|
||||
model_type = config.model_type
|
||||
|
||||
if model_type == "gpt_bigcode":
|
||||
if FLASH_ATTENTION:
|
||||
return FlashSantacoderSharded(
|
||||
model_id,
|
||||
revision,
|
||||
quantize=quantize,
|
||||
dtype=dtype,
|
||||
trust_remote_code=trust_remote_code,
|
||||
)
|
||||
elif sharded:
|
||||
raise NotImplementedError(
|
||||
FLASH_ATT_ERROR_MESSAGE.format("Sharded Santacoder")
|
||||
)
|
||||
else:
|
||||
return SantaCoder(
|
||||
model_id,
|
||||
revision,
|
||||
quantize=quantize,
|
||||
dtype=dtype,
|
||||
trust_remote_code=trust_remote_code,
|
||||
)
|
||||
return SantaCoder(model_id, revision, dtype)
|
||||
|
||||
if model_type == "bloom":
|
||||
return BLOOMSharded(
|
||||
model_id,
|
||||
revision,
|
||||
quantize=quantize,
|
||||
dtype=dtype,
|
||||
trust_remote_code=trust_remote_code,
|
||||
)
|
||||
elif model_type == "mpt":
|
||||
return MPTSharded(
|
||||
model_id,
|
||||
revision,
|
||||
quantize=quantize,
|
||||
dtype=dtype,
|
||||
trust_remote_code=trust_remote_code,
|
||||
)
|
||||
return BLOOM(model_id, revision, dtype)
|
||||
|
||||
elif model_type == "gpt_neox":
|
||||
if FLASH_ATTENTION:
|
||||
return FlashNeoXSharded(
|
||||
model_id,
|
||||
revision,
|
||||
quantize=quantize,
|
||||
dtype=dtype,
|
||||
trust_remote_code=trust_remote_code,
|
||||
)
|
||||
elif sharded:
|
||||
return GPTNeoxSharded(
|
||||
model_id,
|
||||
revision,
|
||||
quantize=quantize,
|
||||
dtype=dtype,
|
||||
trust_remote_code=trust_remote_code,
|
||||
)
|
||||
else:
|
||||
return CausalLM(
|
||||
model_id,
|
||||
revision,
|
||||
quantize=quantize,
|
||||
dtype=dtype,
|
||||
trust_remote_code=trust_remote_code,
|
||||
)
|
||||
|
||||
elif model_type == "llama" or model_type == "baichuan":
|
||||
if FLASH_ATTENTION:
|
||||
return FlashLlama(
|
||||
model_id,
|
||||
revision,
|
||||
quantize=quantize,
|
||||
dtype=dtype,
|
||||
trust_remote_code=trust_remote_code,
|
||||
)
|
||||
elif sharded:
|
||||
raise NotImplementedError(FLASH_ATT_ERROR_MESSAGE.format("Sharded Llama"))
|
||||
else:
|
||||
return CausalLM(
|
||||
model_id,
|
||||
revision,
|
||||
quantize=quantize,
|
||||
dtype=dtype,
|
||||
trust_remote_code=trust_remote_code,
|
||||
)
|
||||
|
||||
if model_type in ["RefinedWeb", "RefinedWebModel", "falcon"]:
|
||||
if sharded:
|
||||
if FLASH_ATTENTION:
|
||||
if config_dict.get("alibi", False):
|
||||
raise NotImplementedError("sharded is not supported for this model")
|
||||
return FlashRWSharded(
|
||||
model_id,
|
||||
revision,
|
||||
quantize=quantize,
|
||||
dtype=dtype,
|
||||
trust_remote_code=trust_remote_code,
|
||||
)
|
||||
raise NotImplementedError(FLASH_ATT_ERROR_MESSAGE.format(f"Sharded Falcon"))
|
||||
else:
|
||||
if FLASH_ATTENTION and not config_dict.get("alibi", False):
|
||||
return FlashRWSharded(
|
||||
model_id,
|
||||
revision,
|
||||
quantize=quantize,
|
||||
dtype=dtype,
|
||||
trust_remote_code=trust_remote_code,
|
||||
)
|
||||
else:
|
||||
return RW(
|
||||
model_id,
|
||||
revision,
|
||||
quantize=quantize,
|
||||
dtype=dtype,
|
||||
trust_remote_code=trust_remote_code,
|
||||
)
|
||||
|
||||
if model_type == "mistral":
|
||||
if MISTRAL:
|
||||
return FlashMistral(
|
||||
model_id,
|
||||
revision,
|
||||
quantize=quantize,
|
||||
dtype=dtype,
|
||||
trust_remote_code=trust_remote_code,
|
||||
)
|
||||
raise NotImplementedError("Mistral model requires flash attention v2")
|
||||
|
||||
if model_type == "opt":
|
||||
return OPTSharded(
|
||||
model_id,
|
||||
revision,
|
||||
quantize=quantize,
|
||||
dtype=dtype,
|
||||
trust_remote_code=trust_remote_code,
|
||||
)
|
||||
|
||||
if model_type == "t5":
|
||||
return T5Sharded(
|
||||
model_id,
|
||||
revision,
|
||||
quantize=quantize,
|
||||
dtype=dtype,
|
||||
trust_remote_code=trust_remote_code,
|
||||
)
|
||||
if model_type == "idefics":
|
||||
if FLASH_ATTENTION:
|
||||
return IDEFICSSharded(
|
||||
model_id,
|
||||
revision,
|
||||
quantize=quantize,
|
||||
dtype=dtype,
|
||||
trust_remote_code=trust_remote_code,
|
||||
)
|
||||
else:
|
||||
raise NotImplementedError(FLASH_ATT_ERROR_MESSAGE.format("Idefics"))
|
||||
|
||||
if sharded:
|
||||
raise ValueError("sharded is not supported for AutoModel")
|
||||
if quantize == "gptq":
|
||||
raise ValueError(
|
||||
"gptq quantization is not supported for AutoModel, you can try to quantize it with `text-generation-server quantize ORIGINAL_MODEL_ID NEW_MODEL_ID`"
|
||||
)
|
||||
if quantize == "awq":
|
||||
raise ValueError("awq quantization is not supported for AutoModel")
|
||||
elif (quantize == "bitsandbytes-fp4") or (quantize == "bitsandbytes-nf4"):
|
||||
raise ValueError("4bit quantization is not supported for AutoModel")
|
||||
if model_type in modeling_auto.MODEL_FOR_CAUSAL_LM_MAPPING_NAMES:
|
||||
return CausalLM(
|
||||
model_id,
|
||||
revision,
|
||||
quantize=quantize,
|
||||
dtype=dtype,
|
||||
trust_remote_code=trust_remote_code,
|
||||
)
|
||||
if model_type in modeling_auto.MODEL_FOR_SEQ_TO_SEQ_CAUSAL_LM_MAPPING_NAMES:
|
||||
return Seq2SeqLM(
|
||||
model_id,
|
||||
revision,
|
||||
quantize=quantize,
|
||||
dtype=dtype,
|
||||
trust_remote_code=trust_remote_code,
|
||||
)
|
||||
|
||||
auto_map = config_dict.get("auto_map", None)
|
||||
if trust_remote_code and auto_map is not None:
|
||||
if "AutoModelForCausalLM" in auto_map.keys():
|
||||
return CausalLM(
|
||||
model_id,
|
||||
revision,
|
||||
quantize=quantize,
|
||||
dtype=dtype,
|
||||
trust_remote_code=trust_remote_code,
|
||||
)
|
||||
if "AutoModelForSeq2SeqLM" in auto_map.keys():
|
||||
return Seq2SeqLM(
|
||||
model_id,
|
||||
revision,
|
||||
quantize=quantize,
|
||||
dtype=dtype,
|
||||
trust_remote_code=trust_remote_code,
|
||||
)
|
||||
return CausalLM(model_id, revision, dtype)
|
||||
|
||||
raise ValueError(f"Unsupported model type {model_type}")
|
||||
|
@ -1,25 +1,12 @@
|
||||
import torch
|
||||
import torch.distributed
|
||||
|
||||
from typing import Optional, Type
|
||||
|
||||
from transformers import (
|
||||
AutoTokenizer,
|
||||
AutoConfig,
|
||||
PreTrainedTokenizerBase,
|
||||
)
|
||||
from transformers import PreTrainedTokenizerBase
|
||||
|
||||
from text_generation_server.models.custom_modeling.bloom_modeling import (
|
||||
BloomForCausalLM,
|
||||
)
|
||||
from text_generation_server.models import CausalLM
|
||||
from text_generation_server.models.causal_lm import CausalLMBatch
|
||||
from text_generation_server.pb import generate_pb2
|
||||
from text_generation_server.utils import (
|
||||
initialize_torch_distributed,
|
||||
weight_files,
|
||||
Weights,
|
||||
)
|
||||
|
||||
|
||||
class BloomCausalLMBatch(CausalLMBatch):
|
||||
@ -30,82 +17,32 @@ class BloomCausalLMBatch(CausalLMBatch):
|
||||
tokenizer: PreTrainedTokenizerBase,
|
||||
dtype: torch.dtype,
|
||||
device: torch.device,
|
||||
is_optimized_for_gaudi: bool = False,
|
||||
) -> "CausalLMBatch":
|
||||
batch = super().from_pb(pb=pb, tokenizer=tokenizer, dtype=dtype, device=device)
|
||||
batch = super().from_pb(
|
||||
pb=pb,
|
||||
tokenizer=tokenizer,
|
||||
dtype=dtype,
|
||||
device=device,
|
||||
is_optimized_for_gaudi=is_optimized_for_gaudi,
|
||||
)
|
||||
batch.keys_head_dim_last = False
|
||||
return batch
|
||||
|
||||
|
||||
class BLOOMSharded(CausalLM):
|
||||
class BLOOM(CausalLM):
|
||||
def __init__(
|
||||
self,
|
||||
model_id: str,
|
||||
revision: Optional[str] = None,
|
||||
quantize: Optional[str] = None,
|
||||
dtype: Optional[torch.dtype] = None,
|
||||
trust_remote_code: bool = False,
|
||||
):
|
||||
self.process_group, rank, world_size = initialize_torch_distributed()
|
||||
if torch.cuda.is_available():
|
||||
device = torch.device(f"cuda:{rank}")
|
||||
dtype = torch.float16 if dtype is None else dtype
|
||||
else:
|
||||
device = torch.device("cpu")
|
||||
dtype = torch.float32 if dtype is None else dtype
|
||||
|
||||
tokenizer = AutoTokenizer.from_pretrained(
|
||||
model_id,
|
||||
super(BLOOM, self).__init__(
|
||||
model_id=model_id,
|
||||
revision=revision,
|
||||
padding_side="left",
|
||||
truncation_side="left",
|
||||
trust_remote_code=trust_remote_code,
|
||||
)
|
||||
|
||||
config = AutoConfig.from_pretrained(
|
||||
model_id,
|
||||
revision=revision,
|
||||
slow_but_exact=False,
|
||||
tp_parallel=True,
|
||||
trust_remote_code=trust_remote_code,
|
||||
)
|
||||
config.pad_token_id = 3
|
||||
config.quantize = quantize
|
||||
|
||||
torch.distributed.barrier(group=self.process_group)
|
||||
filenames = weight_files(model_id, revision=revision, extension=".safetensors")
|
||||
weights = Weights(
|
||||
filenames, device=device, dtype=dtype, process_group=self.process_group
|
||||
)
|
||||
if config.quantize == "gptq":
|
||||
weights._set_gptq_params(model_id)
|
||||
|
||||
model = BloomForCausalLM(config, weights)
|
||||
|
||||
torch.distributed.barrier(group=self.process_group)
|
||||
super(CausalLM, self).__init__(
|
||||
model=model,
|
||||
tokenizer=tokenizer,
|
||||
requires_padding=True,
|
||||
dtype=dtype,
|
||||
device=device,
|
||||
rank=rank,
|
||||
world_size=world_size,
|
||||
)
|
||||
|
||||
@property
|
||||
def batch_type(self) -> Type[CausalLMBatch]:
|
||||
return BloomCausalLMBatch
|
||||
|
||||
def forward(
|
||||
self, input_ids, attention_mask, position_ids, past_key_values: Optional = None
|
||||
):
|
||||
outputs = self.model.forward(
|
||||
input_ids=input_ids,
|
||||
attention_mask=attention_mask,
|
||||
position_ids=position_ids,
|
||||
past_key_values=past_key_values,
|
||||
use_cache=True,
|
||||
)
|
||||
|
||||
logits = outputs.logits
|
||||
return logits, outputs.past_key_values
|
||||
|
@ -1,11 +1,24 @@
|
||||
import os
|
||||
import tempfile
|
||||
|
||||
from text_generation_server.utils.tokens import batch_top_tokens
|
||||
import torch
|
||||
import inspect
|
||||
|
||||
from dataclasses import dataclass
|
||||
from opentelemetry import trace
|
||||
from transformers import AutoTokenizer, AutoModelForCausalLM, PreTrainedTokenizerBase
|
||||
from transformers import AutoTokenizer, AutoModelForCausalLM, PreTrainedTokenizerBase, AutoConfig
|
||||
from typing import Optional, Tuple, List, Type, Dict
|
||||
from habana_frameworks.torch.hpu import wrap_in_hpu_graph
|
||||
import habana_frameworks.torch as htorch
|
||||
from contextlib import nullcontext
|
||||
from optimum.habana.utils import HabanaProfile
|
||||
|
||||
from optimum.habana.transformers.generation import MODELS_OPTIMIZED_WITH_STATIC_SHAPES
|
||||
from optimum.habana.checkpoint_utils import (
|
||||
get_repo_root,
|
||||
model_on_meta,
|
||||
write_checkpoints_json,
|
||||
)
|
||||
|
||||
from text_generation_server.models import Model
|
||||
from text_generation_server.models.types import (
|
||||
@ -16,11 +29,11 @@ from text_generation_server.models.types import (
|
||||
TopTokens,
|
||||
)
|
||||
from text_generation_server.pb import generate_pb2
|
||||
from text_generation_server.utils import NextTokenChooser, StoppingCriteria, Sampling
|
||||
from text_generation_server.utils import HeterogeneousNextTokenChooser, StoppingCriteria, Sampling
|
||||
from loguru import logger
|
||||
|
||||
tracer = trace.get_tracer(__name__)
|
||||
|
||||
|
||||
@dataclass
|
||||
class CausalLMBatch(Batch):
|
||||
batch_id: int
|
||||
@ -42,7 +55,7 @@ class CausalLMBatch(Batch):
|
||||
read_offsets: List[int]
|
||||
|
||||
# Generation helpers
|
||||
next_token_choosers: List[NextTokenChooser]
|
||||
next_token_chooser: HeterogeneousNextTokenChooser
|
||||
stopping_criterias: List[StoppingCriteria]
|
||||
top_n_tokens: List[int]
|
||||
top_n_tokens_tensor: torch.Tensor
|
||||
@ -72,66 +85,90 @@ class CausalLMBatch(Batch):
|
||||
tokenizer: PreTrainedTokenizerBase,
|
||||
dtype: torch.dtype,
|
||||
device: torch.device,
|
||||
is_optimized_for_gaudi: bool = False,
|
||||
) -> "CausalLMBatch":
|
||||
inputs = []
|
||||
next_token_choosers = []
|
||||
next_token_chooser_parameters = []
|
||||
stopping_criterias = []
|
||||
top_n_tokens = []
|
||||
prefix_offsets = []
|
||||
read_offsets = []
|
||||
requests_idx_mapping = {}
|
||||
input_lengths = []
|
||||
|
||||
# Parse batch
|
||||
max_truncation = 0
|
||||
padding_right_offset = 0
|
||||
max_decode_tokens = 0
|
||||
|
||||
# TODO: this should be set to rust side `max_total_tokens`,
|
||||
# (see https://github.com/huggingface/text-generation-inference/blob/main/launcher/src/main.rs#L177)
|
||||
# but TGI does not offer an API to expose this variable to python, as this variable
|
||||
# is handled by the client but it appears the model is initialized by the server.
|
||||
# An alternative could be to initialize the buffers during warmup.
|
||||
# Dummy
|
||||
max_total_tokens = int(os.getenv("MAX_TOTAL_TOKENS", "0"))
|
||||
logger.info("MAX_TOTAL_TOKENS = {}".format(max_total_tokens))
|
||||
|
||||
for i, r in enumerate(pb.requests):
|
||||
requests_idx_mapping[r.id] = i
|
||||
inputs.append(r.inputs)
|
||||
next_token_choosers.append(NextTokenChooser.from_pb(r.parameters, device))
|
||||
stopping_criteria = StoppingCriteria.from_pb(
|
||||
r.stopping_parameters, tokenizer
|
||||
)
|
||||
next_token_chooser_parameters.append(r.parameters)
|
||||
stopping_criteria = StoppingCriteria.from_pb(r.stopping_parameters, tokenizer)
|
||||
stopping_criterias.append(stopping_criteria)
|
||||
top_n_tokens.append(r.top_n_tokens)
|
||||
max_truncation = max(max_truncation, r.truncate)
|
||||
max_decode_tokens += stopping_criteria.max_new_tokens
|
||||
padding_right_offset = max(
|
||||
padding_right_offset, stopping_criteria.max_new_tokens
|
||||
)
|
||||
padding_right_offset = max(padding_right_offset, stopping_criteria.max_new_tokens)
|
||||
|
||||
next_token_chooser = HeterogeneousNextTokenChooser.from_pb(
|
||||
next_token_chooser_parameters, dtype, device
|
||||
)
|
||||
|
||||
tokenized_inputs = tokenizer(
|
||||
inputs,
|
||||
return_tensors="pt",
|
||||
padding=True,
|
||||
padding="max_length",
|
||||
return_token_type_ids=False,
|
||||
truncation=True,
|
||||
max_length=max_truncation,
|
||||
).to(device)
|
||||
)
|
||||
|
||||
for _ in pb.requests:
|
||||
input_len = tokenized_inputs["input_ids"].shape[1]
|
||||
input_lengths.append(input_len)
|
||||
prefix_offsets.append(input_len - 5)
|
||||
read_offsets.append(input_len)
|
||||
|
||||
input_lengths = tokenized_inputs["attention_mask"].sum(1)
|
||||
max_input_length = input_lengths.max()
|
||||
max_input_length = max(input_lengths)
|
||||
if max_total_tokens == 0:
|
||||
max_total_tokens = max_input_length
|
||||
max_tokens = len(inputs) * max_input_length + max_decode_tokens
|
||||
if is_optimized_for_gaudi and max_total_tokens > max_input_length:
|
||||
# pad to max_total_tokens in case max_new_token changes per request and triggers new hpu graph generation
|
||||
padding_right_offset = max_total_tokens - max_input_length
|
||||
|
||||
input_ids = tokenized_inputs["input_ids"]
|
||||
# Allocate maximum attention_mask
|
||||
attention_mask = input_ids.new_zeros(
|
||||
(pb.size, max_input_length + padding_right_offset)
|
||||
)
|
||||
# Copy tokenizer attention_mask into fully allocated attention_mask
|
||||
attention_mask[:, :max_input_length] = tokenized_inputs["attention_mask"]
|
||||
attention_mask = tokenized_inputs["attention_mask"]
|
||||
# only move model inputs to device
|
||||
attention_mask = attention_mask.to(device)
|
||||
|
||||
position_ids = tokenized_inputs["attention_mask"].long().cumsum(-1) - 1
|
||||
position_ids.masked_fill_(tokenized_inputs["attention_mask"] == 0, 1)
|
||||
all_input_ids = tokenized_inputs["input_ids"].T.split(1, dim=1)
|
||||
top_n_tokens_tensor = torch.tensor(
|
||||
top_n_tokens, device=device, dtype=torch.int64
|
||||
)
|
||||
if is_optimized_for_gaudi:
|
||||
input_ids_cpu = torch.nn.functional.pad(
|
||||
input_ids, (0, padding_right_offset), value=tokenizer.pad_token_id
|
||||
)
|
||||
input_ids = input_ids_cpu.to(device)
|
||||
attention_mask = torch.nn.functional.pad(attention_mask, (0, padding_right_offset), value=0)
|
||||
all_input_ids = input_ids_cpu.T.split(1, dim=1)
|
||||
else:
|
||||
all_input_ids = input_ids.clone().T.split(1, dim=1)
|
||||
input_ids = input_ids.to(device)
|
||||
|
||||
max_tokens = len(inputs) * (max_input_length + max_decode_tokens)
|
||||
position_ids = attention_mask.long().cumsum(-1) - 1
|
||||
position_ids.masked_fill_(attention_mask == 0, 1)
|
||||
htorch.core.mark_step()
|
||||
|
||||
top_n_tokens_tensor = torch.tensor(top_n_tokens, device=device, dtype=torch.int64)
|
||||
|
||||
return cls(
|
||||
batch_id=pb.id,
|
||||
@ -142,20 +179,20 @@ class CausalLMBatch(Batch):
|
||||
position_ids=position_ids,
|
||||
past_key_values=None,
|
||||
all_input_ids=list(all_input_ids),
|
||||
input_lengths=input_lengths.tolist(),
|
||||
input_lengths=input_lengths,
|
||||
prefix_offsets=prefix_offsets,
|
||||
read_offsets=read_offsets,
|
||||
next_token_choosers=next_token_choosers,
|
||||
next_token_chooser=next_token_chooser,
|
||||
stopping_criterias=stopping_criterias,
|
||||
top_n_tokens=top_n_tokens,
|
||||
top_n_tokens_tensor=top_n_tokens_tensor,
|
||||
max_input_length=max_input_length.item(),
|
||||
max_input_length=max_input_length,
|
||||
padding_right_offset=padding_right_offset,
|
||||
max_tokens=max_tokens,
|
||||
)
|
||||
|
||||
@tracer.start_as_current_span("filter")
|
||||
def filter(self, request_ids: List[int]) -> Optional["CausalLMBatch"]:
|
||||
def filter(self, request_ids: List[int], is_optimized_for_gaudi: bool = False) -> Optional["CausalLMBatch"]:
|
||||
if len(request_ids) == 0:
|
||||
raise ValueError("Batch must have at least one request")
|
||||
if len(request_ids) == len(self):
|
||||
@ -172,7 +209,6 @@ class CausalLMBatch(Batch):
|
||||
all_input_ids = []
|
||||
max_input_length = 0
|
||||
|
||||
next_token_choosers = []
|
||||
stopping_criterias = []
|
||||
top_n_tokens = []
|
||||
|
||||
@ -193,52 +229,66 @@ class CausalLMBatch(Batch):
|
||||
input_lengths.append(request_input_length)
|
||||
max_input_length = max(max_input_length, request_input_length)
|
||||
|
||||
next_token_choosers.append(self.next_token_choosers[idx])
|
||||
stopping_criteria = self.stopping_criterias[idx]
|
||||
stopping_criterias.append(stopping_criteria)
|
||||
top_n_tokens.append(self.top_n_tokens[idx])
|
||||
remaining_decode_tokens = (
|
||||
stopping_criteria.max_new_tokens - stopping_criteria.current_tokens
|
||||
)
|
||||
remaining_decode_tokens = stopping_criteria.max_new_tokens - stopping_criteria.current_tokens
|
||||
total_remaining_decode_tokens += remaining_decode_tokens
|
||||
new_padding_right_offset = max(
|
||||
new_padding_right_offset, remaining_decode_tokens
|
||||
)
|
||||
new_padding_right_offset = max(new_padding_right_offset, remaining_decode_tokens)
|
||||
|
||||
# Apply indices to input_ids, attention mask, past key values and other items that need to be cached
|
||||
input_ids = self.input_ids[keep_indices]
|
||||
position_ids = self.position_ids[keep_indices]
|
||||
self.attention_mask = self.attention_mask[
|
||||
keep_indices,
|
||||
-(self.padding_right_offset + max_input_length) : (
|
||||
self.attention_mask.shape[1] - self.padding_right_offset
|
||||
)
|
||||
+ new_padding_right_offset,
|
||||
]
|
||||
next_token_chooser = self.next_token_chooser.filter(keep_indices)
|
||||
if is_optimized_for_gaudi:
|
||||
self.attention_mask = self.attention_mask[keep_indices]
|
||||
else:
|
||||
self.attention_mask = self.attention_mask[
|
||||
keep_indices,
|
||||
-(self.padding_right_offset + max_input_length) : (
|
||||
self.attention_mask.shape[1] - self.padding_right_offset
|
||||
)
|
||||
+ new_padding_right_offset,
|
||||
]
|
||||
|
||||
# Ensure that past_key_values tensors can be updated in-place
|
||||
kv_tuple = False
|
||||
if type(self.past_key_values[0]) == tuple:
|
||||
self.past_key_values = [list(layer) for layer in self.past_key_values]
|
||||
kv_tuple = True
|
||||
|
||||
# Update tensors in-place to allow incremental garbage collection
|
||||
past_kv_length = max_input_length - 1
|
||||
for layer in self.past_key_values:
|
||||
past_keys, past_values = layer
|
||||
if len(past_keys.shape) == 3:
|
||||
past_keys_dims = len(past_keys.shape)
|
||||
if past_keys_dims == 3:
|
||||
# Force past to be of dim [self_size, num_heads, ...] for easy indexing
|
||||
past_keys = past_keys.view(len(self), -1, *past_keys.shape[-2:])
|
||||
past_values = past_values.view(len(self), -1, *past_values.shape[-2:])
|
||||
if self.keys_head_dim_last:
|
||||
layer[0] = past_keys[keep_indices, :, -past_kv_length:, :]
|
||||
if is_optimized_for_gaudi:
|
||||
layer[0] = past_keys[keep_indices]
|
||||
del past_keys
|
||||
layer[1] = past_values[keep_indices]
|
||||
del past_values
|
||||
else:
|
||||
layer[0] = past_keys[keep_indices, :, :, -past_kv_length:]
|
||||
del past_keys
|
||||
layer[1] = past_values[keep_indices, :, -past_kv_length:, :]
|
||||
del past_values
|
||||
if self.keys_head_dim_last:
|
||||
layer[0] = past_keys[keep_indices, :, -past_kv_length:, :]
|
||||
else:
|
||||
layer[0] = past_keys[keep_indices, :, :, -past_kv_length:]
|
||||
del past_keys
|
||||
layer[1] = past_values[keep_indices, :, -past_kv_length:, :]
|
||||
del past_values
|
||||
if past_keys_dims == 3:
|
||||
layer[0] = layer[0].view(layer[0].shape[0] * layer[0].shape[1], *layer[0].shape[-2:])
|
||||
layer[1] = layer[1].view(layer[1].shape[0] * layer[1].shape[1], *layer[1].shape[-2:])
|
||||
|
||||
top_n_tokens_tensor = self.top_n_tokens_tensor[keep_indices]
|
||||
max_tokens = len(request_ids) * max_input_length + total_remaining_decode_tokens
|
||||
|
||||
if kv_tuple:
|
||||
self.past_key_values = [tuple(layer) for layer in self.past_key_values]
|
||||
|
||||
self.requests = requests
|
||||
self.requests_idx_mapping = requests_idx_mapping
|
||||
self.input_ids = input_ids
|
||||
@ -247,7 +297,7 @@ class CausalLMBatch(Batch):
|
||||
self.input_lengths = input_lengths
|
||||
self.prefix_offsets = prefix_offsets
|
||||
self.read_offsets = read_offsets
|
||||
self.next_token_choosers = next_token_choosers
|
||||
self.next_token_chooser = next_token_chooser
|
||||
self.stopping_criterias = stopping_criterias
|
||||
self.top_n_tokens = top_n_tokens
|
||||
self.top_n_tokens_tensor = top_n_tokens_tensor
|
||||
@ -259,15 +309,20 @@ class CausalLMBatch(Batch):
|
||||
|
||||
@classmethod
|
||||
@tracer.start_as_current_span("concatenate")
|
||||
def concatenate(cls, batches: List["CausalLMBatch"]) -> "CausalLMBatch":
|
||||
def concatenate(cls, batches: List["CausalLMBatch"], is_optimized_for_gaudi: bool = False) -> "CausalLMBatch":
|
||||
# Used for padding
|
||||
total_batch_size = 0
|
||||
max_input_length = 0
|
||||
padding_right_offset = 0
|
||||
max_total_tokens = 0
|
||||
for batch in batches:
|
||||
total_batch_size += len(batch)
|
||||
max_input_length = max(max_input_length, batch.max_input_length)
|
||||
padding_right_offset = max(padding_right_offset, batch.padding_right_offset)
|
||||
max_total_tokens = max(max_total_tokens, batch.max_input_length + batch.padding_right_offset)
|
||||
|
||||
if is_optimized_for_gaudi and max_total_tokens > max_input_length:
|
||||
padding_right_offset = max_total_tokens - max_input_length
|
||||
|
||||
# Batch attributes
|
||||
requests = []
|
||||
@ -276,7 +331,7 @@ class CausalLMBatch(Batch):
|
||||
prefix_offsets = []
|
||||
read_offsets = []
|
||||
all_input_ids = []
|
||||
next_token_choosers = []
|
||||
next_token_chooser_parameters = []
|
||||
stopping_criterias = []
|
||||
top_n_tokens = []
|
||||
max_tokens = 0
|
||||
@ -297,7 +352,7 @@ class CausalLMBatch(Batch):
|
||||
prefix_offsets.extend(batch.prefix_offsets)
|
||||
read_offsets.extend(batch.read_offsets)
|
||||
all_input_ids.extend(batch.all_input_ids)
|
||||
next_token_choosers.extend(batch.next_token_choosers)
|
||||
next_token_chooser_parameters.extend([r.parameters for r in batch.requests])
|
||||
stopping_criterias.extend(batch.stopping_criterias)
|
||||
top_n_tokens.extend(batch.top_n_tokens)
|
||||
|
||||
@ -338,15 +393,8 @@ class CausalLMBatch(Batch):
|
||||
# We need to slice the attention mask to remove padding from previous steps
|
||||
# and to remove unused allocated space
|
||||
left_offset = max_input_length - batch.max_input_length
|
||||
batch_left_offset = (
|
||||
batch.attention_mask.shape[1]
|
||||
- batch.max_input_length
|
||||
- batch.padding_right_offset
|
||||
)
|
||||
attention_mask[
|
||||
start_index:end_index,
|
||||
left_offset:-padding_right_offset,
|
||||
] = batch.attention_mask[
|
||||
batch_left_offset = batch.attention_mask.shape[1] - batch.max_input_length - batch.padding_right_offset
|
||||
attention_mask[start_index:end_index, left_offset:-padding_right_offset] = batch.attention_mask[
|
||||
:,
|
||||
batch_left_offset : -batch.padding_right_offset,
|
||||
]
|
||||
@ -361,30 +409,38 @@ class CausalLMBatch(Batch):
|
||||
# BLOOM Keys: [batch_size * num_heads, head_dim, seq_length]
|
||||
# BLOOM Values: [batch_size * num_heads, seq_length, head_dim]
|
||||
# And ensure that we can update tensors in-place
|
||||
kv_tuple = False
|
||||
past_key_values_dims = len(batch.past_key_values[0][0].shape)
|
||||
if type(batch.past_key_values[0]) == tuple:
|
||||
batch.past_key_values = [
|
||||
[t.view(len(batch), -1, *t.shape[-2:]) for t in layer]
|
||||
for layer in batch.past_key_values
|
||||
[t.view(len(batch), -1, *t.shape[-2:]) for t in layer] for layer in batch.past_key_values
|
||||
]
|
||||
elif len(batch.past_key_values[0][0].shape) == 3:
|
||||
kv_tuple = True
|
||||
elif past_key_values_dims == 3:
|
||||
for layer in batch.past_key_values:
|
||||
for k, t in enumerate(layer):
|
||||
layer[k] = t.view(len(batch), -1, *t.shape[-2:])
|
||||
|
||||
# Add eventual padding tokens that were added while concatenating
|
||||
max_tokens += batch.max_tokens + (
|
||||
max_input_length - batch.max_input_length
|
||||
) * len(batch)
|
||||
max_tokens += batch.max_tokens + (max_input_length - batch.max_input_length) * len(batch)
|
||||
|
||||
start_index = end_index
|
||||
|
||||
first_past_kvs = batches[0].past_key_values
|
||||
_, num_heads, padded_sequence_length, head_dim = first_past_kvs[0][1].shape
|
||||
next_token_chooser = HeterogeneousNextTokenChooser.from_pb(
|
||||
next_token_chooser_parameters,
|
||||
dtype=batches[0].next_token_chooser.dtype,
|
||||
device=batches[0].next_token_chooser.device,
|
||||
)
|
||||
|
||||
first_past_kvs = batches[0].past_key_values
|
||||
_, num_heads, _, head_dim = first_past_kvs[0][1].shape
|
||||
padded_sequence_length = (
|
||||
max_input_length + padding_right_offset if is_optimized_for_gaudi else max_input_length - 1
|
||||
)
|
||||
padded_past_values_shape = (
|
||||
total_batch_size,
|
||||
num_heads,
|
||||
max_input_length - 1,
|
||||
padded_sequence_length,
|
||||
head_dim,
|
||||
)
|
||||
|
||||
@ -396,7 +452,7 @@ class CausalLMBatch(Batch):
|
||||
total_batch_size,
|
||||
num_heads,
|
||||
head_dim,
|
||||
max_input_length - 1,
|
||||
padded_sequence_length,
|
||||
)
|
||||
|
||||
# Iterate over attention layers
|
||||
@ -413,22 +469,24 @@ class CausalLMBatch(Batch):
|
||||
end_index = start_index + len(batch)
|
||||
# We slice the keys to remove the padding from previous batches
|
||||
past_seq_len = batch.max_input_length - 1
|
||||
# recaculate the offset
|
||||
left_offset = max_input_length - batch.max_input_length
|
||||
batch_left_offset = batch.attention_mask.shape[1] - batch.max_input_length - batch.padding_right_offset
|
||||
|
||||
if batch.keys_head_dim_last:
|
||||
padded_past_keys[
|
||||
start_index:end_index, :, -past_seq_len:, :
|
||||
] = past_keys[:, :, -past_seq_len:, :]
|
||||
start_index:end_index, :, left_offset : left_offset + past_seq_len, :
|
||||
] = past_keys[:, :, batch_left_offset : batch_left_offset + past_seq_len, :]
|
||||
else:
|
||||
# BLOOM case
|
||||
padded_past_keys[
|
||||
start_index:end_index, :, :, -past_seq_len:
|
||||
] = past_keys[:, :, :, -past_seq_len:]
|
||||
start_index:end_index, :, :, left_offset : left_offset + past_seq_len
|
||||
] = past_keys[:, :, :, batch_left_offset : batch_left_offset + past_seq_len]
|
||||
del past_keys
|
||||
|
||||
start_index = end_index
|
||||
|
||||
padded_past_values = first_past_kvs[j][1].new_zeros(
|
||||
padded_past_values_shape
|
||||
)
|
||||
padded_past_values = first_past_kvs[j][1].new_zeros(padded_past_values_shape)
|
||||
start_index = 0
|
||||
for batch in batches:
|
||||
past_values = batch.past_key_values[j][1]
|
||||
@ -439,15 +497,30 @@ class CausalLMBatch(Batch):
|
||||
end_index = start_index + len(batch)
|
||||
# We slice the past values to remove the padding from previous batches
|
||||
past_seq_len = batch.max_input_length - 1
|
||||
# recaculate the offset
|
||||
left_offset = max_input_length - batch.max_input_length
|
||||
batch_left_offset = batch.attention_mask.shape[1] - batch.max_input_length - batch.padding_right_offset
|
||||
|
||||
padded_past_values[
|
||||
start_index:end_index, :, -past_seq_len:, :
|
||||
] = past_values[:, :, -past_seq_len:, :]
|
||||
start_index:end_index, :, left_offset : left_offset + past_seq_len, :
|
||||
] = past_values[:, :, batch_left_offset : batch_left_offset + past_seq_len, :]
|
||||
del past_values
|
||||
|
||||
# Update values
|
||||
start_index = end_index
|
||||
|
||||
past_key_values.append([padded_past_keys, padded_past_values])
|
||||
if past_key_values_dims == 3:
|
||||
padded_past_keys = padded_past_keys.view(
|
||||
padded_past_keys.shape[0] * padded_past_keys.shape[1], *padded_past_keys.shape[-2:]
|
||||
)
|
||||
padded_past_values = padded_past_values.view(
|
||||
padded_past_values.shape[0] * padded_past_values.shape[1], *padded_past_values.shape[-2:]
|
||||
)
|
||||
|
||||
if kv_tuple:
|
||||
past_key_values.append((padded_past_keys, padded_past_values))
|
||||
else:
|
||||
past_key_values.append([padded_past_keys, padded_past_values])
|
||||
|
||||
return cls(
|
||||
batch_id=batches[0].batch_id,
|
||||
@ -461,7 +534,7 @@ class CausalLMBatch(Batch):
|
||||
input_lengths=input_lengths,
|
||||
prefix_offsets=prefix_offsets,
|
||||
read_offsets=read_offsets,
|
||||
next_token_choosers=next_token_choosers,
|
||||
next_token_chooser=next_token_chooser,
|
||||
stopping_criterias=stopping_criterias,
|
||||
top_n_tokens=top_n_tokens,
|
||||
top_n_tokens_tensor=top_n_tokens_tensor,
|
||||
@ -480,39 +553,88 @@ class CausalLM(Model):
|
||||
self,
|
||||
model_id: str,
|
||||
revision: Optional[str] = None,
|
||||
quantize: Optional[str] = None,
|
||||
dtype: Optional[torch.dtype] = None,
|
||||
trust_remote_code: bool = False,
|
||||
):
|
||||
if torch.cuda.is_available():
|
||||
device = torch.device("cuda")
|
||||
dtype = torch.float16 if dtype is None else dtype
|
||||
else:
|
||||
if quantize:
|
||||
raise ValueError("quantization is not available on CPU")
|
||||
device = torch.device("hpu")
|
||||
|
||||
device = torch.device("cpu")
|
||||
dtype = torch.float32 if dtype is None else dtype
|
||||
dtype = torch.bfloat16 if dtype is None else dtype
|
||||
|
||||
from optimum.habana.transformers.modeling_utils import adapt_transformers_to_gaudi
|
||||
|
||||
adapt_transformers_to_gaudi()
|
||||
|
||||
tokenizer = AutoTokenizer.from_pretrained(
|
||||
model_id,
|
||||
revision=revision,
|
||||
padding_side="left",
|
||||
truncation_side="left",
|
||||
trust_remote_code=trust_remote_code,
|
||||
)
|
||||
model = AutoModelForCausalLM.from_pretrained(
|
||||
model_id,
|
||||
revision=revision,
|
||||
torch_dtype=dtype,
|
||||
device_map="auto"
|
||||
if torch.cuda.is_available() and torch.cuda.device_count() > 1
|
||||
else None,
|
||||
load_in_8bit=quantize == "bitsandbytes",
|
||||
trust_remote_code=trust_remote_code,
|
||||
)
|
||||
if torch.cuda.is_available() and torch.cuda.device_count() == 1:
|
||||
model = model.cuda()
|
||||
|
||||
model_kwargs = {
|
||||
"revision": revision,
|
||||
}
|
||||
|
||||
world_size = int(os.getenv("WORLD_SIZE", "1"))
|
||||
rank = int(os.getenv("RANK"), 0)
|
||||
self.enable_hpu_graph = os.getenv("ENABLE_HPU_GRAPH", "true").lower() == "true"
|
||||
self.limit_hpu_graph = os.getenv("LIMIT_HPU_GRAPH", "false").lower() == "true"
|
||||
|
||||
if world_size > 1:
|
||||
import habana_frameworks.torch.hpu as torch_hpu
|
||||
|
||||
# Get world size, rank and local rank
|
||||
from habana_frameworks.torch.distributed.hccl import initialize_distributed_hpu
|
||||
|
||||
world_size, rank, local_rank = initialize_distributed_hpu()
|
||||
import deepspeed
|
||||
|
||||
# Initialize process(es) for DeepSpeed
|
||||
deepspeed.init_distributed(dist_backend="hccl")
|
||||
logger.info(
|
||||
"DeepSpeed is enabled. world_size {} rank {} local_rank {}".format(world_size, rank, local_rank)
|
||||
)
|
||||
config = AutoConfig.from_pretrained(model_id, **model_kwargs)
|
||||
load_to_meta = model_on_meta(config)
|
||||
|
||||
if load_to_meta:
|
||||
# Construct model with fake meta tensors, later will be replaced on devices during ds-inference ckpt load
|
||||
with deepspeed.OnDevice(dtype=dtype, device="meta"):
|
||||
model = AutoModelForCausalLM.from_config(config, torch_dtype=dtype)
|
||||
else:
|
||||
get_repo_root(model_id, local_rank=os.getenv("LOCAL_RANK"))
|
||||
# TODO: revisit placement on CPU when auto-injection is possible
|
||||
with deepspeed.OnDevice(dtype=dtype, device="cpu"):
|
||||
model = AutoModelForCausalLM.from_pretrained(model_id, torch_dtype=dtype, **model_kwargs)
|
||||
model = model.eval()
|
||||
|
||||
# Initialize the model
|
||||
ds_inference_kwargs = {"dtype": dtype}
|
||||
ds_inference_kwargs["tensor_parallel"] = {"tp_size": world_size}
|
||||
ds_inference_kwargs["enable_cuda_graph"] = self.enable_hpu_graph
|
||||
|
||||
if load_to_meta:
|
||||
# model loaded to meta is managed differently
|
||||
checkpoints_json = tempfile.NamedTemporaryFile(suffix=".json", mode="+w")
|
||||
write_checkpoints_json(model_id, local_rank, checkpoints_json)
|
||||
ds_inference_kwargs["checkpoint"] = checkpoints_json.name
|
||||
model = deepspeed.init_inference(model, **ds_inference_kwargs)
|
||||
model = model.module
|
||||
else:
|
||||
get_repo_root(model_id)
|
||||
model = AutoModelForCausalLM.from_pretrained(
|
||||
model_id,
|
||||
revision=revision,
|
||||
torch_dtype=dtype,
|
||||
)
|
||||
model = model.eval().to(device)
|
||||
#wrap in hpu_graph only if self.enable_hpu_graph is set
|
||||
if self.enable_hpu_graph:
|
||||
model = wrap_in_hpu_graph(model)
|
||||
|
||||
if model.config.model_type in MODELS_OPTIMIZED_WITH_STATIC_SHAPES:
|
||||
self.is_optimized_for_gaudi = True
|
||||
else:
|
||||
self.is_optimized_for_gaudi = False
|
||||
|
||||
if tokenizer.pad_token_id is None:
|
||||
if model.config.pad_token_id is not None:
|
||||
@ -524,64 +646,132 @@ class CausalLM(Model):
|
||||
else:
|
||||
tokenizer.add_special_tokens({"pad_token": "[PAD]"})
|
||||
|
||||
kwargs = {
|
||||
"use_cache": True,
|
||||
"return_dict": True,
|
||||
}
|
||||
|
||||
if model.config.model_type == "llama":
|
||||
kwargs["attn_softmax_bf16"] = True
|
||||
kwargs["trim_logits"] = True
|
||||
|
||||
super(CausalLM, self).__init__(
|
||||
model=model,
|
||||
tokenizer=tokenizer,
|
||||
requires_padding=True,
|
||||
dtype=dtype,
|
||||
device=device,
|
||||
rank=rank,
|
||||
kwargs=kwargs,
|
||||
)
|
||||
self.profiling_warmup_steps = int(os.getenv("PROF_WARMUPSTEP", "0"))
|
||||
self.profiling_steps = int(os.getenv("PROF_STEP", "5"))
|
||||
output_dir = os.getenv("PROF_PATH", "/tmp/hpu_profile")
|
||||
self.hb_profer = HabanaProfile(
|
||||
warmup=self.profiling_warmup_steps, active=self.profiling_steps, output_dir=output_dir
|
||||
)
|
||||
if self.profiling_warmup_steps > 0:
|
||||
self.hb_profer_started = True
|
||||
self.hb_profer.start()
|
||||
else:
|
||||
self.hb_profer = None
|
||||
self.hb_profer_started = False
|
||||
self.step = 0
|
||||
|
||||
@property
|
||||
def batch_type(self) -> Type[CausalLMBatch]:
|
||||
return CausalLMBatch
|
||||
|
||||
def decode(self, generated_ids: List[int]) -> str:
|
||||
return self.tokenizer.decode(
|
||||
generated_ids, skip_special_tokens=True, clean_up_tokenization_spaces=False
|
||||
)
|
||||
return self.tokenizer.decode(generated_ids, skip_special_tokens=True, clean_up_tokenization_spaces=False)
|
||||
|
||||
def forward(
|
||||
self, input_ids, attention_mask, position_ids, past_key_values: Optional = None
|
||||
self,
|
||||
input_ids,
|
||||
attention_mask,
|
||||
position_ids,
|
||||
token_idx: Optional = None,
|
||||
past_key_values: Optional = None,
|
||||
bypass_hpu_graph: Optional = None,
|
||||
) -> Tuple[torch.Tensor, List[Tuple[torch.Tensor, torch.Tensor]]]:
|
||||
# Model Forward
|
||||
kwargs = {
|
||||
"input_ids": input_ids,
|
||||
"attention_mask": attention_mask,
|
||||
"past_key_values": past_key_values,
|
||||
"use_cache": True,
|
||||
"return_dict": True,
|
||||
}
|
||||
|
||||
if self.is_optimized_for_gaudi:
|
||||
kwargs["token_idx"] = token_idx
|
||||
|
||||
if self.has_position_ids:
|
||||
kwargs["position_ids"] = position_ids
|
||||
|
||||
if bypass_hpu_graph != None:
|
||||
kwargs["bypass_hpu_graphs"] = bypass_hpu_graph
|
||||
|
||||
kwargs.update(self.kwargs)
|
||||
outputs = self.model.forward(**kwargs)
|
||||
return outputs.logits, outputs.past_key_values
|
||||
|
||||
@tracer.start_as_current_span("generate_token")
|
||||
def generate_token(
|
||||
self, batch: CausalLMBatch
|
||||
) -> Tuple[List[Generation], Optional[CausalLMBatch]]:
|
||||
# slice the attention mask to the correct shape
|
||||
attention_mask = batch.attention_mask[:, : -batch.padding_right_offset]
|
||||
def generate_token(self, batch: CausalLMBatch) -> Tuple[List[Generation], Optional[CausalLMBatch]]:
|
||||
self.step = self.step + 1
|
||||
if self.hb_profer_started == True and self.step > self.profiling_warmup_steps + self.profiling_steps:
|
||||
self.hb_profer.stop()
|
||||
self.hb_profer_started = False
|
||||
|
||||
if self.is_optimized_for_gaudi:
|
||||
token_idx = torch.tensor(batch.attention_mask.shape[-1] - batch.padding_right_offset).to(self.device)
|
||||
attention_mask = batch.attention_mask
|
||||
|
||||
else:
|
||||
token_idx = None
|
||||
# slice the attention mask to the correct shape
|
||||
attention_mask = batch.attention_mask[:, : -batch.padding_right_offset]
|
||||
prefill = batch.past_key_values is None
|
||||
if batch.past_key_values:
|
||||
if token_idx is not None:
|
||||
input_ids = torch.index_select(batch.input_ids, 1, token_idx - 1)
|
||||
else:
|
||||
input_ids = batch.input_ids
|
||||
|
||||
logits, past = self.forward(
|
||||
batch.input_ids,
|
||||
input_ids,
|
||||
attention_mask,
|
||||
batch.position_ids,
|
||||
token_idx,
|
||||
batch.past_key_values,
|
||||
bypass_hpu_graph = prefill and self.limit_hpu_graph if self.enable_hpu_graph else None
|
||||
)
|
||||
|
||||
# Results
|
||||
generations: List[Generation] = []
|
||||
stopped = True
|
||||
|
||||
# Select next token
|
||||
input_length = batch.input_lengths[0]
|
||||
if self.is_optimized_for_gaudi and logits.shape[-2] > 1:
|
||||
next_input_ids, next_token_logprobs, logprobs = batch.next_token_chooser(
|
||||
batch.input_ids[:, :token_idx], logits[:, input_length - 1 : input_length, :].squeeze(-2)
|
||||
)
|
||||
else:
|
||||
next_input_ids, next_token_logprobs, logprobs = batch.next_token_chooser(
|
||||
batch.input_ids[:, :token_idx], logits.squeeze(-2)
|
||||
)
|
||||
|
||||
batch_top_token_ids, batch_top_token_logprobs = batch_top_tokens(
|
||||
batch.top_n_tokens,
|
||||
batch.top_n_tokens_tensor,
|
||||
torch.log_softmax(logits[:, -1], -1),
|
||||
logprobs,
|
||||
)
|
||||
|
||||
htorch.core.mark_step()
|
||||
logits = logits.to("cpu")
|
||||
|
||||
next_token_logprobs = next_token_logprobs.tolist()
|
||||
next_token_ids = next_input_ids
|
||||
|
||||
# Zipped iterator
|
||||
iterator = zip(
|
||||
batch.requests,
|
||||
@ -589,14 +779,16 @@ class CausalLM(Model):
|
||||
batch.prefix_offsets,
|
||||
batch.read_offsets,
|
||||
logits,
|
||||
batch.next_token_choosers,
|
||||
batch.next_token_chooser.do_sample,
|
||||
batch.next_token_chooser.seeds,
|
||||
batch.stopping_criterias,
|
||||
batch.all_input_ids,
|
||||
batch.top_n_tokens,
|
||||
next_token_ids,
|
||||
next_token_logprobs,
|
||||
batch_top_token_ids,
|
||||
batch_top_token_logprobs,
|
||||
)
|
||||
|
||||
# For each member of the batch
|
||||
for i, (
|
||||
request,
|
||||
@ -604,32 +796,31 @@ class CausalLM(Model):
|
||||
prefix_offset,
|
||||
read_offset,
|
||||
logits,
|
||||
next_token_chooser,
|
||||
do_sample,
|
||||
seed,
|
||||
stopping_criteria,
|
||||
all_input_ids,
|
||||
top_n_tokens,
|
||||
next_token_id,
|
||||
next_token_logprob,
|
||||
top_token_ids,
|
||||
top_token_logprobs,
|
||||
) in enumerate(iterator):
|
||||
# Select next token
|
||||
next_token_id, logprobs = next_token_chooser(
|
||||
all_input_ids.view(1, -1), logits[-1:, :]
|
||||
)
|
||||
|
||||
# Append next token to all tokens
|
||||
all_input_ids = torch.cat([all_input_ids, next_token_id])
|
||||
if self.is_optimized_for_gaudi:
|
||||
all_input_ids[input_length] = next_token_id
|
||||
else:
|
||||
all_input_ids = torch.cat([all_input_ids, next_token_id])
|
||||
new_input_length = input_length + 1
|
||||
|
||||
# Generated token
|
||||
next_token_logprob = logprobs[-1, next_token_id]
|
||||
next_token_id_squeezed = next_token_id.squeeze()
|
||||
next_token_text, prefix_offset, read_offset = self.decode_token(
|
||||
all_input_ids[:, 0], prefix_offset, read_offset
|
||||
all_input_ids[0:new_input_length, 0], prefix_offset, read_offset
|
||||
)
|
||||
|
||||
# Evaluate stopping criteria
|
||||
stop, reason = stopping_criteria(
|
||||
next_token_id_squeezed,
|
||||
next_token_id,
|
||||
next_token_text,
|
||||
)
|
||||
|
||||
@ -641,23 +832,14 @@ class CausalLM(Model):
|
||||
if i % self.world_size == self.rank:
|
||||
if stop:
|
||||
# Decode generated tokens
|
||||
output_text, _, _ = self.decode_token(
|
||||
all_input_ids[:, 0],
|
||||
prefix_offset=len(all_input_ids)
|
||||
- stopping_criteria.current_tokens
|
||||
- 1,
|
||||
read_offset=len(all_input_ids)
|
||||
- stopping_criteria.current_tokens,
|
||||
skip_special_tokens=True,
|
||||
output_text = self.decode(
|
||||
all_input_ids[new_input_length - stopping_criteria.current_tokens : new_input_length, 0]
|
||||
)
|
||||
# Get seed
|
||||
if isinstance(next_token_chooser.choice, Sampling):
|
||||
seed = next_token_chooser.choice.seed
|
||||
else:
|
||||
seed = None
|
||||
|
||||
generated_text = GeneratedText(
|
||||
output_text, stopping_criteria.current_tokens, reason, seed
|
||||
output_text,
|
||||
stopping_criteria.current_tokens,
|
||||
reason,
|
||||
seed if do_sample else None,
|
||||
)
|
||||
else:
|
||||
generated_text = None
|
||||
@ -665,20 +847,14 @@ class CausalLM(Model):
|
||||
# Prefill
|
||||
if stopping_criteria.current_tokens == 1 and request.prefill_logprobs:
|
||||
# Remove generated token to only have prefill and add nan for first prompt token
|
||||
prefill_logprobs = [float("nan")] + torch.log_softmax(
|
||||
logits, -1
|
||||
).gather(1, all_input_ids[1:]).squeeze(1)[
|
||||
-new_input_length:-1
|
||||
].tolist()
|
||||
prefill_token_ids = all_input_ids[-new_input_length:-1]
|
||||
prefill_logprobs = [float("nan")] + next_token_logprobs
|
||||
prefill_token_ids = all_input_ids[0 : new_input_length - 1]
|
||||
prefill_texts = self.tokenizer.batch_decode(
|
||||
prefill_token_ids,
|
||||
clean_up_tokenization_spaces=False,
|
||||
skip_special_tokens=False,
|
||||
)
|
||||
prefill_tokens = PrefillTokens(
|
||||
prefill_token_ids, prefill_logprobs, prefill_texts
|
||||
)
|
||||
prefill_tokens = PrefillTokens(prefill_token_ids, prefill_logprobs, prefill_texts)
|
||||
else:
|
||||
prefill_tokens = None
|
||||
|
||||
@ -688,9 +864,7 @@ class CausalLM(Model):
|
||||
clean_up_tokenization_spaces=False,
|
||||
skip_special_tokens=False,
|
||||
)
|
||||
special_toptokens = [
|
||||
token_id in self.all_special_ids for token_id in top_token_ids
|
||||
]
|
||||
special_toptokens = [token_id in self.all_special_ids for token_id in top_token_ids]
|
||||
top_tokens = TopTokens(
|
||||
top_token_ids,
|
||||
top_token_logprobs,
|
||||
@ -703,40 +877,53 @@ class CausalLM(Model):
|
||||
generation = Generation(
|
||||
request.id,
|
||||
prefill_tokens,
|
||||
next_token_id_squeezed,
|
||||
next_token_id,
|
||||
next_token_logprob,
|
||||
next_token_text,
|
||||
next_token_id_squeezed.item() in self.all_special_ids,
|
||||
next_token_id in self.all_special_ids,
|
||||
generated_text,
|
||||
top_tokens,
|
||||
)
|
||||
|
||||
generations.append(generation)
|
||||
|
||||
# Update values
|
||||
batch.input_ids[i, 0] = next_token_id
|
||||
batch.all_input_ids[i] = all_input_ids
|
||||
batch.input_lengths[i] = new_input_length
|
||||
batch.prefix_offsets[i] = prefix_offset
|
||||
batch.read_offsets[i] = read_offset
|
||||
batch.max_input_length = max(batch.max_input_length, new_input_length)
|
||||
|
||||
next_tokens = torch.tensor(next_token_ids, dtype=torch.int64).to(self.device)
|
||||
if token_idx is None:
|
||||
batch.input_ids[:, 0] = next_tokens[:, 0]
|
||||
else:
|
||||
batch.input_ids[:, token_idx] = next_tokens
|
||||
# We finished all generations in the batch; there is no next batch
|
||||
if stopped:
|
||||
if self.hb_profer_started == True:
|
||||
self.hb_profer.step()
|
||||
return generations, None
|
||||
|
||||
# Slice unused values from prefill
|
||||
batch.input_ids = batch.input_ids[:, :1]
|
||||
# Slice unused values from prefill, use it to store next token
|
||||
if token_idx is None:
|
||||
batch.input_ids = batch.input_ids[:, :1]
|
||||
|
||||
# Update attention_mask as we added a new token to input_ids
|
||||
batch.attention_mask[:, -batch.padding_right_offset] = 1
|
||||
if self.is_optimized_for_gaudi:
|
||||
batch.attention_mask.index_fill_(1, token_idx, 1)
|
||||
else:
|
||||
batch.attention_mask[:, -batch.padding_right_offset] = 1
|
||||
# Decrease right offset
|
||||
batch.padding_right_offset -= 1
|
||||
|
||||
# Update position_ids
|
||||
batch.position_ids = batch.position_ids[:, -1:] + 1
|
||||
|
||||
if prefill:
|
||||
batch.position_ids = batch.position_ids[:, token_idx - 1 : token_idx] + 1
|
||||
else:
|
||||
batch.position_ids += 1
|
||||
# Update past key values
|
||||
batch.past_key_values = past
|
||||
if self.hb_profer_started == True:
|
||||
self.hb_profer.step()
|
||||
|
||||
return generations, batch
|
||||
|
@ -2,10 +2,10 @@ import inspect
|
||||
import torch
|
||||
|
||||
from abc import ABC, abstractmethod
|
||||
from typing import List, Tuple, Optional, TypeVar, Type
|
||||
from transformers import PreTrainedTokenizerBase, PretrainedConfig
|
||||
from typing import List, Optional, Tuple, Type, TypeVar
|
||||
from transformers import PreTrainedTokenizerBase
|
||||
|
||||
from text_generation_server.models.types import Batch, Generation
|
||||
from text_generation_server.models.types import Batch, GeneratedText
|
||||
from text_generation_server.pb.generate_pb2 import InfoResponse
|
||||
|
||||
B = TypeVar("B", bound=Batch)
|
||||
@ -21,9 +21,9 @@ class Model(ABC):
|
||||
device: torch.device,
|
||||
rank: int = 0,
|
||||
world_size: int = 1,
|
||||
sliding_window: Optional[int] = None,
|
||||
kwargs: dict = {},
|
||||
):
|
||||
self.model = model.eval()
|
||||
self.model = model
|
||||
self.tokenizer = tokenizer
|
||||
self.all_special_ids = set(tokenizer.all_special_ids)
|
||||
self.requires_padding = requires_padding
|
||||
@ -31,25 +31,17 @@ class Model(ABC):
|
||||
self.device = device
|
||||
self.rank = rank
|
||||
self.world_size = world_size
|
||||
self.sliding_window = sliding_window
|
||||
|
||||
self.has_position_ids = (
|
||||
inspect.signature(model.forward).parameters.get("position_ids", None)
|
||||
is not None
|
||||
)
|
||||
self.kwargs = kwargs
|
||||
self.has_position_ids = inspect.signature(model.forward).parameters.get("position_ids", None) is not None
|
||||
|
||||
self.check_initialized()
|
||||
|
||||
@property
|
||||
def info(self) -> InfoResponse:
|
||||
if self.requires_padding and self.sliding_window is not None:
|
||||
raise NotImplementedError("sliding_window is not implemented with padding")
|
||||
|
||||
return InfoResponse(
|
||||
requires_padding=self.requires_padding,
|
||||
dtype=str(self.dtype),
|
||||
device_type=self.device.type,
|
||||
window_size=self.sliding_window,
|
||||
)
|
||||
|
||||
@property
|
||||
@ -58,31 +50,24 @@ class Model(ABC):
|
||||
raise NotImplementedError
|
||||
|
||||
@abstractmethod
|
||||
def generate_token(self, batch: B) -> Tuple[List[Generation], Optional[B]]:
|
||||
def generate_token(self, batch: B) -> Tuple[List[GeneratedText], Optional[B]]:
|
||||
raise NotImplementedError
|
||||
|
||||
def warmup(self, batch: B) -> Optional[int]:
|
||||
def warmup(self, batch: B, max_total_tokens: int):
|
||||
self.generate_token(batch)
|
||||
return None
|
||||
|
||||
def decode_token(
|
||||
self,
|
||||
all_input_ids: List[int],
|
||||
prefix_offset: int = 0,
|
||||
read_offset: int = 0,
|
||||
skip_special_tokens: bool = False,
|
||||
) -> Tuple[str, int, int]:
|
||||
"""Hack to hopefully support generate_stream for the maximum number of tokenizers"""
|
||||
|
||||
# The prefix text is necessary only to defeat cleanup algorithms in the decode
|
||||
# which decide to add a space or not depending on the surrounding ids.
|
||||
prefix_text = self.tokenizer.decode(
|
||||
all_input_ids[prefix_offset:read_offset],
|
||||
skip_special_tokens=skip_special_tokens,
|
||||
)
|
||||
new_text = self.tokenizer.decode(
|
||||
all_input_ids[prefix_offset:], skip_special_tokens=skip_special_tokens
|
||||
)
|
||||
prefix_text = self.tokenizer.decode(all_input_ids[prefix_offset:read_offset], skip_special_tokens=False)
|
||||
new_text = self.tokenizer.decode(all_input_ids[prefix_offset:], skip_special_tokens=False)
|
||||
|
||||
if len(new_text) > len(prefix_text) and not new_text.endswith("<EFBFBD>"):
|
||||
# utf-8 char at the end means it's a potential unfinished byte sequence
|
||||
|
@ -1,8 +1,5 @@
|
||||
import torch
|
||||
import torch.distributed
|
||||
|
||||
from typing import Optional, List
|
||||
from transformers import AutoTokenizer, AutoModelForCausalLM
|
||||
import torch
|
||||
|
||||
from text_generation_server.models import CausalLM
|
||||
|
||||
@ -18,28 +15,11 @@ class SantaCoder(CausalLM):
|
||||
self,
|
||||
model_id: str,
|
||||
revision: Optional[str] = None,
|
||||
quantize: Optional[str] = None,
|
||||
dtype: Optional[torch.dtype] = None,
|
||||
trust_remote_code: bool = False,
|
||||
):
|
||||
if torch.cuda.is_available():
|
||||
device = torch.device("cuda")
|
||||
dtype = torch.float16 if dtype is None else dtype
|
||||
else:
|
||||
if quantize:
|
||||
raise ValueError("quantization is not available on CPU")
|
||||
super().__init__(model_id=model_id, revision=revision, dtype=dtype)
|
||||
|
||||
device = torch.device("cpu")
|
||||
dtype = torch.float32 if dtype is None else dtype
|
||||
|
||||
tokenizer = AutoTokenizer.from_pretrained(
|
||||
model_id,
|
||||
revision=revision,
|
||||
padding_side="left",
|
||||
truncation_side="left",
|
||||
trust_remote_code=trust_remote_code,
|
||||
)
|
||||
tokenizer.add_special_tokens(
|
||||
self.tokenizer.add_special_tokens(
|
||||
{
|
||||
"additional_special_tokens": [
|
||||
EOD,
|
||||
@ -51,25 +31,7 @@ class SantaCoder(CausalLM):
|
||||
"pad_token": EOD,
|
||||
}
|
||||
)
|
||||
with device:
|
||||
model = AutoModelForCausalLM.from_pretrained(
|
||||
model_id,
|
||||
revision=revision,
|
||||
torch_dtype=dtype,
|
||||
load_in_8bit=quantize == "bitsandbytes",
|
||||
trust_remote_code=trust_remote_code,
|
||||
)
|
||||
|
||||
super(CausalLM, self).__init__(
|
||||
model=model,
|
||||
tokenizer=tokenizer,
|
||||
requires_padding=True,
|
||||
dtype=dtype,
|
||||
device=device,
|
||||
)
|
||||
|
||||
def decode(self, generated_ids: List[int]) -> str:
|
||||
# Do not skip special tokens as they are used for custom parsing rules of the generated text
|
||||
return self.tokenizer.decode(
|
||||
generated_ids, skip_special_tokens=False, clean_up_tokenization_spaces=False
|
||||
)
|
||||
return self.tokenizer.decode(generated_ids, skip_special_tokens=False, clean_up_tokenization_spaces=False)
|
||||
|
@ -1,5 +1,6 @@
|
||||
import asyncio
|
||||
import os
|
||||
import sys
|
||||
import torch
|
||||
|
||||
from grpc import aio
|
||||
@ -14,7 +15,6 @@ from text_generation_server.interceptor import ExceptionInterceptor
|
||||
from text_generation_server.models import Model, get_model
|
||||
from text_generation_server.pb import generate_pb2_grpc, generate_pb2
|
||||
from text_generation_server.tracing import UDSOpenTelemetryAioServerInterceptor
|
||||
from text_generation_server.models.idefics_causal_lm import IdeficsCausalLMBatch
|
||||
|
||||
|
||||
class TextGenerationService(generate_pb2_grpc.TextGenerationServiceServicer):
|
||||
@ -23,16 +23,18 @@ class TextGenerationService(generate_pb2_grpc.TextGenerationServiceServicer):
|
||||
self.model = model
|
||||
self.server_urls = server_urls
|
||||
# For some reason, inference_mode does not work well with GLOO which we use on CPU
|
||||
if model.device.type == "cuda":
|
||||
# Force inference mode for the lifetime of TextGenerationService
|
||||
self._inference_mode_raii_guard = torch._C._InferenceMode(True)
|
||||
# TODO: The inferecemode set messes up the autograd op dispatch. And results in aten::matmul
|
||||
# op not optimized issue. Will investigate further.
|
||||
# if model.device.type == "hpu":
|
||||
# Force inference mode for the lifetime of TextGenerationService
|
||||
# self._inference_mode_raii_guard = torch._C._InferenceMode(True)
|
||||
|
||||
async def Info(self, request, context):
|
||||
return self.model.info
|
||||
|
||||
async def Health(self, request, context):
|
||||
if self.model.device.type == "cuda":
|
||||
torch.zeros((2, 2)).cuda()
|
||||
if self.model.device.type == "hpu":
|
||||
torch.zeros((2, 2)).to("hpu")
|
||||
return generate_pb2.HealthResponse()
|
||||
|
||||
async def ServiceDiscovery(self, request, context):
|
||||
@ -49,47 +51,27 @@ class TextGenerationService(generate_pb2_grpc.TextGenerationServiceServicer):
|
||||
batch = self.cache.pop(request.batch_id)
|
||||
if batch is None:
|
||||
raise ValueError(f"Batch ID {request.batch_id} not found in cache.")
|
||||
filtered_batch = batch.filter(request.request_ids)
|
||||
filtered_batch = batch.filter(request.request_ids, self.model.is_optimized_for_gaudi)
|
||||
self.cache.set(filtered_batch)
|
||||
|
||||
return generate_pb2.FilterBatchResponse(batch=filtered_batch.to_pb())
|
||||
|
||||
async def Warmup(self, request, context):
|
||||
if (
|
||||
self.model.batch_type == IdeficsCausalLMBatch
|
||||
): # Hack, i would rather use kwargs in the `from_pb` call
|
||||
batch = self.model.batch_type.from_pb(
|
||||
request.batch,
|
||||
self.model.tokenizer,
|
||||
self.model.processor,
|
||||
self.model.dtype,
|
||||
self.model.device,
|
||||
)
|
||||
else:
|
||||
batch = self.model.batch_type.from_pb(
|
||||
request.batch, self.model.tokenizer, self.model.dtype, self.model.device
|
||||
)
|
||||
max_supported_total_tokens = self.model.warmup(batch)
|
||||
# batch = self.model.batch_type.from_pb(
|
||||
# request.batch, self.model.tokenizer, self.model.dtype, self.model.device
|
||||
# )
|
||||
# max_supported_total_tokens = self.model.warmup(batch)
|
||||
|
||||
return generate_pb2.WarmupResponse(
|
||||
max_supported_total_tokens=max_supported_total_tokens
|
||||
)
|
||||
# return generate_pb2.WarmupResponse(
|
||||
# max_supported_total_tokens=max_supported_total_tokens
|
||||
# )
|
||||
logger.warning("Warmup is not enabled on HPU.")
|
||||
return generate_pb2.WarmupResponse()
|
||||
|
||||
async def Prefill(self, request, context):
|
||||
if (
|
||||
self.model.batch_type == IdeficsCausalLMBatch
|
||||
): # Hack, i would rather use kwargs in the `from_pb` call
|
||||
batch = self.model.batch_type.from_pb(
|
||||
request.batch,
|
||||
self.model.tokenizer,
|
||||
self.model.processor,
|
||||
self.model.dtype,
|
||||
self.model.device,
|
||||
)
|
||||
else:
|
||||
batch = self.model.batch_type.from_pb(
|
||||
request.batch, self.model.tokenizer, self.model.dtype, self.model.device
|
||||
)
|
||||
batch = self.model.batch_type.from_pb(
|
||||
request.batch, self.model.tokenizer, self.model.dtype, self.model.device, self.model.is_optimized_for_gaudi
|
||||
)
|
||||
|
||||
generations, next_batch = self.model.generate_token(batch)
|
||||
self.cache.set(next_batch)
|
||||
@ -114,7 +96,7 @@ class TextGenerationService(generate_pb2_grpc.TextGenerationServiceServicer):
|
||||
raise ValueError("All batches are empty")
|
||||
|
||||
if len(batches) > 1:
|
||||
batch = self.model.batch_type.concatenate(batches)
|
||||
batch = self.model.batch_type.concatenate(batches, self.model.is_optimized_for_gaudi)
|
||||
else:
|
||||
batch = batches[0]
|
||||
|
||||
@ -130,54 +112,53 @@ class TextGenerationService(generate_pb2_grpc.TextGenerationServiceServicer):
|
||||
def serve(
|
||||
model_id: str,
|
||||
revision: Optional[str],
|
||||
sharded: bool,
|
||||
quantize: Optional[str],
|
||||
dtype: Optional[str],
|
||||
trust_remote_code: bool,
|
||||
uds_path: Path,
|
||||
sharded: bool,
|
||||
):
|
||||
# Remove default handler
|
||||
logger.remove()
|
||||
logger.add(
|
||||
sys.stdout,
|
||||
format="{message}",
|
||||
filter="text_generation_server",
|
||||
level="INFO",
|
||||
serialize=False,
|
||||
backtrace=True,
|
||||
diagnose=False,
|
||||
)
|
||||
|
||||
async def serve_inner(
|
||||
model_id: str,
|
||||
revision: Optional[str],
|
||||
sharded: bool = False,
|
||||
quantize: Optional[str] = None,
|
||||
dtype: Optional[str] = None,
|
||||
trust_remote_code: bool = False,
|
||||
sharded: bool = False,
|
||||
):
|
||||
unix_socket_template = "unix://{}-{}"
|
||||
logger.info("Server:server_inner: sharded ={}".format(sharded))
|
||||
|
||||
if sharded:
|
||||
rank = int(os.environ["RANK"])
|
||||
logger.info("Server:server_inner: rank ={}".format(rank))
|
||||
server_urls = [
|
||||
unix_socket_template.format(uds_path, rank)
|
||||
for rank in range(int(os.environ["WORLD_SIZE"]))
|
||||
unix_socket_template.format(uds_path, rank) for rank in range(int(os.environ["WORLD_SIZE"]))
|
||||
]
|
||||
local_url = server_urls[int(os.environ["RANK"])]
|
||||
else:
|
||||
local_url = unix_socket_template.format(uds_path, 0)
|
||||
server_urls = [local_url]
|
||||
|
||||
logger.info("Server:server_inner: data type = {}, local_url = {}".format(dtype, local_url))
|
||||
if dtype == "bfloat16" or None:
|
||||
data_type = torch.bfloat16
|
||||
else:
|
||||
data_type = torch.float
|
||||
try:
|
||||
model = get_model(
|
||||
model_id, revision, sharded, quantize, dtype, trust_remote_code
|
||||
)
|
||||
model = get_model(model_id, revision=revision, dtype=data_type)
|
||||
except Exception:
|
||||
logger.exception("Error when initializing model")
|
||||
raise
|
||||
|
||||
if quantize == "gptq":
|
||||
try:
|
||||
# When using GPTQ, Exllama kernels need some global kernels
|
||||
# For which we have the finale shapes only after the model has loaded
|
||||
# This will allocate those buffers.
|
||||
from text_generation_server.utils.gptq.exllama import (
|
||||
create_exllama_buffers,
|
||||
set_device,
|
||||
)
|
||||
|
||||
set_device(model.device)
|
||||
create_exllama_buffers()
|
||||
except ImportError:
|
||||
pass
|
||||
|
||||
server = aio.server(
|
||||
interceptors=[
|
||||
ExceptionInterceptor(),
|
||||
@ -204,6 +185,9 @@ def serve(
|
||||
logger.info("Signal received. Shutting down")
|
||||
await server.stop(0)
|
||||
|
||||
asyncio.run(
|
||||
serve_inner(model_id, revision, sharded, quantize, dtype, trust_remote_code)
|
||||
logger.info(
|
||||
"Starting Server : model_id= {}, revision = {} dtype = {} sharded = {} ".format(
|
||||
model_id, revision, dtype, sharded
|
||||
)
|
||||
)
|
||||
asyncio.run(serve_inner(model_id, revision, dtype, sharded))
|
||||
|
29
server/text_generation_server/tgi_service.py
Normal file
29
server/text_generation_server/tgi_service.py
Normal file
@ -0,0 +1,29 @@
|
||||
import os
|
||||
from pathlib import Path
|
||||
from loguru import logger
|
||||
import sys
|
||||
from text_generation_server import server
|
||||
import argparse
|
||||
|
||||
|
||||
def main(args):
|
||||
logger.info("TGIService: starting tgi service .... ")
|
||||
logger.info(
|
||||
"TGIService: --model_id {}, --revision {}, --sharded {}, --dtype {}, --uds_path {} ".format(
|
||||
args.model_id, args.revision, args.sharded, args.dtype, args.uds_path
|
||||
)
|
||||
)
|
||||
server.serve(
|
||||
model_id=args.model_id, revision=args.revision, dtype=args.dtype, uds_path=args.uds_path, sharded=args.sharded
|
||||
)
|
||||
|
||||
|
||||
if __name__ == "__main__":
|
||||
parser = argparse.ArgumentParser()
|
||||
parser.add_argument("--model_id", type=str)
|
||||
parser.add_argument("--revision", type=str)
|
||||
parser.add_argument("--sharded", type=bool)
|
||||
parser.add_argument("--dtype", type=str)
|
||||
parser.add_argument("--uds_path", type=Path)
|
||||
args = parser.parse_args()
|
||||
main(args)
|
@ -44,6 +44,12 @@ class FakeGroup:
|
||||
|
||||
|
||||
def initialize_torch_distributed():
|
||||
import habana_frameworks.torch.core as htcore
|
||||
|
||||
rank = int(os.getenv("RANK", "0"))
|
||||
world_size = int(os.getenv("WORLD_SIZE", "1"))
|
||||
|
||||
options = None
|
||||
if torch.cuda.is_available():
|
||||
from torch.distributed import ProcessGroupNCCL
|
||||
|
||||
@ -56,9 +62,13 @@ def initialize_torch_distributed():
|
||||
options = ProcessGroupNCCL.Options()
|
||||
options.is_high_priority_stream = True
|
||||
options._timeout = timedelta(seconds=60)
|
||||
elif torch.hpu.is_available():
|
||||
backend = "hccl"
|
||||
n_hpus = torch.hpu.device_count()
|
||||
if world_size > n_hpus:
|
||||
raise ValueError(f"WORLD_SIZE ({world_size}) is higher than the number of available HPUs ({n_hpus}).")
|
||||
else:
|
||||
backend = "gloo"
|
||||
options = None
|
||||
|
||||
if WORLD_SIZE == 1:
|
||||
return FakeGroup(RANK, WORLD_SIZE), RANK, WORLD_SIZE
|
||||
|
@ -1,5 +1,6 @@
|
||||
import math
|
||||
import torch
|
||||
import habana_frameworks.torch.core as htcore
|
||||
|
||||
from functools import lru_cache
|
||||
from typing import Optional, List, Dict, Union
|
||||
@ -36,37 +37,31 @@ class StaticWarper:
|
||||
if typical_p is not None and typical_p < 1.0:
|
||||
self.warpers.append(TypicalLogitsWarper(mass=typical_p))
|
||||
|
||||
self.cuda_graph = None
|
||||
self.hpu_graph = None
|
||||
self.static_scores = None
|
||||
self.static_warped_scores = None
|
||||
self.static_next_logprob = None
|
||||
|
||||
def __call__(self, scores):
|
||||
if torch.cuda.is_available():
|
||||
if self.cuda_graph is None:
|
||||
self.static_scores = scores
|
||||
self.cuda_graph = torch.cuda.CUDAGraph()
|
||||
if self.hpu_graph is None:
|
||||
self.static_scores = scores.clone().contiguous()
|
||||
self.static_warped_scores = scores.clone().contiguous()
|
||||
self.static_next_logprob = scores.clone().contiguous()
|
||||
self.hpu_graph = htcore.hpu.HPUGraph()
|
||||
|
||||
with torch.cuda.graph(self.cuda_graph, pool=mempool):
|
||||
local_scores = self.static_scores
|
||||
for warper in self.warpers:
|
||||
local_scores = warper(None, local_scores)
|
||||
with htcore.hpu.graph(self.hpu_graph):
|
||||
local_scores = self.static_scores
|
||||
for warper in self.warpers:
|
||||
local_scores = warper(None, local_scores)
|
||||
|
||||
self.static_warped_scores = local_scores
|
||||
# Compute logprobs
|
||||
self.static_next_logprob = torch.log_softmax(
|
||||
self.static_warped_scores, -1
|
||||
)
|
||||
self.static_warped_scores.copy_(local_scores)
|
||||
# Compute logprobs
|
||||
self.static_next_logprob.copy_(torch.log_softmax(self.static_warped_scores, -1))
|
||||
|
||||
self.static_scores.copy_(scores)
|
||||
self.cuda_graph.replay()
|
||||
self.static_scores.copy_(scores)
|
||||
self.hpu_graph.replay()
|
||||
|
||||
return self.static_warped_scores, self.static_next_logprob
|
||||
|
||||
# CPU branch
|
||||
for warper in self.warpers:
|
||||
scores = warper(None, scores)
|
||||
return scores, torch.log_softmax(scores, -1)
|
||||
return self.static_warped_scores, self.static_next_logprob
|
||||
|
||||
|
||||
@lru_cache(10)
|
||||
@ -76,9 +71,7 @@ def static_warper(
|
||||
top_p: Optional[float],
|
||||
typical_p: Optional[float],
|
||||
) -> StaticWarper:
|
||||
return StaticWarper(
|
||||
temperature=temperature, top_k=top_k, top_p=top_p, typical_p=typical_p
|
||||
)
|
||||
return StaticWarper(temperature=temperature, top_k=top_k, top_p=top_p, typical_p=typical_p)
|
||||
|
||||
|
||||
class HeterogeneousRepetitionPenaltyLogitsProcessor(LogitsProcessor):
|
||||
@ -95,17 +88,13 @@ class HeterogeneousRepetitionPenaltyLogitsProcessor(LogitsProcessor):
|
||||
|
||||
def __init__(self, penalty: List[float], dtype: torch.dtype, device: torch.device):
|
||||
self.penalty = penalty
|
||||
self.penalty_tensor = torch.tensor(
|
||||
penalty, dtype=dtype, device=device
|
||||
).unsqueeze(1)
|
||||
self.penalty_tensor = torch.tensor(penalty, dtype=dtype, device=device).unsqueeze(1)
|
||||
|
||||
def __call__(self, input_ids: torch.Tensor, scores: torch.Tensor) -> torch.Tensor:
|
||||
score = torch.gather(scores, 1, input_ids)
|
||||
|
||||
# if score < 0 then repetition penalty has to be multiplied to reduce the previous token probability
|
||||
score = torch.where(
|
||||
score < 0, score * self.penalty_tensor, score / self.penalty_tensor
|
||||
)
|
||||
score = torch.where(score < 0, score * self.penalty_tensor, score / self.penalty_tensor)
|
||||
|
||||
scores.scatter_(1, input_ids, score)
|
||||
return scores
|
||||
@ -129,13 +118,9 @@ class HeterogeneousTemperatureLogitsWarper:
|
||||
The value used to module the logits distribution.
|
||||
"""
|
||||
|
||||
def __init__(
|
||||
self, temperature: List[float], dtype: torch.dtype, device: torch.device
|
||||
):
|
||||
def __init__(self, temperature: List[float], dtype: torch.dtype, device: torch.device):
|
||||
self.temperature = temperature
|
||||
self.temperature_tensor = torch.tensor(
|
||||
temperature, dtype=dtype, device=device
|
||||
).unsqueeze(1)
|
||||
self.temperature_tensor = torch.tensor(temperature, dtype=dtype, device=device).unsqueeze(1)
|
||||
|
||||
def __call__(self, input_ids: torch.Tensor, scores: torch.Tensor) -> torch.Tensor:
|
||||
scores.div_(self.temperature_tensor)
|
||||
@ -174,9 +159,7 @@ class HeterogeneousTopPLogitsWarper(LogitsWarper):
|
||||
min_tokens_to_keep: int = 1,
|
||||
):
|
||||
self.top_p = top_p
|
||||
self.top_p_opposite = 1 - torch.tensor(
|
||||
top_p, dtype=dtype, device=device
|
||||
).unsqueeze(1)
|
||||
self.top_p_opposite = 1 - torch.tensor(top_p, dtype=dtype, device=device).unsqueeze(1)
|
||||
self.filter_value = filter_value
|
||||
self.min_tokens_to_keep = min_tokens_to_keep
|
||||
|
||||
@ -193,9 +176,7 @@ class HeterogeneousTopPLogitsWarper(LogitsWarper):
|
||||
sorted_indices_to_remove[..., -self.min_tokens_to_keep :] = 0
|
||||
|
||||
# scatter sorted tensors to original indexing
|
||||
indices_to_remove = sorted_indices_to_remove.scatter(
|
||||
1, sorted_indices, sorted_indices_to_remove
|
||||
)
|
||||
indices_to_remove = sorted_indices_to_remove.scatter(1, sorted_indices, sorted_indices_to_remove)
|
||||
warped_scores = scores.masked_fill_(indices_to_remove, self.filter_value)
|
||||
|
||||
return warped_scores
|
||||
@ -243,9 +224,7 @@ class HeterogeneousTopKLogitsWarper(LogitsWarper):
|
||||
disabled = [x == 0 for x in top_k]
|
||||
|
||||
if any(disabled):
|
||||
self.top_k_disabled_mask = torch.tensor(
|
||||
disabled, dtype=torch.bool, device=device
|
||||
).view(-1, 1)
|
||||
self.top_k_disabled_mask = torch.tensor(disabled, dtype=torch.bool, device=device).view(-1, 1)
|
||||
else:
|
||||
self.top_k_disabled_mask = None
|
||||
|
||||
@ -281,9 +260,7 @@ class HeterogeneousTopKLogitsWarper(LogitsWarper):
|
||||
self.max_top_k = max(self.top_k)
|
||||
|
||||
if self.top_k_disabled_mask is not None:
|
||||
self.top_k_disabled_mask = (
|
||||
self.top_k_disabled_mask[indices] if any(disabled) else None
|
||||
)
|
||||
self.top_k_disabled_mask = self.top_k_disabled_mask[indices] if any(disabled) else None
|
||||
|
||||
return self
|
||||
return None
|
||||
@ -349,15 +326,11 @@ class HeterogeneousTypicalLogitsWarper(LogitsWarper):
|
||||
if self.disabled_mask is not None:
|
||||
last_ind.masked_fill_(self.disabled_mask, scores.shape[-1] - 1)
|
||||
|
||||
sorted_indices_to_remove = sorted_scores > sorted_scores.gather(
|
||||
1, last_ind.view(-1, 1)
|
||||
)
|
||||
sorted_indices_to_remove = sorted_scores > sorted_scores.gather(1, last_ind.view(-1, 1))
|
||||
if self.min_tokens_to_keep > 1:
|
||||
# Keep at least min_tokens_to_keep (set to min_tokens_to_keep-1 because we add the first one below)
|
||||
sorted_indices_to_remove[..., : self.min_tokens_to_keep] = 0
|
||||
indices_to_remove = sorted_indices_to_remove.scatter(
|
||||
1, sorted_indices, sorted_indices_to_remove
|
||||
)
|
||||
indices_to_remove = sorted_indices_to_remove.scatter(1, sorted_indices, sorted_indices_to_remove)
|
||||
|
||||
warped_scores = scores.masked_fill_(indices_to_remove, self.filter_value)
|
||||
|
||||
@ -371,9 +344,7 @@ class HeterogeneousTypicalLogitsWarper(LogitsWarper):
|
||||
self.mass_tensor = self.mass_tensor[indices]
|
||||
|
||||
if self.disabled_mask is not None:
|
||||
self.disabled_mask = (
|
||||
self.disabled_mask[indices] if any(disabled) else None
|
||||
)
|
||||
self.disabled_mask = self.disabled_mask[indices] if any(disabled) else None
|
||||
|
||||
return self
|
||||
return None
|
||||
|
@ -30,13 +30,9 @@ class NextTokenChooser:
|
||||
seed=0,
|
||||
device="cpu",
|
||||
):
|
||||
self.watermark_processor = (
|
||||
WatermarkLogitsProcessor(device=device) if watermark else None
|
||||
)
|
||||
self.watermark_processor = WatermarkLogitsProcessor(device=device) if watermark else None
|
||||
self.repetition_processor = (
|
||||
RepetitionPenaltyLogitsProcessor(penalty=repetition_penalty)
|
||||
if repetition_penalty
|
||||
else None
|
||||
RepetitionPenaltyLogitsProcessor(penalty=repetition_penalty) if repetition_penalty else None
|
||||
)
|
||||
|
||||
has_warpers = (
|
||||
@ -46,9 +42,7 @@ class NextTokenChooser:
|
||||
or (typical_p is not None and typical_p < 1.0)
|
||||
)
|
||||
if has_warpers:
|
||||
self.static_warper = static_warper(
|
||||
temperature=temperature, top_k=top_k, top_p=top_p, typical_p=typical_p
|
||||
)
|
||||
self.static_warper = static_warper(temperature=temperature, top_k=top_k, top_p=top_p, typical_p=typical_p)
|
||||
else:
|
||||
self.static_warper = None
|
||||
|
||||
@ -136,9 +130,7 @@ class StoppingCriteria:
|
||||
pb: generate_pb2.StoppingCriteriaParameters,
|
||||
tokenizer: PreTrainedTokenizerBase,
|
||||
) -> "StoppingCriteria":
|
||||
stop_sequence_criterias = [
|
||||
StopSequenceCriteria(sequence) for sequence in pb.stop_sequences
|
||||
]
|
||||
stop_sequence_criterias = [StopSequenceCriteria(sequence) for sequence in pb.stop_sequences]
|
||||
return StoppingCriteria(
|
||||
tokenizer.eos_token_id,
|
||||
stop_sequence_criterias,
|
||||
@ -176,20 +168,14 @@ class HeterogeneousNextTokenChooser:
|
||||
)
|
||||
|
||||
self.repetition_processor = (
|
||||
HeterogeneousRepetitionPenaltyLogitsProcessor(
|
||||
repetition_penalty, dtype, device
|
||||
)
|
||||
HeterogeneousRepetitionPenaltyLogitsProcessor(repetition_penalty, dtype, device)
|
||||
if any([x != 1.0 for x in repetition_penalty])
|
||||
else None
|
||||
)
|
||||
|
||||
if any([x != 1.0 for x in temperature]):
|
||||
do_sample = [
|
||||
sample or x != 1.0 for x, sample in zip(temperature, do_sample)
|
||||
]
|
||||
warpers.append(
|
||||
HeterogeneousTemperatureLogitsWarper(temperature, dtype, device)
|
||||
)
|
||||
do_sample = [sample or x != 1.0 for x, sample in zip(temperature, do_sample)]
|
||||
warpers.append(HeterogeneousTemperatureLogitsWarper(temperature, dtype, device))
|
||||
|
||||
if any([x != 0 for x in top_k]):
|
||||
do_sample = [sample or x != 0 for x, sample in zip(top_k, do_sample)]
|
||||
@ -277,7 +263,7 @@ class HeterogeneousNextTokenChooser:
|
||||
|
||||
class Sampling:
|
||||
def __init__(self, seed: int, device: str = "cpu"):
|
||||
self.generator = torch.Generator(device)
|
||||
self.generator = torch.Generator("cpu")
|
||||
self.generator.manual_seed(seed)
|
||||
self.seed = seed
|
||||
|
||||
@ -355,30 +341,21 @@ def batch_top_tokens(
|
||||
# Parallel kthvalue adapted from https://discuss.pytorch.org/t/how-to-efficiently-get-the-k-th-largest-values-in-parallel/160529/2
|
||||
# Sorted topk is faster than torch.sort() since we only need a small subset
|
||||
sorted_top_k = torch.topk(logprobs, k=max_top_n, dim=1, sorted=True).values
|
||||
nth_highest = torch.gather(
|
||||
sorted_top_k, 1, (top_n_tokens_tensor - 1).clip(min=0).unsqueeze(1)
|
||||
)
|
||||
nth_highest = torch.gather(sorted_top_k, 1, (top_n_tokens_tensor - 1).clip(min=0).unsqueeze(1))
|
||||
nth_highest[nth_highest == -float("inf")] = torch.finfo(logprobs.dtype).min
|
||||
|
||||
# Find the new "fuzzy" top n values
|
||||
top_n_indices = (logprobs >= nth_highest).nonzero()
|
||||
_, top_n_ishes = torch.unique_consecutive(top_n_indices[:, 0], return_counts=True)
|
||||
|
||||
k = 1 if top_n_ishes.numel() == 0 else top_n_ishes.max()
|
||||
# Take a new topk for these new max n values
|
||||
top_k = torch.topk(logprobs, k=k, dim=1, sorted=True)
|
||||
top_k = torch.topk(logprobs, k=top_n_ishes.max(), dim=1, sorted=True)
|
||||
|
||||
top_n_ishes = top_n_ishes.tolist()
|
||||
top_indices = top_k.indices.tolist()
|
||||
top_values = top_k.values.tolist()
|
||||
|
||||
return (
|
||||
[
|
||||
idxs[:n] if req_n > 0 else []
|
||||
for idxs, n, req_n in zip(top_indices, top_n_ishes, top_n_tokens)
|
||||
],
|
||||
[
|
||||
vals[:n] if req_n > 0 else []
|
||||
for vals, n, req_n in zip(top_values, top_n_ishes, top_n_tokens)
|
||||
],
|
||||
[idxs[:n] if req_n > 0 else [] for idxs, n, req_n in zip(top_indices, top_n_ishes, top_n_tokens)],
|
||||
[vals[:n] if req_n > 0 else [] for vals, n, req_n in zip(top_values, top_n_ishes, top_n_tokens)],
|
||||
)
|
||||
|
@ -34,21 +34,17 @@ class WatermarkLogitsProcessor(LogitsProcessor):
|
||||
# watermarking parameters
|
||||
self.gamma = gamma
|
||||
self.delta = delta
|
||||
self.rng = torch.Generator(device=device)
|
||||
self.rng = torch.Generator(device="cpu")
|
||||
self.hash_key = hash_key
|
||||
|
||||
def _seed_rng(self, input_ids: Union[List[int], torch.LongTensor]):
|
||||
if isinstance(input_ids, list):
|
||||
assert (
|
||||
len(input_ids) >= 1
|
||||
), "requires at least a 1 token prefix sequence to seed rng"
|
||||
assert len(input_ids) >= 1, "requires at least a 1 token prefix sequence to seed rng"
|
||||
prev_token = input_ids[-1]
|
||||
else:
|
||||
assert len(input_ids) == 1
|
||||
input_ids = input_ids[0]
|
||||
assert (
|
||||
input_ids.shape[-1] >= 1
|
||||
), "requires at least a 1 token prefix sequence to seed rng"
|
||||
assert input_ids.shape[-1] >= 1, "requires at least a 1 token prefix sequence to seed rng"
|
||||
prev_token = input_ids[-1].item()
|
||||
self.rng.manual_seed(self.hash_key * prev_token)
|
||||
|
||||
@ -67,9 +63,7 @@ class WatermarkLogitsProcessor(LogitsProcessor):
|
||||
return greenlist_ids
|
||||
|
||||
@staticmethod
|
||||
def _calc_greenlist_mask(
|
||||
scores: torch.FloatTensor, greenlist_token_ids
|
||||
) -> torch.BoolTensor:
|
||||
def _calc_greenlist_mask(scores: torch.FloatTensor, greenlist_token_ids) -> torch.BoolTensor:
|
||||
green_tokens_mask = torch.zeros_like(scores)
|
||||
green_tokens_mask[-1, greenlist_token_ids] = 1
|
||||
final_mask = green_tokens_mask.bool()
|
||||
@ -82,15 +76,9 @@ class WatermarkLogitsProcessor(LogitsProcessor):
|
||||
scores[greenlist_mask] = scores[greenlist_mask] + greenlist_bias
|
||||
return scores
|
||||
|
||||
def __call__(
|
||||
self, input_ids: Union[List[int], torch.LongTensor], scores: torch.FloatTensor
|
||||
) -> torch.FloatTensor:
|
||||
greenlist_ids = self._get_greenlist_ids(
|
||||
input_ids, scores.shape[-1], scores.device
|
||||
)
|
||||
green_tokens_mask = self._calc_greenlist_mask(
|
||||
scores=scores, greenlist_token_ids=greenlist_ids
|
||||
)
|
||||
def __call__(self, input_ids: Union[List[int], torch.LongTensor], scores: torch.FloatTensor) -> torch.FloatTensor:
|
||||
greenlist_ids = self._get_greenlist_ids(input_ids, scores.shape[-1], scores.device)
|
||||
green_tokens_mask = self._calc_greenlist_mask(scores=scores, greenlist_token_ids=greenlist_ids)
|
||||
|
||||
scores = self._bias_greenlist_logits(
|
||||
scores=scores, greenlist_mask=green_tokens_mask, greenlist_bias=self.delta
|
||||
|
Loading…
Reference in New Issue
Block a user