diff --git a/.github/workflows/build.yaml b/.github/workflows/build.yaml
index 066ea889..f1131450 100644
--- a/.github/workflows/build.yaml
+++ b/.github/workflows/build.yaml
@@ -274,12 +274,105 @@ jobs:
cache-from: type=registry,ref=registry.internal.huggingface.tech/api-inference/community/text-generation-inference:cache-rocm,mode=min
cache-to: type=registry,ref=registry.internal.huggingface.tech/api-inference/community/text-generation-inference:cache-rocm,mode=min
+ build-and-push-image-intel:
+ concurrency:
+ group: ${{ github.workflow }}-build-and-push-image-intel-${{ github.head_ref || github.run_id }}
+ cancel-in-progress: true
+ needs:
+ - start-runner
+ - build-and-push-image # Wait for the main docker image to be built
+ - integration-tests # Wait for the main integration-tests
+ runs-on: ${{ needs.start-runner.outputs.label }} # run the job on the newly created runner
+ permissions:
+ contents: write
+ packages: write
+ # This is used to complete the identity challenge
+ # with sigstore/fulcio when running outside of PRs.
+ id-token: write
+ security-events: write
+ steps:
+ - name: Checkout repository
+ uses: actions/checkout@v3
+ - name: Initialize Docker Buildx
+ uses: docker/setup-buildx-action@v2.0.0
+ with:
+ install: true
+ - name: Inject slug/short variables
+ uses: rlespinasse/github-slug-action@v4.4.1
+ - name: Tailscale
+ uses: tailscale/github-action@7bd8039bf25c23c4ab1b8d6e2cc2da2280601966
+ with:
+ authkey: ${{ secrets.TAILSCALE_AUTHKEY }}
+ - name: Login to GitHub Container Registry
+ if: github.event_name != 'pull_request'
+ uses: docker/login-action@v2
+ with:
+ registry: ghcr.io
+ username: ${{ github.actor }}
+ password: ${{ secrets.GITHUB_TOKEN }}
+ - name: Login to internal Container Registry
+ uses: docker/login-action@v2.1.0
+ with:
+ username: ${{ secrets.TAILSCALE_DOCKER_USERNAME }}
+ password: ${{ secrets.TAILSCALE_DOCKER_PASSWORD }}
+ registry: registry.internal.huggingface.tech
+ - name: Login to Azure Container Registry
+ if: github.event_name != 'pull_request'
+ uses: docker/login-action@v2.1.0
+ with:
+ username: ${{ secrets.AZURE_DOCKER_USERNAME }}
+ password: ${{ secrets.AZURE_DOCKER_PASSWORD }}
+ registry: db4c2190dd824d1f950f5d1555fbadf0.azurecr.io
+ # If pull request
+ - name: Extract metadata (tags, labels) for Docker
+ if: ${{ github.event_name == 'pull_request' }}
+ id: meta-pr
+ uses: docker/metadata-action@v4.3.0
+ with:
+ images: |
+ registry.internal.huggingface.tech/api-inference/community/text-generation-inference
+ tags: |
+ type=raw,value=sha-${{ env.GITHUB_SHA_SHORT }}-intel
+ # If main, release or tag
+ - name: Extract metadata (tags, labels) for Docker
+ if: ${{ github.event_name != 'pull_request' }}
+ id: meta
+ uses: docker/metadata-action@v4.3.0
+ with:
+ flavor: |
+ latest=false
+ images: |
+ registry.internal.huggingface.tech/api-inference/community/text-generation-inference
+ ghcr.io/huggingface/text-generation-inference
+ db4c2190dd824d1f950f5d1555fbadf0.azurecr.io/text-generation-inference
+ tags: |
+ type=semver,pattern={{version}}-intel
+ type=semver,pattern={{major}}.{{minor}}-intel
+ type=raw,value=latest-intel,enable=${{ github.ref == format('refs/heads/{0}', github.event.repository.default_branch) }}
+ type=raw,value=sha-${{ env.GITHUB_SHA_SHORT }}-intel
+ - name: Build and push Docker image
+ id: build-and-push
+ uses: docker/build-push-action@v4
+ with:
+ context: .
+ file: Dockerfile_intel
+ push: true
+ platforms: 'linux/amd64'
+ build-args: |
+ GIT_SHA=${{ env.GITHUB_SHA }}
+ DOCKER_LABEL=sha-${{ env.GITHUB_SHA_SHORT }}-intel
+ tags: ${{ steps.meta.outputs.tags || steps.meta-pr.outputs.tags }}
+ labels: ${{ steps.meta.outputs.labels || steps.meta-pr.outputs.labels }}
+ cache-from: type=registry,ref=registry.internal.huggingface.tech/api-inference/community/text-generation-inference:cache-intel,mode=min
+ cache-to: type=registry,ref=registry.internal.huggingface.tech/api-inference/community/text-generation-inference:cache-intel,mode=min
+
stop-runner:
name: Stop self-hosted EC2 runner
needs:
- start-runner
- build-and-push-image
- build-and-push-image-rocm
+ - build-and-push-image-intel
- integration-tests
runs-on: ubuntu-latest
env:
diff --git a/.gitignore b/.gitignore
index b3ca772b..2ac2f6b4 100644
--- a/.gitignore
+++ b/.gitignore
@@ -11,3 +11,5 @@ server/exllama_kernels/exllama_kernels/hip_func/
*_hip.cuh
server/exllama_kernels/exllama_kernels/hip_buffers.cuh
server/exllama_kernels/exllama_kernels/exllama_ext_hip.cpp
+
+data/
diff --git a/Cargo.toml b/Cargo.toml
index 593fd950..34e55652 100644
--- a/Cargo.toml
+++ b/Cargo.toml
@@ -9,7 +9,7 @@ members = [
resolver = "2"
[workspace.package]
-version = "2.0.1"
+version = "2.0.2"
edition = "2021"
authors = ["Olivier Dehaene"]
homepage = "https://github.com/huggingface/text-generation-inference"
diff --git a/Dockerfile_intel b/Dockerfile_intel
new file mode 100644
index 00000000..d0791cac
--- /dev/null
+++ b/Dockerfile_intel
@@ -0,0 +1,105 @@
+FROM lukemathwalker/cargo-chef:latest-rust-1.75 AS chef
+WORKDIR /usr/src
+
+ARG CARGO_REGISTRIES_CRATES_IO_PROTOCOL=sparse
+
+FROM chef as planner
+COPY Cargo.toml Cargo.toml
+COPY rust-toolchain.toml rust-toolchain.toml
+COPY proto proto
+COPY benchmark benchmark
+COPY router router
+COPY launcher launcher
+RUN cargo chef prepare --recipe-path recipe.json
+
+FROM chef AS builder
+
+ARG GIT_SHA
+ARG DOCKER_LABEL
+
+RUN PROTOC_ZIP=protoc-21.12-linux-x86_64.zip && \
+ curl -OL https://github.com/protocolbuffers/protobuf/releases/download/v21.12/$PROTOC_ZIP && \
+ unzip -o $PROTOC_ZIP -d /usr/local bin/protoc && \
+ unzip -o $PROTOC_ZIP -d /usr/local 'include/*' && \
+ rm -f $PROTOC_ZIP
+
+COPY --from=planner /usr/src/recipe.json recipe.json
+RUN cargo chef cook --release --recipe-path recipe.json
+
+COPY Cargo.toml Cargo.toml
+COPY rust-toolchain.toml rust-toolchain.toml
+COPY proto proto
+COPY benchmark benchmark
+COPY router router
+COPY launcher launcher
+RUN cargo build --release
+
+
+# Text Generation Inference base image for Intel
+FROM intel/intel-extension-for-pytorch:2.1.10-xpu as base
+
+USER root
+# libssl.so.1.1 is not installed on Ubuntu 22.04 by default, install it
+RUN wget http://nz2.archive.ubuntu.com/ubuntu/pool/main/o/openssl/libssl1.1_1.1.1f-1ubuntu2_amd64.deb && \
+ dpkg -i ./libssl1.1_1.1.1f-1ubuntu2_amd64.deb
+
+
+RUN wget -O- https://apt.repos.intel.com/intel-gpg-keys/GPG-PUB-KEY-INTEL-SW-PRODUCTS.PUB \
+| gpg --dearmor | tee /usr/share/keyrings/oneapi-archive-keyring.gpg > /dev/null && echo "deb [signed-by=/usr/share/keyrings/oneapi-archive-keyring.gpg] https://apt.repos.intel.com/oneapi all main" | tee /etc/apt/sources.list.d/oneAPI.list
+
+RUN apt-get update && apt install -y intel-basekit xpu-smi cmake python3-dev ninja-build
+
+# Text Generation Inference base env
+ENV HUGGINGFACE_HUB_CACHE=/data \
+ HF_HUB_ENABLE_HF_TRANSFER=1 \
+ PORT=80
+
+
+WORKDIR /usr/src
+# Build pytorch and ipex
+RUN git clone https://github.com/intel/intel-extension-for-pytorch && cd intel-extension-for-pytorch && git checkout -b xpu_main origin/xpu-main
+RUN git clone https://github.com/pytorch/pytorch.git && cd pytorch && git checkout 209f2fa8ff86652f67d75c2f19bf9cb9942fd018 && git apply /usr/src/intel-extension-for-pytorch/torch_patches/00*.patch
+
+# Install server
+COPY proto proto
+COPY server server
+COPY server/Makefile server/Makefile
+RUN cd server && \
+ make gen-server && \
+ pip install -r requirements_cuda.txt && \
+ pip install ".[accelerate, peft, outlines]" --no-cache-dir
+
+ENV CCL_ROOT=/opt/intel/oneapi/ccl/latest
+ENV I_MPI_ROOT=/opt/intel/oneapi/mpi/latest
+ENV FI_PROVIDER_PATH=/opt/intel/oneapi/mpi/latest/opt/mpi/libfabric/lib/prov:/usr/lib/x86_64-linux-gnu/libfabric
+ENV DIAGUTIL_PATH=/opt/intel/oneapi/compiler/latest/etc/compiler/sys_check/sys_check.sh
+ENV CCL_CONFIGURATION=cpu_gpu_dpcpp
+ENV MANPATH=/opt/intel/oneapi/mpi/latest/share/man:/opt/intel/oneapi/mpi/latest/share/man:/opt/intel/oneapi/compiler/latest/share/man
+ENV CMAKE_PREFIX_PATH=/opt/intel/oneapi/mkl/latest/lib/cmake:/opt/intel/oneapi/compiler/latest
+ENV CMPLR_ROOT=/opt/intel/oneapi/compiler/latest
+ENV LIBRARY_PATH=/opt/intel/oneapi/mpi/latest/lib:/opt/intel/oneapi/ccl/latest/lib/:/opt/intel/oneapi/mkl/latest/lib/:/opt/intel/oneapi/compiler/latest/lib
+ENV OCL_ICD_FILENAMES=libintelocl_emu.so:libalteracl.so:/opt/intel/oneapi/compiler/latest/lib/libintelocl.so
+ENV CLASSPATH=/opt/intel/oneapi/mpi/latest/share/java/mpi.jar:/opt/intel/oneapi/mpi/latest/share/java/mpi.jar
+ENV LD_LIBRARY_PATH=/opt/intel/oneapi/ccl/latest/lib/:/opt/intel/oneapi/mpi/latest/opt/mpi/libfabric/lib:/opt/intel/oneapi/mpi/latest/lib:/opt/intel/oneapi/mkl/latest/lib:/opt/intel/oneapi/compiler/latest/opt/compiler/lib:/opt/intel/oneapi/compiler/latest/lib:/opt/intel/oneapi/lib:/opt/intel/oneapi/lib/intel64:
+ENV MKLROOT=/opt/intel/oneapi/mkl/latest
+ENV NLSPATH=/opt/intel/oneapi/mkl/latest/share/locale/%l_%t/%N:/opt/intel/oneapi/compiler/latest/lib/locale/%l_%t/%N
+ENV PATH=/opt/intel/oneapi/mpi/latest/opt/mpi/libfabric/bin:/opt/intel/oneapi/mpi/latest/bin:/opt/intel/oneapi/mpi/latest/opt/mpi/libfabric/bin:/opt/intel/oneapi/mkl/latest/bin/:/opt/intel/oneapi/compiler/latest/bin:/usr/local/sbin:/usr/local/bin:/usr/sbin:/usr/bin:/sbin:/bin
+ENV CPATH=/opt/intel/oneapi/mpi/latest/include:/opt/intel/oneapi/ccl/latest/include:/opt/intel/oneapi/mkl/latest/include
+ENV CCL_ZE_IPC_EXCHANGE=sockets
+
+
+RUN pip uninstall -y torch && cd pytorch && git submodule update --init --recursive && python setup.py install
+RUN pip uninstall -y intel-extension-for-pytorch && cd intel-extension-for-pytorch && git submodule update --init --recursive && USE_AOT_DEVLIST='pvc' BUILD_SEPARATE_OPS=ON BUILD_WITH_CPU=ON USE_XETLA=ON python setup.py install
+
+# Install benchmarker
+COPY --from=builder /usr/src/target/release/text-generation-benchmark /usr/local/bin/text-generation-benchmark
+# Install router
+COPY --from=builder /usr/src/target/release/text-generation-router /usr/local/bin/text-generation-router
+# Install launcher
+COPY --from=builder /usr/src/target/release/text-generation-launcher /usr/local/bin/text-generation-launcher
+
+# Final image
+FROM base
+
+ENTRYPOINT ["text-generation-launcher"]
+CMD ["--json-output"]
diff --git a/clients/python/text_generation/client.py b/clients/python/text_generation/client.py
index 95d23901..0e86901d 100644
--- a/clients/python/text_generation/client.py
+++ b/clients/python/text_generation/client.py
@@ -80,6 +80,7 @@ class Client:
temperature: Optional[float] = None,
top_p: Optional[float] = None,
tools: Optional[List[Tool]] = None,
+ tool_prompt: Optional[str] = None,
tool_choice: Optional[str] = None,
):
"""
@@ -119,6 +120,8 @@ class Client:
higher are kept for generation
tools (`List[Tool]`):
List of tools to use
+ tool_prompt (`str`):
+ A prompt to be appended before the tools
tool_choice (`str`):
The tool to use
@@ -139,6 +142,7 @@ class Client:
temperature=temperature,
top_p=top_p,
tools=tools,
+ tool_prompt=tool_prompt,
tool_choice=tool_choice,
)
if not stream:
@@ -466,6 +470,7 @@ class AsyncClient:
temperature: Optional[float] = None,
top_p: Optional[float] = None,
tools: Optional[List[Tool]] = None,
+ tool_prompt: Optional[str] = None,
tool_choice: Optional[str] = None,
) -> Union[ChatComplete, AsyncIterator[ChatCompletionChunk]]:
"""
@@ -505,6 +510,8 @@ class AsyncClient:
higher are kept for generation
tools (`List[Tool]`):
List of tools to use
+ tool_prompt (`str`):
+ A prompt to be appended before the tools
tool_choice (`str`):
The tool to use
@@ -525,6 +532,7 @@ class AsyncClient:
temperature=temperature,
top_p=top_p,
tools=tools,
+ tool_prompt=tool_prompt,
tool_choice=tool_choice,
)
if not stream:
diff --git a/clients/python/text_generation/types.py b/clients/python/text_generation/types.py
index cfa2a9ed..5e32bc6f 100644
--- a/clients/python/text_generation/types.py
+++ b/clients/python/text_generation/types.py
@@ -159,6 +159,8 @@ class ChatRequest(BaseModel):
top_p: Optional[float] = None
# List of tools to be used
tools: Optional[List[Tool]] = None
+ # A prompt to be appended before the tools
+ tool_prompt: Optional[str] = None
# Choice of tool to be used
tool_choice: Optional[str] = None
diff --git a/docs/source/_toctree.yml b/docs/source/_toctree.yml
index 1598c248..c815b535 100644
--- a/docs/source/_toctree.yml
+++ b/docs/source/_toctree.yml
@@ -25,6 +25,10 @@
title: Non-core Model Serving
- local: basic_tutorials/safety
title: Safety
+ - local: basic_tutorials/using_guidance
+ title: Using Guidance, JSON, tools
+ - local: basic_tutorials/visual_language_models
+ title: Visual Language Models
title: Tutorials
- sections:
- local: conceptual/streaming
@@ -42,5 +46,6 @@
- local: conceptual/speculation
title: Speculation (Medusa, ngram)
- local: conceptual/guidance
- title: Guidance, JSON, tools (using outlines)
+ title: How Guidance Works (via outlines)
+
title: Conceptual Guides
diff --git a/docs/source/basic_tutorials/launcher.md b/docs/source/basic_tutorials/launcher.md
index de7c995d..1e5b6fd2 100644
--- a/docs/source/basic_tutorials/launcher.md
+++ b/docs/source/basic_tutorials/launcher.md
@@ -162,7 +162,7 @@ Options:
This setting is only applied if there is room in the batch as defined by `max_batch_total_tokens`.
[env: WAITING_SERVED_RATIO=]
- [default: 1.2]
+ [default: 0.3]
```
## MAX_BATCH_PREFILL_TOKENS
diff --git a/docs/source/basic_tutorials/using_guidance.md b/docs/source/basic_tutorials/using_guidance.md
new file mode 100644
index 00000000..606f2453
--- /dev/null
+++ b/docs/source/basic_tutorials/using_guidance.md
@@ -0,0 +1,419 @@
+# Guidance
+
+Text Generation Inference (TGI) now supports [JSON and regex grammars](#grammar-and-constraints) and [tools and functions](#tools-and-functions) to help developers guide LLM responses to fit their needs.
+
+These feature are available starting from version `1.4.3`. They are accessible via the [text_generation](https://pypi.org/project/text-generation/) library. The tool support is compatible with OpenAI's client libraries. The following guide will walk you through the new features and how to use them!
+
+_note: guidance is supported as grammar in the `/generate` endpoint and as tools in the `/chat/completions` endpoint._
+
+## How it works
+
+TGI leverages the [outlines](https://github.com/outlines-dev/outlines) library to efficiently parse and compile the grammatical structures and tools specified by users. This integration transforms the defined grammars into an intermediate representation that acts as a framework to guide and constrain content generation, ensuring that outputs adhere to the specified grammatical rules.
+
+If you are interested in the technical details on how outlines is used in TGI, you can check out the [conceptual guidance documentation](../conceptual/guidance).
+
+## Table of Contents 📚
+
+### Grammar and Constraints
+
+- [The Grammar Parameter](#the-grammar-parameter): Shape your AI's responses with precision.
+- [Constrain with Pydantic](#constrain-with-pydantic): Define a grammar using Pydantic models.
+- [JSON Schema Integration](#json-schema-integration): Fine-grained control over your requests via JSON schema.
+- [Using the client](#using-the-client): Use TGI's client libraries to shape the AI's responses.
+
+### Tools and Functions
+
+- [The Tools Parameter](#the-tools-parameter): Enhance the AI's capabilities with predefined functions.
+- [Via the client](#text-generation-inference-client): Use TGI's client libraries to interact with the Messages API and Tool functions.
+- [OpenAI integration](#openai-integration): Use OpenAI's client libraries to interact with TGI's Messages API and Tool functions.
+
+## Grammar and Constraints 🛣️
+
+### The Grammar Parameter
+
+In TGI `1.4.3`, we've introduced the grammar parameter, which allows you to specify the format of the response you want from the LLM.
+
+Using curl, you can make a request to TGI's Messages API with the grammar parameter. This is the most primitive way to interact with the API and using [Pydantic](#constrain-with-pydantic) is recommended for ease of use and readability.
+
+```json
+curl localhost:3000/generate \
+ -X POST \
+ -H 'Content-Type: application/json' \
+ -d '{
+ "inputs": "I saw a puppy a cat and a raccoon during my bike ride in the park",
+ "parameters": {
+ "repetition_penalty": 1.3,
+ "grammar": {
+ "type": "json",
+ "value": {
+ "properties": {
+ "location": {
+ "type": "string"
+ },
+ "activity": {
+ "type": "string"
+ },
+ "animals_seen": {
+ "type": "integer",
+ "minimum": 1,
+ "maximum": 5
+ },
+ "animals": {
+ "type": "array",
+ "items": {
+ "type": "string"
+ }
+ }
+ },
+ "required": ["location", "activity", "animals_seen", "animals"]
+ }
+ }
+ }
+}'
+// {"generated_text":"{ \n\n\"activity\": \"biking\",\n\"animals\": [\"puppy\",\"cat\",\"raccoon\"],\n\"animals_seen\": 3,\n\"location\": \"park\"\n}"}
+
+```
+
+A grammar can be defined using Pydantic models, JSON schemas, or regular expressions. The LLM will then generate a response that conforms to the specified grammar.
+
+> Note: A grammar must compile to an intermediate representation to constrain the output. Grammar compilation is a computationally expensive and may take a few seconds to complete on the first request. Subsequent requests will use the cached grammar and will be much faster.
+
+### Constrain with Pydantic
+
+Using Pydantic models we can define a similar grammar as the previous example in a shorter and more readable way.
+
+```python
+import requests
+from pydantic import BaseModel, conint
+from typing import List
+
+class Animals(BaseModel):
+ location: str
+ activity: str
+ animals_seen: conint(ge=1, le=5) # Constrained integer type
+ animals: List[str]
+
+prompt = "convert to JSON: I saw a puppy a cat and a raccoon during my bike ride in the park"
+
+data = {
+ "inputs": prompt,
+ "parameters": {
+ "repetition_penalty": 1.3,
+ "grammar": {
+ "type": "json",
+ "value": Animals.schema()
+ }
+ }
+}
+
+headers = {
+ "Content-Type": "application/json",
+}
+
+response = requests.post(
+ 'http://127.0.0.1:3000/generate',
+ headers=headers,
+ json=data
+)
+print(response.json())
+# {'generated_text': '{ "activity": "bike riding", "animals": ["puppy","cat","raccoon"],"animals_seen": 3, "location":"park" }'}
+
+```
+
+### JSON Schema Integration
+
+If Pydantic's not your style, go raw with direct JSON Schema integration. This is similar to the first example but with programmatic control.
+
+```python
+import requests
+
+json_schema = {
+ "properties": {
+ "location": {
+ "type": "string"
+ },
+ "activity": {
+ "type": "string"
+ },
+ "animals_seen": {
+ "type": "integer",
+ "minimum": 1,
+ "maximum": 5
+ },
+ "animals": {
+ "type": "array",
+ "items": {
+ "type": "string"
+ }
+ }
+ },
+ "required": ["location", "activity", "animals_seen", "animals"]
+}
+
+data = {
+ "inputs": "convert to JSON: I saw a puppy a cat and a raccoon during my bike ride in the park",
+ "parameters": {
+ "max_new_tokens": 200,
+ "repetition_penalty": 1.3,
+ "grammar": {
+ "type": "json",
+ "value": json_schema
+ }
+ }
+}
+
+headers = {
+ "Content-Type": "application/json",
+}
+
+response = requests.post(
+ 'http://127.0.0.1:3000/generate',
+ headers=headers,
+ json=data
+)
+print(response.json())
+# {'generated_text': '{\n"activity": "biking",\n"animals": ["puppy","cat","raccoon"]\n , "animals_seen": 3,\n "location":"park"}'}
+
+```
+
+### Using the client
+
+TGI provides a client library to that make it easy to send requests with all of the parameters we've discussed above. Here's an example of how to use the client to send a request with a grammar parameter.
+
+```python
+from text_generation import AsyncClient
+from text_generation.types import GrammarType
+
+# NOTE: tools defined above and removed for brevity
+
+# Define an async function to encapsulate the async operation
+async def main():
+ client = AsyncClient(base_url="http://localhost:3000")
+
+ # Use 'await' to wait for the async method 'chat' to complete
+ response = await client.generate(
+ "Whats Googles DNS",
+ max_new_tokens=10,
+ decoder_input_details=True,
+ seed=1,
+ grammar={
+ "type": GrammarType.Regex,
+ "value": "((25[0-5]|2[0-4]\\d|[01]?\\d\\d?)\\.){3}(25[0-5]|2[0-4]\\d|[01]?\\d\\d?)",
+ },
+ )
+
+ # Once the response is received, you can process it
+ print(response.generated_text)
+
+# Ensure the main async function is run in the event loop
+if __name__ == "__main__":
+ import asyncio
+ asyncio.run(main())
+
+# 118.8.0.84
+
+```
+
+## Tools and Functions 🛠️
+
+### The Tools Parameter
+
+In addition to the grammar parameter, we've also introduced a set of tools and functions to help you get the most out of the Messages API.
+
+Tools are a set of user defined functions that can be used in tandem with the chat functionality to enhance the LLM's capabilities. Functions, similar to grammar are defined as JSON schema and can be passed as part of the parameters to the Messages API.
+
+Functions, similar to grammar are defined as JSON schema and can be passed as part of the parameters to the Messages API.
+
+```json
+curl localhost:3000/v1/chat/completions \
+ -X POST \
+ -H 'Content-Type: application/json' \
+ -d '{
+ "model": "tgi",
+ "messages": [
+ {
+ "role": "user",
+ "content": "What is the weather like in New York?"
+ }
+ ],
+ "tools": [
+ {
+ "type": "function",
+ "function": {
+ "name": "get_current_weather",
+ "description": "Get the current weather",
+ "parameters": {
+ "type": "object",
+ "properties": {
+ "location": {
+ "type": "string",
+ "description": "The city and state, e.g. San Francisco, CA"
+ },
+ "format": {
+ "type": "string",
+ "enum": ["celsius", "fahrenheit"],
+ "description": "The temperature unit to use. Infer this from the users location."
+ }
+ },
+ "required": ["location", "format"]
+ }
+ }
+ }
+ ],
+ "tool_choice": "get_current_weather"
+}'
+// {"id":"","object":"text_completion","created":1709051640,"model":"HuggingFaceH4/zephyr-7b-beta","system_fingerprint":"1.4.3-native","choices":[{"index":0,"message":{"role":"assistant","tool_calls":{"id":0,"type":"function","function":{"description":null,"name":"tools","parameters":{"format":"celsius","location":"New York"}}}},"logprobs":null,"finish_reason":"eos_token"}],"usage":{"prompt_tokens":157,"completion_tokens":19,"total_tokens":176}}
+```
+
+### Text Generation Inference Client
+
+TGI provides a client library to interact with the Messages API and Tool functions. The client library is available in both synchronous and asynchronous versions.
+
+```python
+from text_generation import AsyncClient
+
+# NOTE: tools defined above and removed for brevity
+
+# Define an async function to encapsulate the async operation
+async def main():
+ client = AsyncClient(base_url="http://localhost:3000")
+
+ # Use 'await' to wait for the async method 'chat' to complete
+ response = await client.chat(
+ max_tokens=100,
+ seed=1,
+ tools=tools,
+ presence_penalty=-1.1,
+ messages=[
+ {
+ "role": "system",
+ "content": "You're a helpful assistant! Answer the users question best you can.",
+ },
+ {
+ "role": "user",
+ "content": "What is the weather like in Brooklyn, New York?",
+ },
+ ],
+ )
+
+ # Once the response is received, you can process it
+ print(response.choices[0].message.tool_calls)
+
+# Ensure the main async function is run in the event loop
+if __name__ == "__main__":
+ import asyncio
+ asyncio.run(main())
+
+# {"id":"","object":"text_completion","created":1709051942,"model":"HuggingFaceH4/zephyr-7b-beta","system_fingerprint":"1.4.3-native","choices":[{"index":0,"message":{"role":"assistant","tool_calls":{"id":0,"type":"function","function":{"description":null,"name":"tools","parameters":{"format":"celsius","location":"New York"}}}},"logprobs":null,"finish_reason":"eos_token"}],"usage":{"prompt_tokens":157,"completion_tokens":20,"total_tokens":177}}
+
+```
+
+
+ Tools used in example above
+
+```python
+ tools = [
+ {
+ "type": "function",
+ "function": {
+ "name": "get_current_weather",
+ "description": "Get the current weather",
+ "parameters": {
+ "type": "object",
+ "properties": {
+ "location": {
+ "type": "string",
+ "description": "The city and state, e.g. San Francisco, CA",
+ },
+ "format": {
+ "type": "string",
+ "enum": ["celsius", "fahrenheit"],
+ "description": "The temperature unit to use. Infer this from the users location.",
+ },
+ },
+ "required": ["location", "format"],
+ },
+ },
+ },
+ {
+ "type": "function",
+ "function": {
+ "name": "get_n_day_weather_forecast",
+ "description": "Get an N-day weather forecast",
+ "parameters": {
+ "type": "object",
+ "properties": {
+ "location": {
+ "type": "string",
+ "description": "The city and state, e.g. San Francisco, CA",
+ },
+ "format": {
+ "type": "string",
+ "enum": ["celsius", "fahrenheit"],
+ "description": "The temperature unit to use. Infer this from the users location.",
+ },
+ "num_days": {
+ "type": "integer",
+ "description": "The number of days to forecast",
+ },
+ },
+ "required": ["location", "format", "num_days"],
+ },
+ },
+ }
+ ]
+```
+
+
+
+### OpenAI integration
+
+TGI exposes an OpenAI-compatible API, which means you can use OpenAI's client libraries to interact with TGI's Messages API and Tool functions.
+
+However there are some minor differences in the API, for example `tool_choice="auto"` will ALWAYS choose the tool for you. This is different from OpenAI's API where `tool_choice="auto"` will choose a tool if the model thinks it's necessary.
+
+```python
+from openai import OpenAI
+
+# Initialize the client, pointing it to one of the available models
+client = OpenAI(
+ base_url="http://localhost:3000/v1",
+ api_key="_",
+)
+
+# NOTE: tools defined above and removed for brevity
+
+chat_completion = client.chat.completions.create(
+ model="tgi",
+ messages=[
+ {
+ "role": "system",
+ "content": "Don't make assumptions about what values to plug into functions. Ask for clarification if a user request is ambiguous.",
+ },
+ {
+ "role": "user",
+ "content": "What's the weather like the next 3 days in San Francisco, CA?",
+ },
+ ],
+ tools=tools,
+ tool_choice="auto", # tool selected by model
+ max_tokens=500,
+)
+
+
+called = chat_completion.choices[0].message.tool_calls
+print(called)
+# {
+# "id": 0,
+# "type": "function",
+# "function": {
+# "description": None,
+# "name": "tools",
+# "parameters": {
+# "format": "celsius",
+# "location": "San Francisco, CA",
+# "num_days": 3,
+# },
+# },
+# }
+```
diff --git a/docs/source/basic_tutorials/visual_language_models.md b/docs/source/basic_tutorials/visual_language_models.md
new file mode 100644
index 00000000..e804ef09
--- /dev/null
+++ b/docs/source/basic_tutorials/visual_language_models.md
@@ -0,0 +1,170 @@
+# Vision Language Model Inference in TGI
+
+Visual Language Model (VLM) are models that consume both image and text inputs to generate text.
+
+VLM's are trained on a combination of image and text data and can handle a wide range of tasks, such as image captioning, visual question answering, and visual dialog.
+
+> What distinguishes VLMs from other text and image models is their ability to handle long context and generate text that is coherent and relevant to the image even after multiple turns or in some cases, multiple images.
+
+Below are couple of common use cases for vision language models:
+
+- **Image Captioning**: Given an image, generate a caption that describes the image.
+- **Visual Question Answering (VQA)**: Given an image and a question about the image, generate an answer to the question.
+- **Mulimodal Dialog**: Generate response to multiple turns of images and conversations.
+- **Image Information Retrieval**: Given an image, retrieve information from the image.
+
+## How to Use a Vision Language Model?
+
+### Hugging Face Hub Python Library
+
+To infer with vision language models through Python, you can use the [`huggingface_hub`](https://pypi.org/project/huggingface-hub/) library. The `InferenceClient` class provides a simple way to interact with the [Inference API](https://huggingface.co/docs/api-inference/index). Images can be passed as URLs or base64-encoded strings. The `InferenceClient` will automatically detect the image format.
+
+```python
+from huggingface_hub import InferenceClient
+
+client = InferenceClient("http://127.0.0.1:3000")
+image = "https://huggingface.co/datasets/huggingface/documentation-images/resolve/main/transformers/rabbit.png"
+prompt = f"What is this a picture of?\n\n"
+for token in client.text_generation(prompt, max_new_tokens=16, stream=True):
+ print(token)
+
+# This is a picture of an anthropomorphic rabbit in a space suit.
+```
+
+```python
+from huggingface_hub import InferenceClient
+import base64
+import requests
+import io
+
+client = InferenceClient("http://127.0.0.1:3000")
+
+# read image from local file
+image_path = "rabbit.png"
+with open(image_path, "rb") as f:
+ image = base64.b64encode(f.read()).decode("utf-8")
+
+image = f"data:image/png;base64,{image}"
+prompt = f"What is this a picture of?\n\n"
+
+for token in client.text_generation(prompt, max_new_tokens=10, stream=True):
+ print(token)
+
+# This is a picture of an anthropomorphic rabbit in a space suit.
+```
+
+If you want additional details, you can add `details=True`. In this case, you get a `TextGenerationStreamResponse` which contains additional information such as the probabilities and the tokens. For the final response in the stream, it also returns the full generated text.
+
+### Inference Through Sending `cURL` Requests
+
+To use the `generate_stream` endpoint with curl, you can add the `-N` flag. This flag disables curl default buffering and shows data as it arrives from the server.
+
+```bash
+curl -N 127.0.0.1:3000/generate_stream \
+ -X POST \
+ -d '{"inputs":"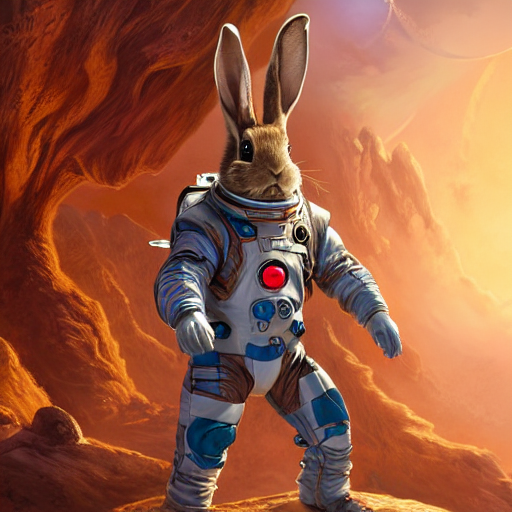What is this a picture of?\n\n","parameters":{"max_new_tokens":16, "seed": 42}}' \
+ -H 'Content-Type: application/json'
+
+# ...
+# data:{"index":16,"token":{"id":28723,"text":".","logprob":-0.6196289,"special":false},"generated_text":"This is a picture of an anthropomorphic rabbit in a space suit.","details":null}
+```
+
+### Inference Through JavaScript
+
+First, we need to install the `@huggingface/inference` library.
+
+```bash
+npm install @huggingface/inference
+```
+
+If you're using the free Inference API, you can use [Huggingface.js](https://huggingface.co/docs/huggingface.js/inference/README)'s `HfInference`. If you're using inference endpoints, you can use `HfInferenceEndpoint` class to easily interact with the Inference API.
+
+We can create a `HfInferenceEndpoint` providing our endpoint URL and We can create a `HfInferenceEndpoint` providing our endpoint URL and [Hugging Face access token](https://huggingface.co/settings/tokens).
+
+```js
+import { HfInferenceEndpoint } from "@huggingface/inference";
+
+const hf = new HfInferenceEndpoint("http://127.0.0.1:3000", "HF_TOKEN");
+
+const prompt =
+ "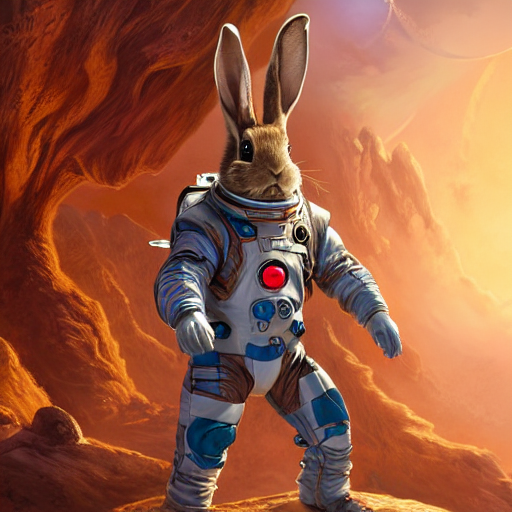What is this a picture of?\n\n";
+
+const stream = hf.textGenerationStream({
+ inputs: prompt,
+ parameters: { max_new_tokens: 16, seed: 42 },
+});
+for await (const r of stream) {
+ // yield the generated token
+ process.stdout.write(r.token.text);
+}
+
+// This is a picture of an anthropomorphic rabbit in a space suit.
+```
+
+## Combining Vision Language Models with Other Features
+
+VLMs in TGI have several advantages, for example these models can be used in tandem with other features for more complex tasks. For example, you can use VLMs with [Guided Generation](/docs/conceptual/guided-generation) to generate specific JSON data from an image.
+
+
+
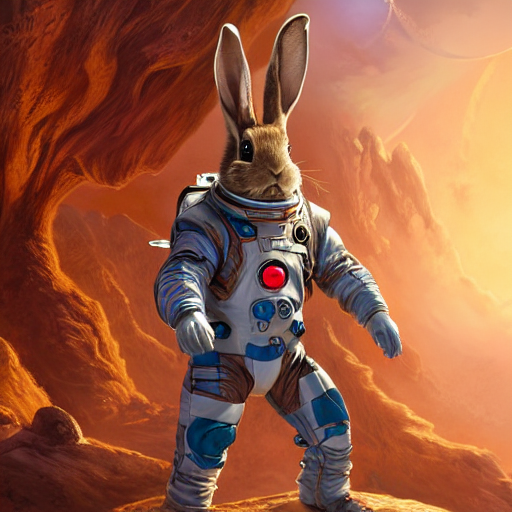
+
+
+For example we can extract information from the rabbit image and generate a JSON object with the location, activity, number of animals seen, and the animals seen. That would look like this:
+
+```json
+{
+ "activity": "Standing",
+ "animals": ["Rabbit"],
+ "animals_seen": 1,
+ "location": "Rocky surface with mountains in the background and a red light on the rabbit's chest"
+}
+```
+
+All we need to do is provide a JSON schema to the VLM model and it will generate the JSON object for us.
+
+```bash
+curl localhost:3000/generate \
+ -X POST \
+ -H 'Content-Type: application/json' \
+ -d '{
+ "inputs":"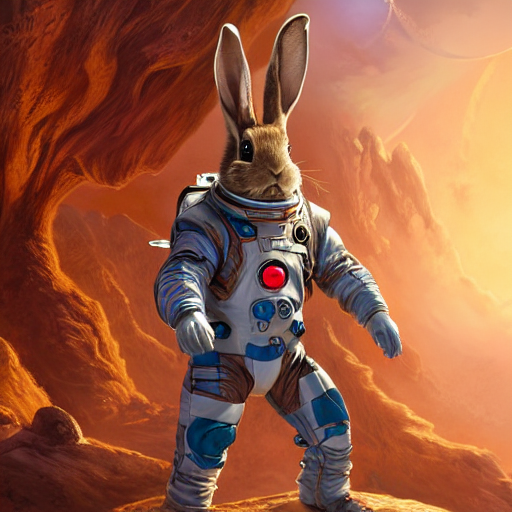What is this a picture of?\n\n",
+ "parameters": {
+ "max_new_tokens": 100,
+ "seed": 42,
+ "grammar": {
+ "type": "json",
+ "value": {
+ "properties": {
+ "location": {
+ "type": "string"
+ },
+ "activity": {
+ "type": "string"
+ },
+ "animals_seen": {
+ "type": "integer",
+ "minimum": 1,
+ "maximum": 5
+ },
+ "animals": {
+ "type": "array",
+ "items": {
+ "type": "string"
+ }
+ }
+ },
+ "required": ["location", "activity", "animals_seen", "animals"]
+ }
+ }
+ }
+}'
+
+# {
+# "generated_text": "{ \"activity\": \"Standing\", \"animals\": [ \"Rabbit\" ], \"animals_seen\": 1, \"location\": \"Rocky surface with mountains in the background and a red light on the rabbit's chest\" }"
+# }
+```
+
+Want to learn more about how Vision Language Models work? Check out the [awesome blog post on the topic](https://huggingface.co/blog/vlms).
diff --git a/docs/source/conceptual/guidance.md b/docs/source/conceptual/guidance.md
index 0a3bbd60..0ce34f2f 100644
--- a/docs/source/conceptual/guidance.md
+++ b/docs/source/conceptual/guidance.md
@@ -1,419 +1,86 @@
# Guidance
-Text Generation Inference (TGI) now supports [JSON and regex grammars](#grammar-and-constraints) and [tools and functions](#tools-and-functions) to help developer guide LLM responses to fit their needs.
+## What is Guidance?
-These feature are available starting from version `1.4.3`. They are accessible via the [text_generation](https://pypi.org/project/text-generation/) library and is compatible with OpenAI's client libraries. The following guide will walk you through the new features and how to use them!
+Guidance is a feature that allows users to constrain the generation of a large language model with a specified grammar. This feature is particularly useful when you want to generate text that follows a specific structure or uses a specific set of words or produce output in a specific format.
-## Quick Start
+## How is it used?
-Before we jump into the deep end, ensure your system is using TGI version `1.4.3` or later to access all the features we're about to explore in this guide.
+Guidance can be in many ways and the community is always finding new ways to use it. Here are some examples of how you can use guidance:
-If you're not up to date, grab the latest version and let's get started!
+Technically, guidance can be used to generate:
-## Table of Contents 📚
+- a specific JSON object
+- a function signature
+- typed output like a list of integers
-### Grammar and Constraints
+However these use cases can span a wide range of applications, such as:
-- [The Grammar Parameter](#the-grammar-parameter): Shape your AI's responses with precision.
-- [Constrain with Pydantic](#constrain-with-pydantic): Define a grammar using Pydantic models.
-- [JSON Schema Integration](#json-schema-integration): Fine grain control over your requests via JSON schema.
-- [Using the client](#using-the-client): Use TGI's client libraries to shape the AI's responses.
+- extracting structured data from unstructured text
+- summarizing text into a specific format
+- limit output to specific classes of words (act as a LLM powered classifier)
+- generate the input to specific APIs or services
+- provide reliable and consistent output for downstream tasks
+- extract data from multimodal inputs
-### Tools and Functions
+## How it works?
-- [The Tools Parameter](#the-tools-parameter): Enhance the AI's capabilities with predefined functions.
-- [Via the client](#text-generation-inference-client): Use TGI's client libraries to interact with the Messages API and Tool functions.
-- [OpenAI integration](#openai-integration): Use OpenAI's client libraries to interact with TGI's Messages API and Tool functions.
+Diving into the details, guidance is enabled by including a grammar with a generation request that is compiled, and used to modify the chosen tokens.
-## Grammar and Constraints 🛣️
+This process can be broken down into the following steps:
-### The Grammar Parameter
+1. A request is sent to the backend, it is processed and placed in batch. Processing includes compiling the grammar into a finite state machine and a grammar state.
-In TGI `1.4.3`, we've introduced the grammar parameter, which allows you to specify the format of the response you want from the AI. This is a game-changer for those who need precise control over the AI's output.
+
+
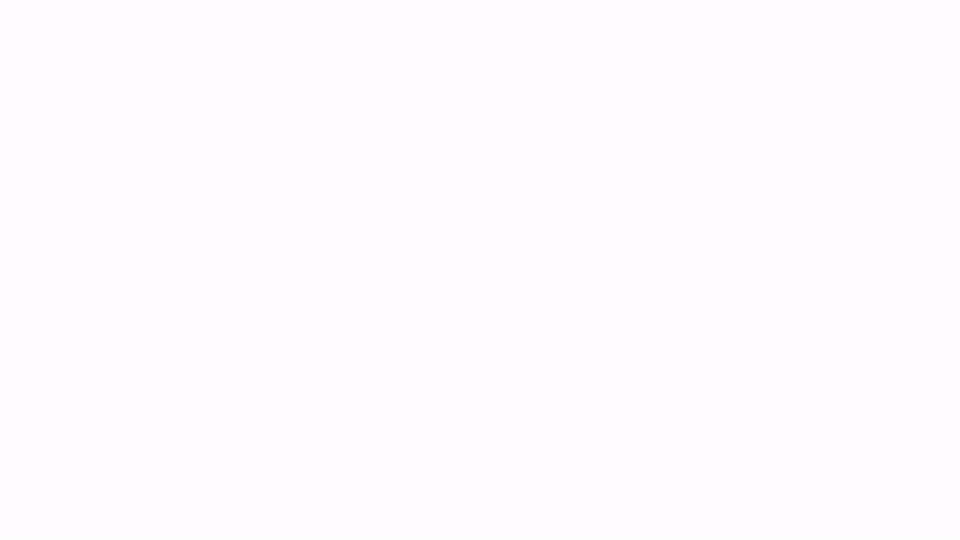
+

+
-Using curl, you can make a request to TGI's Messages API with the grammar parameter. This is the most primitive way to interact with the API and using [Pydantic](#constrain-with-pydantic) is recommended for ease of use and readability.
+2. The model does a forward pass over the batch. This returns probabilities for each token in the vocabulary for each request in the batch.
-```json
-curl localhost:3000/generate \
- -X POST \
- -H 'Content-Type: application/json' \
- -d '{
- "inputs": "I saw a puppy a cat and a raccoon during my bike ride in the park",
- "parameters": {
- "repetition_penalty": 1.3,
- "grammar": {
- "type": "json",
- "value": {
- "properties": {
- "location": {
- "type": "string"
- },
- "activity": {
- "type": "string"
- },
- "animals_seen": {
- "type": "integer",
- "minimum": 1,
- "maximum": 5
- },
- "animals": {
- "type": "array",
- "items": {
- "type": "string"
- }
- }
- },
- "required": ["location", "activity", "animals_seen", "animals"]
- }
- }
- }
-}'
-// {"generated_text":"{ \n\n\"activity\": \"biking\",\n\"animals\": [\"puppy\",\"cat\",\"raccoon\"],\n\"animals_seen\": 3,\n\"location\": \"park\"\n}"}
+3. The process of choosing one of those tokens is called `sampling`. The model samples from the distribution of probabilities to choose the next token. In TGI all of the steps before sampling are called `processor`. Grammars are applied as a processor that masks out tokens that are not allowed by the grammar.
-```
+
+

+
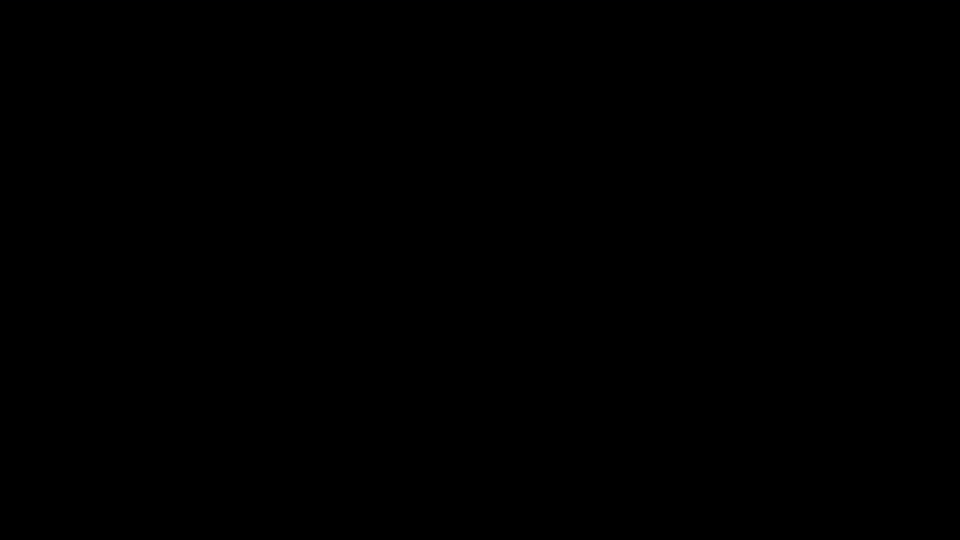
+
-A grammar can be defined using Pydantic models, JSON schema, or regular expressions. The AI will then generate a response that conforms to the specified grammar.
+4. The grammar mask is applied and the model samples from the remaining tokens. Once a token is chosen, we update the grammar state with the new token, to prepare it for the next pass.
-> Note: A grammar must compile to a intermediate representation to constrain the output. Grammar compilation is a computationally expensive and may take a few seconds to complete on the first request. Subsequent requests will use the cached grammar and will be much faster.
+
+

+

+
-### Constrain with Pydantic
+## How to use Guidance?
-Pydantic is a powerful library for data validation and settings management. It's the perfect tool for crafting the a specific response format.
+There are two main ways to use guidance; you can either use the `/generate` endpoint with a grammar or use the `/chat/completion` endpoint with tools.
-Using Pydantic models we can define a similar grammar as the previous example in a shorter and more readable way.
+Under the hood tools are a special case of grammars that allows the model to choose one or none of the provided tools.
-```python
-import requests
-from pydantic import BaseModel, conint
-from typing import List
+Please refer to [using guidance](../basic_tutorial/using_guidance) for more examples and details on how to use guidance in Python, JavaScript, and cURL.
-class Animals(BaseModel):
- location: str
- activity: str
- animals_seen: conint(ge=1, le=5) # Constrained integer type
- animals: List[str]
+### Getting the most out of guidance
-prompt = "convert to JSON: I saw a puppy a cat and a raccoon during my bike ride in the park"
+Depending on how you are using guidance, you may want to make use of different features. Here are some tips to get the most out of guidance:
-data = {
- "inputs": prompt,
- "parameters": {
- "repetition_penalty": 1.3,
- "grammar": {
- "type": "json",
- "value": Animals.schema()
- }
- }
-}
-
-headers = {
- "Content-Type": "application/json",
-}
-
-response = requests.post(
- 'http://127.0.0.1:3000/generate',
- headers=headers,
- json=data
-)
-print(response.json())
-# {'generated_text': '{ "activity": "bike riding", "animals": ["puppy","cat","raccoon"],"animals_seen": 3, "location":"park" }'}
-
-```
-
-### JSON Schema Integration
-
-If Pydantic's not your style, go raw with direct JSON Schema integration. It's like having a conversation with the AI in its own language. This is simliar to the first example but with programmatic control.
-
-```python
-import requests
-
-json_schema = {
- "properties": {
- "location": {
- "type": "string"
- },
- "activity": {
- "type": "string"
- },
- "animals_seen": {
- "type": "integer",
- "minimum": 1,
- "maximum": 5
- },
- "animals": {
- "type": "array",
- "items": {
- "type": "string"
- }
- }
- },
- "required": ["location", "activity", "animals_seen", "animals"]
-}
-
-data = {
- "inputs": "[INST]convert to JSON: I saw a puppy a cat and a raccoon during my bike ride in the park [/INST]",
- "parameters": {
- "max_new_tokens": 200,
- "repetition_penalty": 1.3,
- "grammar": {
- "type": "json",
- "value": json_schema
- }
- }
-}
-
-headers = {
- "Content-Type": "application/json",
-}
-
-response = requests.post(
- 'http://127.0.0.1:3000/generate',
- headers=headers,
- json=data
-)
-print(response.json())
-# {'generated_text': '{\n"activity": "biking",\n"animals": ["puppy","cat","raccoon"]\n , "animals_seen": 3,\n "location":"park"}'}
-
-```
-
-### Using the client
-
-TGI provides a client library to that make it easy to send requests with all of the parameters we've discussed above. Here's an example of how to use the client to send a request with a grammar parameter.
-
-```python
-from text_generation import AsyncClient
-from text_generation.types import GrammarType
-
-# NOTE: tools defined above and removed for brevity
-
-# Define an async function to encapsulate the async operation
-async def main():
- client = AsyncClient(base_url="http://localhost:3000")
-
- # Use 'await' to wait for the async method 'chat' to complete
- response = await client.generate(
- "Whats Googles DNS",
- max_new_tokens=10,
- decoder_input_details=True,
- seed=1,
- grammar={
- "type": GrammarType.Regex,
- "value": "((25[0-5]|2[0-4]\\d|[01]?\\d\\d?)\\.){3}(25[0-5]|2[0-4]\\d|[01]?\\d\\d?)",
- },
- )
-
- # Once the response is received, you can process it
- print(response.generated_text)
-
-# Ensure the main async function is run in the event loop
-if __name__ == "__main__":
- import asyncio
- asyncio.run(main())
-
-# 118.8.0.84
-
-```
-
-## Tools and Functions 🛠️
-
-### The Tools Parameter
-
-In addition to the grammar parameter, we've also introduced a set of tools and functions to help you get the most out of the Messages API.
-
-Tools are a set of user defined functions that can be used in tandem with the chat functionality to enhance the AI's capabilities. You can use these tools to perform a variety of tasks, such as data manipulation, formatting, and more.
-
-Functions, similar to grammar are defined as JSON schema and can be passed as part of the parameters to the Messages API.
-
-```json
-curl localhost:3000/v1/chat/completions \
- -X POST \
- -H 'Content-Type: application/json' \
- -d '{
- "model": "tgi",
- "messages": [
- {
- "role": "user",
- "content": "What is the weather like in New York?"
- }
- ],
- "tools": [
- {
- "type": "function",
- "function": {
- "name": "get_current_weather",
- "description": "Get the current weather",
- "parameters": {
- "type": "object",
- "properties": {
- "location": {
- "type": "string",
- "description": "The city and state, e.g. San Francisco, CA"
- },
- "format": {
- "type": "string",
- "enum": ["celsius", "fahrenheit"],
- "description": "The temperature unit to use. Infer this from the users location."
- }
- },
- "required": ["location", "format"]
- }
- }
- }
- ],
- "tool_choice": "get_current_weather"
-}'
-// {"id":"","object":"text_completion","created":1709051640,"model":"HuggingFaceH4/zephyr-7b-beta","system_fingerprint":"1.4.3-native","choices":[{"index":0,"message":{"role":"assistant","tool_calls":{"id":0,"type":"function","function":{"description":null,"name":"tools","parameters":{"format":"celsius","location":"New York"}}}},"logprobs":null,"finish_reason":"eos_token"}],"usage":{"prompt_tokens":157,"completion_tokens":19,"total_tokens":176}}
-```
-
-
- Tools used in example below
-
- ```python
- tools = [
- {
- "type": "function",
- "function": {
- "name": "get_current_weather",
- "description": "Get the current weather",
- "parameters": {
- "type": "object",
- "properties": {
- "location": {
- "type": "string",
- "description": "The city and state, e.g. San Francisco, CA",
- },
- "format": {
- "type": "string",
- "enum": ["celsius", "fahrenheit"],
- "description": "The temperature unit to use. Infer this from the users location.",
- },
- },
- "required": ["location", "format"],
- },
- },
- },
- {
- "type": "function",
- "function": {
- "name": "get_n_day_weather_forecast",
- "description": "Get an N-day weather forecast",
- "parameters": {
- "type": "object",
- "properties": {
- "location": {
- "type": "string",
- "description": "The city and state, e.g. San Francisco, CA",
- },
- "format": {
- "type": "string",
- "enum": ["celsius", "fahrenheit"],
- "description": "The temperature unit to use. Infer this from the users location.",
- },
- "num_days": {
- "type": "integer",
- "description": "The number of days to forecast",
- },
- },
- "required": ["location", "format", "num_days"],
- },
- },
- }
- ]
- ```
-
-
-
-### Text Generation Inference Client
-
-TGI provides a client library to interact with the Messages API and Tool functions. The client library is available in both synchronous and asynchronous versions.
-
-```python
-from text_generation import AsyncClient
-
-# NOTE: tools defined above and removed for brevity
-
-# Define an async function to encapsulate the async operation
-async def main():
- client = AsyncClient(base_url="http://localhost:3000")
-
- # Use 'await' to wait for the async method 'chat' to complete
- response = await client.chat(
- max_tokens=100,
- seed=1,
- tools=tools,
- presence_penalty=-1.1,
- messages=[
- {
- "role": "system",
- "content": "You're a helpful assistant! Answer the users question best you can.",
- },
- {
- "role": "user",
- "content": "What is the weather like in Brooklyn, New York?",
- },
- ],
- )
-
- # Once the response is received, you can process it
- print(response.choices[0].message.tool_calls)
-
-# Ensure the main async function is run in the event loop
-if __name__ == "__main__":
- import asyncio
- asyncio.run(main())
-
-# {"id":"","object":"text_completion","created":1709051942,"model":"HuggingFaceH4/zephyr-7b-beta","system_fingerprint":"1.4.3-native","choices":[{"index":0,"message":{"role":"assistant","tool_calls":{"id":0,"type":"function","function":{"description":null,"name":"tools","parameters":{"format":"celsius","location":"New York"}}}},"logprobs":null,"finish_reason":"eos_token"}],"usage":{"prompt_tokens":157,"completion_tokens":20,"total_tokens":177}}
-
-```
-
-### OpenAI integration
-
-TGI exposes an OpenAI-compatible API, which means you can use OpenAI's client libraries to interact with TGI's Messages API and Tool functions.
-
-However there are some minor differences in the API, for example `tool_choice="auto"` will ALWAYS choose the tool for you. This is different from OpenAI's API where `tool_choice="auto"` will choose a tool if the model thinks it's necessary.
-
-```python
-from openai import OpenAI
-
-# Initialize the client, pointing it to one of the available models
-client = OpenAI(
- base_url="http://localhost:3000/v1",
- api_key="_",
-)
-
-# NOTE: tools defined above and removed for brevity
-
-chat_completion = client.chat.completions.create(
- model="tgi",
- messages=[
- {
- "role": "system",
- "content": "Don't make assumptions about what values to plug into functions. Ask for clarification if a user request is ambiguous.",
- },
- {
- "role": "user",
- "content": "What's the weather like the next 3 days in San Francisco, CA?",
- },
- ],
- tools=tools,
- tool_choice="auto", # tool selected by model
- max_tokens=500,
-)
-
-
-called = chat_completion.choices[0].message.tool_calls
-print(called)
-# {
-# "id": 0,
-# "type": "function",
-# "function": {
-# "description": None,
-# "name": "tools",
-# "parameters": {
-# "format": "celsius",
-# "location": "San Francisco, CA",
-# "num_days": 3,
-# },
-# },
-# }
-```
+- If you are using the `/generate` with a `grammar` it is recommended to include the grammar in the prompt prefixed by something like `Please use the following JSON schema to generate the output:`. This will help the model understand the context of the grammar and generate the output accordingly.
+- If you are getting a response with many repeated tokens, please use the `frequency_penalty` or `repetition_penalty` to reduce the number of repeated tokens in the output.
diff --git a/docs/source/conceptual/speculation.md b/docs/source/conceptual/speculation.md
index 071b7b68..79b1c82e 100644
--- a/docs/source/conceptual/speculation.md
+++ b/docs/source/conceptual/speculation.md
@@ -1,5 +1,6 @@
## Speculation
+
Speculative decoding, assisted generation, Medusa, and others are a few different names for the same idea.
The idea is to generate tokens *before* the large model actually runs, and only *check* if those tokens where valid.
@@ -36,7 +37,7 @@ In order to use medusa models in TGI, simply point to a medusa enabled model, an
If you don't have a medusa model, or don't have the resource to fine-tune, you can try to use `n-gram`.
-Ngram works by trying to find in the previous sequence existing tokens that match, and use those as speculation.
+N-gram works by trying to find matching tokens in the previous sequence, and use those as speculation for generating new tokens. For example, if the tokens "np.mean" appear multiple times in the sequence, the model can speculate that the next continuation of the tokens "np." is probably also "mean".
This is an extremely simple method, which works best for code, or highly repetitive text. This might not be beneficial, if the speculation misses too much.
diff --git a/docs/source/conceptual/streaming.md b/docs/source/conceptual/streaming.md
index 505a0d9e..71ec9b25 100644
--- a/docs/source/conceptual/streaming.md
+++ b/docs/source/conceptual/streaming.md
@@ -15,7 +15,7 @@ Token streaming is the mode in which the server returns the tokens one by one as
/>
-With token streaming, the server can start returning the tokens one by one before having to generate the whole response. Users can have a sense of the generation's quality earlier than the end of the generation. This has different positive effects:
+With token streaming, the server can start returning the tokens one by one before having to generate the whole response. Users can have a sense of the generation's quality before the end of the generation. This has different positive effects:
* Users can get results orders of magnitude earlier for extremely long queries.
* Seeing something in progress allows users to stop the generation if it's not going in the direction they expect.
@@ -116,7 +116,7 @@ curl -N 127.0.0.1:8080/generate_stream \
First, we need to install the `@huggingface/inference` library.
`npm install @huggingface/inference`
-If you're using the free Inference API, you can use `HfInference`. If you're using inference endpoints, you can use `HfInferenceEndpoint`. Let's
+If you're using the free Inference API, you can use `HfInference`. If you're using inference endpoints, you can use `HfInferenceEndpoint`.
We can create a `HfInferenceEndpoint` providing our endpoint URL and credential.
diff --git a/docs/source/index.md b/docs/source/index.md
index 8bf45dce..309442b1 100644
--- a/docs/source/index.md
+++ b/docs/source/index.md
@@ -18,8 +18,8 @@ Text Generation Inference implements many optimizations and features, such as:
- Logits warper (temperature scaling, top-p, top-k, repetition penalty)
- Stop sequences
- Log probabilities
-- Custom Prompt Generation: Easily generate text by providing custom prompts to guide the model's output.
- Fine-tuning Support: Utilize fine-tuned models for specific tasks to achieve higher accuracy and performance.
+- [Guidance](../conceptual/guidance): Enable function calling and tool-use by forcing the model to generate structured outputs based on your own predefined output schemas.
Text Generation Inference is used in production by multiple projects, such as:
diff --git a/integration-tests/conftest.py b/integration-tests/conftest.py
index cf0f498d..ae3f977b 100644
--- a/integration-tests/conftest.py
+++ b/integration-tests/conftest.py
@@ -293,6 +293,7 @@ def launcher(event_loop):
dtype: Optional[str] = None,
revision: Optional[str] = None,
max_input_length: Optional[int] = None,
+ max_batch_prefill_tokens: Optional[int] = None,
max_total_tokens: Optional[int] = None,
):
port = random.randint(8000, 10_000)
@@ -334,6 +335,9 @@ def launcher(event_loop):
if max_input_length:
args.append("--max-input-length")
args.append(str(max_input_length))
+ if max_batch_prefill_tokens:
+ args.append("--max-batch-prefill-tokens")
+ args.append(str(max_batch_prefill_tokens))
if max_total_tokens:
args.append("--max-total-tokens")
args.append(str(max_total_tokens))
@@ -371,6 +375,7 @@ def launcher(event_loop):
dtype: Optional[str] = None,
revision: Optional[str] = None,
max_input_length: Optional[int] = None,
+ max_batch_prefill_tokens: Optional[int] = None,
max_total_tokens: Optional[int] = None,
):
port = random.randint(8000, 10_000)
@@ -395,6 +400,9 @@ def launcher(event_loop):
if max_input_length:
args.append("--max-input-length")
args.append(str(max_input_length))
+ if max_batch_prefill_tokens:
+ args.append("--max-batch-prefill-tokens")
+ args.append(str(max_batch_prefill_tokens))
if max_total_tokens:
args.append("--max-total-tokens")
args.append(str(max_total_tokens))
diff --git a/integration-tests/models/__snapshots__/test_idefics2/test_flash_idefics2_next_all_params.json b/integration-tests/models/__snapshots__/test_idefics2/test_flash_idefics2_next_all_params.json
new file mode 100644
index 00000000..45601505
--- /dev/null
+++ b/integration-tests/models/__snapshots__/test_idefics2/test_flash_idefics2_next_all_params.json
@@ -0,0 +1,89 @@
+{
+ "details": {
+ "best_of_sequences": null,
+ "finish_reason": "length",
+ "generated_tokens": 10,
+ "prefill": [
+ {
+ "id": 1,
+ "logprob": null,
+ "text": ""
+ },
+ {
+ "id": 3735,
+ "logprob": -8.5625,
+ "text": "Test"
+ },
+ {
+ "id": 2159,
+ "logprob": -10.78125,
+ "text": "request"
+ }
+ ],
+ "seed": 0,
+ "tokens": [
+ {
+ "id": 288,
+ "logprob": -0.2854004,
+ "special": false,
+ "text": "ing"
+ },
+ {
+ "id": 264,
+ "logprob": -0.37573242,
+ "special": false,
+ "text": " a"
+ },
+ {
+ "id": 633,
+ "logprob": -0.09301758,
+ "special": false,
+ "text": " new"
+ },
+ {
+ "id": 4480,
+ "logprob": -0.3322754,
+ "special": false,
+ "text": " feature"
+ },
+ {
+ "id": 297,
+ "logprob": -0.8510742,
+ "special": false,
+ "text": " in"
+ },
+ {
+ "id": 272,
+ "logprob": -0.13464355,
+ "special": false,
+ "text": " the"
+ },
+ {
+ "id": 2039,
+ "logprob": 0.0,
+ "special": false,
+ "text": " game"
+ },
+ {
+ "id": 28723,
+ "logprob": -0.89990234,
+ "special": false,
+ "text": "."
+ },
+ {
+ "id": 13,
+ "logprob": 0.0,
+ "special": false,
+ "text": "\n"
+ },
+ {
+ "id": 13,
+ "logprob": 0.0,
+ "special": false,
+ "text": "\n"
+ }
+ ],
+ "top_tokens": null
+ },
+ "generated_text": "Test requesting a new feature in the game.\n\n"
+}
diff --git a/integration-tests/models/__snapshots__/test_idefics2/test_flash_idefics2_next_load.json b/integration-tests/models/__snapshots__/test_idefics2/test_flash_idefics2_next_load.json
new file mode 100644
index 00000000..4bc90896
--- /dev/null
+++ b/integration-tests/models/__snapshots__/test_idefics2/test_flash_idefics2_next_load.json
@@ -0,0 +1,7018 @@
+[
+ {
+ "details": {
+ "best_of_sequences": null,
+ "finish_reason": "length",
+ "generated_tokens": 10,
+ "prefill": [
+ {
+ "id": 1,
+ "logprob": null,
+ "text": ""
+ },
+ {
+ "id": 1247,
+ "logprob": -5.2421875,
+ "text": "User"
+ },
+ {
+ "id": 28747,
+ "logprob": -6.9570312,
+ "text": ":"
+ },
+ {
+ "id": 32000,
+ "logprob": -16.234375,
+ "text": ""
+ },
+ {
+ "id": 32001,
+ "logprob": -18.96875,
+ "text": ""
+ },
+ {
+ "id": 32001,
+ "logprob": -18.1875,
+ "text": ""
+ },
+ {
+ "id": 32001,
+ "logprob": -19.484375,
+ "text": ""
+ },
+ {
+ "id": 32001,
+ "logprob": -17.578125,
+ "text": ""
+ },
+ {
+ "id": 32001,
+ "logprob": -16.8125,
+ "text": ""
+ },
+ {
+ "id": 32001,
+ "logprob": -19.296875,
+ "text": ""
+ },
+ {
+ "id": 32001,
+ "logprob": -20.234375,
+ "text": ""
+ },
+ {
+ "id": 32001,
+ "logprob": -16.421875,
+ "text": ""
+ },
+ {
+ "id": 32001,
+ "logprob": -19.828125,
+ "text": ""
+ },
+ {
+ "id": 32001,
+ "logprob": -23.25,
+ "text": ""
+ },
+ {
+ "id": 32001,
+ "logprob": -19.421875,
+ "text": ""
+ },
+ {
+ "id": 32001,
+ "logprob": -15.28125,
+ "text": ""
+ },
+ {
+ "id": 32001,
+ "logprob": -17.734375,
+ "text": ""
+ },
+ {
+ "id": 32001,
+ "logprob": -20.34375,
+ "text": ""
+ },
+ {
+ "id": 32001,
+ "logprob": -21.296875,
+ "text": ""
+ },
+ {
+ "id": 32001,
+ "logprob": -21.015625,
+ "text": ""
+ },
+ {
+ "id": 32001,
+ "logprob": -20.421875,
+ "text": ""
+ },
+ {
+ "id": 32001,
+ "logprob": -16.015625,
+ "text": ""
+ },
+ {
+ "id": 32001,
+ "logprob": -19.0625,
+ "text": ""
+ },
+ {
+ "id": 32001,
+ "logprob": -22.765625,
+ "text": ""
+ },
+ {
+ "id": 32001,
+ "logprob": -23.625,
+ "text": ""
+ },
+ {
+ "id": 32001,
+ "logprob": -20.40625,
+ "text": ""
+ },
+ {
+ "id": 32001,
+ "logprob": -20.421875,
+ "text": ""
+ },
+ {
+ "id": 32001,
+ "logprob": -20.84375,
+ "text": ""
+ },
+ {
+ "id": 32001,
+ "logprob": -16.5,
+ "text": ""
+ },
+ {
+ "id": 32001,
+ "logprob": -19.984375,
+ "text": ""
+ },
+ {
+ "id": 32001,
+ "logprob": -18.21875,
+ "text": ""
+ },
+ {
+ "id": 32001,
+ "logprob": -23.59375,
+ "text": ""
+ },
+ {
+ "id": 32001,
+ "logprob": -21.203125,
+ "text": ""
+ },
+ {
+ "id": 32001,
+ "logprob": -17.359375,
+ "text": ""
+ },
+ {
+ "id": 32001,
+ "logprob": -16.53125,
+ "text": ""
+ },
+ {
+ "id": 32001,
+ "logprob": -18.984375,
+ "text": ""
+ },
+ {
+ "id": 32001,
+ "logprob": -21.78125,
+ "text": ""
+ },
+ {
+ "id": 32001,
+ "logprob": -19.328125,
+ "text": ""
+ },
+ {
+ "id": 32001,
+ "logprob": -18.0,
+ "text": ""
+ },
+ {
+ "id": 32001,
+ "logprob": -18.828125,
+ "text": ""
+ },
+ {
+ "id": 32001,
+ "logprob": -17.9375,
+ "text": ""
+ },
+ {
+ "id": 32001,
+ "logprob": -19.1875,
+ "text": ""
+ },
+ {
+ "id": 32001,
+ "logprob": -18.640625,
+ "text": ""
+ },
+ {
+ "id": 32001,
+ "logprob": -20.125,
+ "text": ""
+ },
+ {
+ "id": 32001,
+ "logprob": -19.4375,
+ "text": ""
+ },
+ {
+ "id": 32001,
+ "logprob": -14.8828125,
+ "text": ""
+ },
+ {
+ "id": 32001,
+ "logprob": -18.453125,
+ "text": ""
+ },
+ {
+ "id": 32001,
+ "logprob": -23.21875,
+ "text": ""
+ },
+ {
+ "id": 32001,
+ "logprob": -17.4375,
+ "text": ""
+ },
+ {
+ "id": 32001,
+ "logprob": -23.671875,
+ "text": ""
+ },
+ {
+ "id": 32001,
+ "logprob": -23.015625,
+ "text": ""
+ },
+ {
+ "id": 32001,
+ "logprob": -19.75,
+ "text": ""
+ },
+ {
+ "id": 32001,
+ "logprob": -17.078125,
+ "text": ""
+ },
+ {
+ "id": 32001,
+ "logprob": -17.640625,
+ "text": ""
+ },
+ {
+ "id": 32001,
+ "logprob": -17.046875,
+ "text": ""
+ },
+ {
+ "id": 32001,
+ "logprob": -18.40625,
+ "text": ""
+ },
+ {
+ "id": 32001,
+ "logprob": -20.578125,
+ "text": ""
+ },
+ {
+ "id": 32001,
+ "logprob": -18.34375,
+ "text": ""
+ },
+ {
+ "id": 32001,
+ "logprob": -17.140625,
+ "text": ""
+ },
+ {
+ "id": 32001,
+ "logprob": -18.671875,
+ "text": ""
+ },
+ {
+ "id": 32001,
+ "logprob": -18.875,
+ "text": ""
+ },
+ {
+ "id": 32001,
+ "logprob": -16.0,
+ "text": ""
+ },
+ {
+ "id": 32001,
+ "logprob": -18.1875,
+ "text": ""
+ },
+ {
+ "id": 32001,
+ "logprob": -18.84375,
+ "text": ""
+ },
+ {
+ "id": 32001,
+ "logprob": -20.15625,
+ "text": ""
+ },
+ {
+ "id": 32001,
+ "logprob": -18.96875,
+ "text": ""
+ },
+ {
+ "id": 32001,
+ "logprob": -18.71875,
+ "text": ""
+ },
+ {
+ "id": 32001,
+ "logprob": -17.9375,
+ "text": ""
+ },
+ {
+ "id": 32001,
+ "logprob": -17.921875,
+ "text": ""
+ },
+ {
+ "id": 32001,
+ "logprob": -19.125,
+ "text": ""
+ },
+ {
+ "id": 32001,
+ "logprob": -20.296875,
+ "text": ""
+ },
+ {
+ "id": 32001,
+ "logprob": -17.25,
+ "text": ""
+ },
+ {
+ "id": 32001,
+ "logprob": -17.96875,
+ "text": ""
+ },
+ {
+ "id": 32001,
+ "logprob": -19.546875,
+ "text": ""
+ },
+ {
+ "id": 32001,
+ "logprob": -19.921875,
+ "text": ""
+ },
+ {
+ "id": 32001,
+ "logprob": -16.3125,
+ "text": ""
+ },
+ {
+ "id": 32001,
+ "logprob": -19.546875,
+ "text": ""
+ },
+ {
+ "id": 32001,
+ "logprob": -20.1875,
+ "text": ""
+ },
+ {
+ "id": 32001,
+ "logprob": -20.953125,
+ "text": ""
+ },
+ {
+ "id": 32001,
+ "logprob": -15.828125,
+ "text": ""
+ },
+ {
+ "id": 32001,
+ "logprob": -16.375,
+ "text": ""
+ },
+ {
+ "id": 32001,
+ "logprob": -20.171875,
+ "text": ""
+ },
+ {
+ "id": 32001,
+ "logprob": -19.03125,
+ "text": ""
+ },
+ {
+ "id": 32001,
+ "logprob": -21.71875,
+ "text": ""
+ },
+ {
+ "id": 32001,
+ "logprob": -20.65625,
+ "text": ""
+ },
+ {
+ "id": 32001,
+ "logprob": -19.453125,
+ "text": ""
+ },
+ {
+ "id": 32001,
+ "logprob": -19.484375,
+ "text": ""
+ },
+ {
+ "id": 32001,
+ "logprob": -16.875,
+ "text": ""
+ },
+ {
+ "id": 32001,
+ "logprob": -20.65625,
+ "text": ""
+ },
+ {
+ "id": 32001,
+ "logprob": -21.296875,
+ "text": ""
+ },
+ {
+ "id": 32001,
+ "logprob": -20.546875,
+ "text": ""
+ },
+ {
+ "id": 32001,
+ "logprob": -20.78125,
+ "text": ""
+ },
+ {
+ "id": 32001,
+ "logprob": -17.96875,
+ "text": ""
+ },
+ {
+ "id": 32001,
+ "logprob": -20.5,
+ "text": ""
+ },
+ {
+ "id": 32001,
+ "logprob": -15.4140625,
+ "text": ""
+ },
+ {
+ "id": 32001,
+ "logprob": -19.046875,
+ "text": ""
+ },
+ {
+ "id": 32001,
+ "logprob": -18.109375,
+ "text": ""
+ },
+ {
+ "id": 32001,
+ "logprob": -15.7265625,
+ "text": ""
+ },
+ {
+ "id": 32001,
+ "logprob": -18.5625,
+ "text": ""
+ },
+ {
+ "id": 32001,
+ "logprob": -20.734375,
+ "text": ""
+ },
+ {
+ "id": 32001,
+ "logprob": -20.359375,
+ "text": ""
+ },
+ {
+ "id": 32001,
+ "logprob": -18.421875,
+ "text": ""
+ },
+ {
+ "id": 32001,
+ "logprob": -17.984375,
+ "text": ""
+ },
+ {
+ "id": 32001,
+ "logprob": -17.265625,
+ "text": ""
+ },
+ {
+ "id": 32001,
+ "logprob": -16.78125,
+ "text": ""
+ },
+ {
+ "id": 32001,
+ "logprob": -21.046875,
+ "text": ""
+ },
+ {
+ "id": 32001,
+ "logprob": -18.4375,
+ "text": ""
+ },
+ {
+ "id": 32001,
+ "logprob": -19.78125,
+ "text": ""
+ },
+ {
+ "id": 32001,
+ "logprob": -19.671875,
+ "text": ""
+ },
+ {
+ "id": 32001,
+ "logprob": -14.2421875,
+ "text": ""
+ },
+ {
+ "id": 32001,
+ "logprob": -19.0,
+ "text": ""
+ },
+ {
+ "id": 32001,
+ "logprob": -18.40625,
+ "text": ""
+ },
+ {
+ "id": 32001,
+ "logprob": -17.59375,
+ "text": ""
+ },
+ {
+ "id": 32001,
+ "logprob": -20.671875,
+ "text": ""
+ },
+ {
+ "id": 32001,
+ "logprob": -20.265625,
+ "text": ""
+ },
+ {
+ "id": 32001,
+ "logprob": -20.578125,
+ "text": ""
+ },
+ {
+ "id": 32001,
+ "logprob": -18.234375,
+ "text": ""
+ },
+ {
+ "id": 32001,
+ "logprob": -17.484375,
+ "text": ""
+ },
+ {
+ "id": 32001,
+ "logprob": -16.84375,
+ "text": ""
+ },
+ {
+ "id": 32001,
+ "logprob": -18.296875,
+ "text": ""
+ },
+ {
+ "id": 32001,
+ "logprob": -15.8671875,
+ "text": ""
+ },
+ {
+ "id": 32001,
+ "logprob": -18.375,
+ "text": ""
+ },
+ {
+ "id": 32001,
+ "logprob": -18.765625,
+ "text": ""
+ },
+ {
+ "id": 32001,
+ "logprob": -18.609375,
+ "text": ""
+ },
+ {
+ "id": 32001,
+ "logprob": -17.515625,
+ "text": ""
+ },
+ {
+ "id": 32001,
+ "logprob": -16.25,
+ "text": ""
+ },
+ {
+ "id": 32001,
+ "logprob": -19.640625,
+ "text": ""
+ },
+ {
+ "id": 32001,
+ "logprob": -14.8515625,
+ "text": ""
+ },
+ {
+ "id": 32001,
+ "logprob": -20.875,
+ "text": ""
+ },
+ {
+ "id": 32001,
+ "logprob": -20.28125,
+ "text": ""
+ },
+ {
+ "id": 32001,
+ "logprob": -18.453125,
+ "text": ""
+ },
+ {
+ "id": 32001,
+ "logprob": -18.25,
+ "text": ""
+ },
+ {
+ "id": 32001,
+ "logprob": -19.203125,
+ "text": ""
+ },
+ {
+ "id": 32001,
+ "logprob": -18.71875,
+ "text": ""
+ },
+ {
+ "id": 32001,
+ "logprob": -19.390625,
+ "text": ""
+ },
+ {
+ "id": 32001,
+ "logprob": -17.984375,
+ "text": ""
+ },
+ {
+ "id": 32001,
+ "logprob": -17.390625,
+ "text": ""
+ },
+ {
+ "id": 32001,
+ "logprob": -20.5,
+ "text": ""
+ },
+ {
+ "id": 32001,
+ "logprob": -18.296875,
+ "text": ""
+ },
+ {
+ "id": 32001,
+ "logprob": -16.4375,
+ "text": ""
+ },
+ {
+ "id": 32001,
+ "logprob": -20.015625,
+ "text": ""
+ },
+ {
+ "id": 32001,
+ "logprob": -18.359375,
+ "text": ""
+ },
+ {
+ "id": 32001,
+ "logprob": -17.375,
+ "text": ""
+ },
+ {
+ "id": 32001,
+ "logprob": -15.8125,
+ "text": ""
+ },
+ {
+ "id": 32001,
+ "logprob": -16.953125,
+ "text": ""
+ },
+ {
+ "id": 32001,
+ "logprob": -17.515625,
+ "text": ""
+ },
+ {
+ "id": 32001,
+ "logprob": -16.875,
+ "text": ""
+ },
+ {
+ "id": 32001,
+ "logprob": -19.109375,
+ "text": ""
+ },
+ {
+ "id": 32001,
+ "logprob": -19.265625,
+ "text": ""
+ },
+ {
+ "id": 32001,
+ "logprob": -17.234375,
+ "text": ""
+ },
+ {
+ "id": 32001,
+ "logprob": -19.28125,
+ "text": ""
+ },
+ {
+ "id": 32001,
+ "logprob": -20.25,
+ "text": ""
+ },
+ {
+ "id": 32001,
+ "logprob": -20.25,
+ "text": ""
+ },
+ {
+ "id": 32001,
+ "logprob": -20.765625,
+ "text": ""
+ },
+ {
+ "id": 32001,
+ "logprob": -19.609375,
+ "text": ""
+ },
+ {
+ "id": 32001,
+ "logprob": -20.359375,
+ "text": ""
+ },
+ {
+ "id": 32001,
+ "logprob": -19.90625,
+ "text": ""
+ },
+ {
+ "id": 32001,
+ "logprob": -20.28125,
+ "text": ""
+ },
+ {
+ "id": 32001,
+ "logprob": -19.5,
+ "text": ""
+ },
+ {
+ "id": 32001,
+ "logprob": -20.0625,
+ "text": ""
+ },
+ {
+ "id": 32001,
+ "logprob": -18.125,
+ "text": ""
+ },
+ {
+ "id": 32001,
+ "logprob": -17.40625,
+ "text": ""
+ },
+ {
+ "id": 32001,
+ "logprob": -16.546875,
+ "text": ""
+ },
+ {
+ "id": 32001,
+ "logprob": -19.84375,
+ "text": ""
+ },
+ {
+ "id": 32001,
+ "logprob": -20.484375,
+ "text": ""
+ },
+ {
+ "id": 32001,
+ "logprob": -19.265625,
+ "text": ""
+ },
+ {
+ "id": 32001,
+ "logprob": -16.0625,
+ "text": ""
+ },
+ {
+ "id": 32001,
+ "logprob": -20.234375,
+ "text": ""
+ },
+ {
+ "id": 32001,
+ "logprob": -15.9453125,
+ "text": ""
+ },
+ {
+ "id": 32001,
+ "logprob": -21.0625,
+ "text": ""
+ },
+ {
+ "id": 32001,
+ "logprob": -20.515625,
+ "text": ""
+ },
+ {
+ "id": 32001,
+ "logprob": -19.375,
+ "text": ""
+ },
+ {
+ "id": 32001,
+ "logprob": -17.796875,
+ "text": ""
+ },
+ {
+ "id": 32001,
+ "logprob": -16.03125,
+ "text": ""
+ },
+ {
+ "id": 32001,
+ "logprob": -18.671875,
+ "text": ""
+ },
+ {
+ "id": 32001,
+ "logprob": -20.15625,
+ "text": ""
+ },
+ {
+ "id": 32001,
+ "logprob": -20.234375,
+ "text": ""
+ },
+ {
+ "id": 32001,
+ "logprob": -20.84375,
+ "text": ""
+ },
+ {
+ "id": 32001,
+ "logprob": -19.78125,
+ "text": ""
+ },
+ {
+ "id": 32001,
+ "logprob": -19.234375,
+ "text": ""
+ },
+ {
+ "id": 32001,
+ "logprob": -18.078125,
+ "text": ""
+ },
+ {
+ "id": 32001,
+ "logprob": -19.28125,
+ "text": ""
+ },
+ {
+ "id": 32001,
+ "logprob": -17.09375,
+ "text": ""
+ },
+ {
+ "id": 32001,
+ "logprob": -16.59375,
+ "text": ""
+ },
+ {
+ "id": 32001,
+ "logprob": -16.65625,
+ "text": ""
+ },
+ {
+ "id": 32001,
+ "logprob": -19.453125,
+ "text": ""
+ },
+ {
+ "id": 32001,
+ "logprob": -19.9375,
+ "text": ""
+ },
+ {
+ "id": 32001,
+ "logprob": -16.703125,
+ "text": ""
+ },
+ {
+ "id": 32001,
+ "logprob": -18.15625,
+ "text": ""
+ },
+ {
+ "id": 32001,
+ "logprob": -16.46875,
+ "text": ""
+ },
+ {
+ "id": 32001,
+ "logprob": -19.796875,
+ "text": ""
+ },
+ {
+ "id": 32001,
+ "logprob": -17.34375,
+ "text": ""
+ },
+ {
+ "id": 32001,
+ "logprob": -17.3125,
+ "text": ""
+ },
+ {
+ "id": 32001,
+ "logprob": -20.203125,
+ "text": ""
+ },
+ {
+ "id": 32001,
+ "logprob": -18.921875,
+ "text": ""
+ },
+ {
+ "id": 32001,
+ "logprob": -18.09375,
+ "text": ""
+ },
+ {
+ "id": 32001,
+ "logprob": -18.6875,
+ "text": ""
+ },
+ {
+ "id": 32001,
+ "logprob": -22.625,
+ "text": ""
+ },
+ {
+ "id": 32001,
+ "logprob": -21.375,
+ "text": ""
+ },
+ {
+ "id": 32001,
+ "logprob": -18.765625,
+ "text": ""
+ },
+ {
+ "id": 32001,
+ "logprob": -18.46875,
+ "text": ""
+ },
+ {
+ "id": 32001,
+ "logprob": -20.546875,
+ "text": ""
+ },
+ {
+ "id": 32001,
+ "logprob": -18.875,
+ "text": ""
+ },
+ {
+ "id": 32001,
+ "logprob": -16.453125,
+ "text": ""
+ },
+ {
+ "id": 32001,
+ "logprob": -21.09375,
+ "text": ""
+ },
+ {
+ "id": 32001,
+ "logprob": -19.5625,
+ "text": ""
+ },
+ {
+ "id": 32001,
+ "logprob": -19.15625,
+ "text": ""
+ },
+ {
+ "id": 32001,
+ "logprob": -16.171875,
+ "text": ""
+ },
+ {
+ "id": 32001,
+ "logprob": -17.671875,
+ "text": ""
+ },
+ {
+ "id": 32001,
+ "logprob": -18.84375,
+ "text": ""
+ },
+ {
+ "id": 32001,
+ "logprob": -20.75,
+ "text": ""
+ },
+ {
+ "id": 32001,
+ "logprob": -21.8125,
+ "text": ""
+ },
+ {
+ "id": 32001,
+ "logprob": -19.96875,
+ "text": ""
+ },
+ {
+ "id": 32001,
+ "logprob": -19.046875,
+ "text": ""
+ },
+ {
+ "id": 32001,
+ "logprob": -19.78125,
+ "text": ""
+ },
+ {
+ "id": 32001,
+ "logprob": -19.421875,
+ "text": ""
+ },
+ {
+ "id": 32001,
+ "logprob": -21.21875,
+ "text": ""
+ },
+ {
+ "id": 32001,
+ "logprob": -21.515625,
+ "text": ""
+ },
+ {
+ "id": 32001,
+ "logprob": -19.609375,
+ "text": ""
+ },
+ {
+ "id": 32001,
+ "logprob": -20.71875,
+ "text": ""
+ },
+ {
+ "id": 32001,
+ "logprob": -19.046875,
+ "text": ""
+ },
+ {
+ "id": 32001,
+ "logprob": -20.1875,
+ "text": ""
+ },
+ {
+ "id": 32001,
+ "logprob": -21.1875,
+ "text": ""
+ },
+ {
+ "id": 32001,
+ "logprob": -19.828125,
+ "text": ""
+ },
+ {
+ "id": 32001,
+ "logprob": -18.359375,
+ "text": ""
+ },
+ {
+ "id": 32001,
+ "logprob": -17.75,
+ "text": ""
+ },
+ {
+ "id": 32001,
+ "logprob": -18.90625,
+ "text": ""
+ },
+ {
+ "id": 32001,
+ "logprob": -18.765625,
+ "text": ""
+ },
+ {
+ "id": 32001,
+ "logprob": -20.453125,
+ "text": ""
+ },
+ {
+ "id": 32001,
+ "logprob": -19.890625,
+ "text": ""
+ },
+ {
+ "id": 32001,
+ "logprob": -16.015625,
+ "text": ""
+ },
+ {
+ "id": 32001,
+ "logprob": -18.90625,
+ "text": ""
+ },
+ {
+ "id": 32001,
+ "logprob": -15.953125,
+ "text": ""
+ },
+ {
+ "id": 32001,
+ "logprob": -21.46875,
+ "text": ""
+ },
+ {
+ "id": 32001,
+ "logprob": -19.984375,
+ "text": ""
+ },
+ {
+ "id": 32001,
+ "logprob": -19.875,
+ "text": ""
+ },
+ {
+ "id": 32001,
+ "logprob": -18.859375,
+ "text": ""
+ },
+ {
+ "id": 32001,
+ "logprob": -16.046875,
+ "text": ""
+ },
+ {
+ "id": 32001,
+ "logprob": -20.375,
+ "text": ""
+ },
+ {
+ "id": 32001,
+ "logprob": -20.140625,
+ "text": ""
+ },
+ {
+ "id": 32001,
+ "logprob": -21.140625,
+ "text": ""
+ },
+ {
+ "id": 32001,
+ "logprob": -21.6875,
+ "text": ""
+ },
+ {
+ "id": 32001,
+ "logprob": -21.453125,
+ "text": ""
+ },
+ {
+ "id": 32001,
+ "logprob": -19.171875,
+ "text": ""
+ },
+ {
+ "id": 32001,
+ "logprob": -17.78125,
+ "text": ""
+ },
+ {
+ "id": 32001,
+ "logprob": -19.65625,
+ "text": ""
+ },
+ {
+ "id": 32001,
+ "logprob": -17.078125,
+ "text": ""
+ },
+ {
+ "id": 32001,
+ "logprob": -17.109375,
+ "text": ""
+ },
+ {
+ "id": 32001,
+ "logprob": -19.171875,
+ "text": ""
+ },
+ {
+ "id": 32001,
+ "logprob": -20.453125,
+ "text": ""
+ },
+ {
+ "id": 32001,
+ "logprob": -21.0625,
+ "text": ""
+ },
+ {
+ "id": 32001,
+ "logprob": -16.734375,
+ "text": ""
+ },
+ {
+ "id": 32001,
+ "logprob": -19.21875,
+ "text": ""
+ },
+ {
+ "id": 32001,
+ "logprob": -16.421875,
+ "text": ""
+ },
+ {
+ "id": 32001,
+ "logprob": -20.015625,
+ "text": "